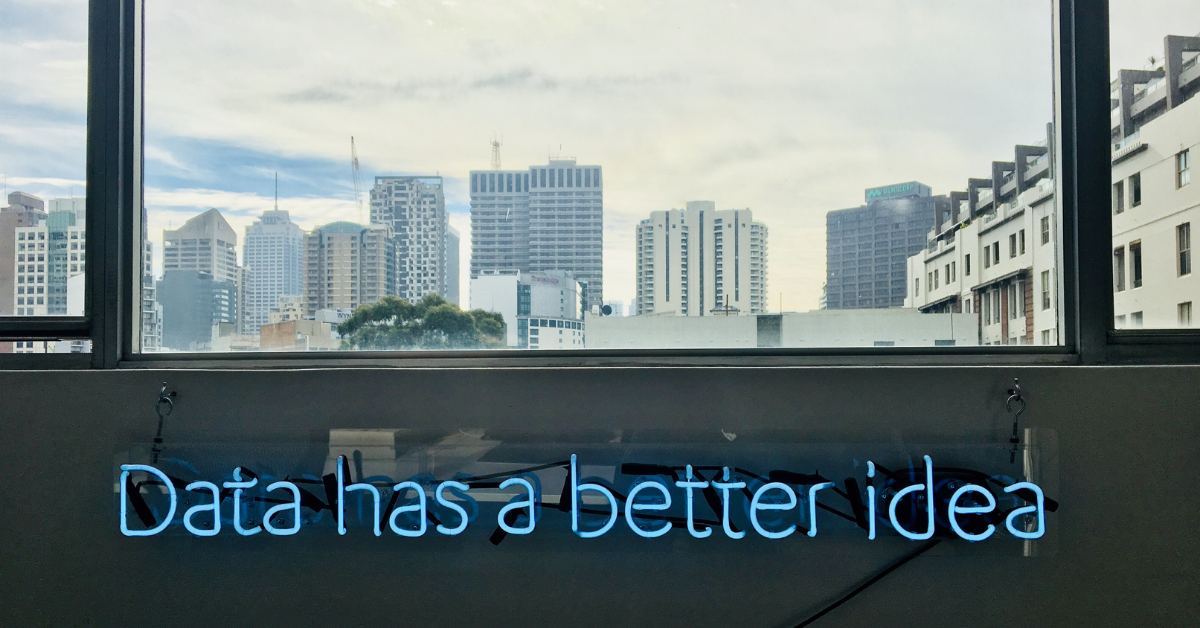
Self-service data analytics is a major milestone for many enterprises, but it often requires an iterative approach to data and analytics architectures to get there. Learn more about how a multi-billion dollar consumer packaged goods leader built a world-class self-service analytics capability after investing in modernizing their highly siloed data architecture.
Through careful investment in technology, strategy, and data processes, this organization was well-positioned to capitalize on the dynamic market for consumer goods caused by the pandemic. Here’s a brief recap of the company’s journey.
The Beginning of a Data & Analytics Journey
In the past, there were a lot of costs associated with data at this large food manufacturing company. For example, data from a business process in disparate systems would be stored temporarily and thrown out after use. This was an inefficient process that discouraged business user adoption of data analytics throughout the organization. When the company started storing data specifically for business intelligence use, however, its business leaders realized that data could offer invaluable insights into the past.
As the food manufacturing company continued its data and analytics journey, the company discovered that bringing data together into a cross-functional format offered even more value. For this, its analytics team focused on moving data into a centralized relational database management system (RDBMS), which later evolved into an enterprise-grade data warehouse solution. This allowed the company to leverage descriptive analytics throughout the entire organization, but the solution couldn’t scale as data began playing a more integral role for the business.
CALLOUT QUOTE “With a data warehouse, we could really harmonize data across different data sets to get more insights out of it,” a data and analytics leader at the company explained. “While this worked great, after a while we realized we needed something more scalable.”
From Descriptive to Predictive Analytics
As the food manufacturing company continued to invest heavily in technology and amass a large amount of data, its data analytics team created a connected data strategy to leverage big data analytics. The goal was to bring all of the organization’s enterprise and functional data under the Hadoop platform so that more time could be spent on analysis rather than moving data around.
By finding a new way to process big data, the food manufacturer was not only able to understand what has happened, but also forecast what is going to happen in the future. This shift from descriptive to predictive analytics enabled the company to consider additional ways to become a more data-driven organization. The problem was that Hadoop alone could no longer scale to meet the company’s needs.
Adopting Self-Service Data Analytics
The move to Hadoop enabled this leading food manufacturer to experiment with self-service data analytics, but in order to keep up with demand, the company wanted to democratize data for its tens of thousands of employees. The only way to achieve this level of data analytics maturity and scale was to adopt a cloud data platform that facilitated stronger governance and consistency.
At the beginning, consistency was a major challenge for the company because a lot of business units were using one-off reports that displayed different results for the same query. That’s why the organizationcreated a new data architecture and data hub, which used a semantic layer to greatly improve the consistency of data analytics and reporting by defining certain performance metrics across different business functions.
A semantic layer is a great equalizer, hiding the physical complexity from users by presenting them with understandable business terms and business-friendly data instead of database schemas and raw SQL. As you can see in the architecture below, with a proper semantic layer, you get a centralized repository of business terms and a data virtualization engine that makes data access ubiquitous for any analytics consumer.
(Source)
CALLOUT QUOTE “Self-service isn’t just running ad hoc SQL queries and creating dynamic Tableau dashboards,” a data and analytics leader at the company said. “It’s really important to make sure that we have good trust in our data and we have the ability to find that data.”
Governance Creates Data Transparency
When it comes to self-service, the food manufacturer also found that governance is critical. A key part of data governance is documenting what data models actually mean for both technical users and business partners. With clearly defined performance metrics available via a semantic layer, different business units and departments could focus on the insights derived from a centralized data source rather than reconciling what numbers represent from a disparate set of systems.
As the company faced a sudden spike in demand during the pandemic, its investments in digital technology and data self-service paid off. In fact, the company’s central data hub was able to define a single source of truth that improved the organization’s overall data literacy and consistency. Now business users view data as a strategic asset rather than a cost center.
Today, this leading food manufacturer considers its new modern data architecture and strategic priority on data as key factors for driving cost-savings and revenue generation going forward.
SHARE
Case Study: Vodafone Portugal Modernizes Data Analytics