February 2, 2023
What Is an AI Center Of Excellence?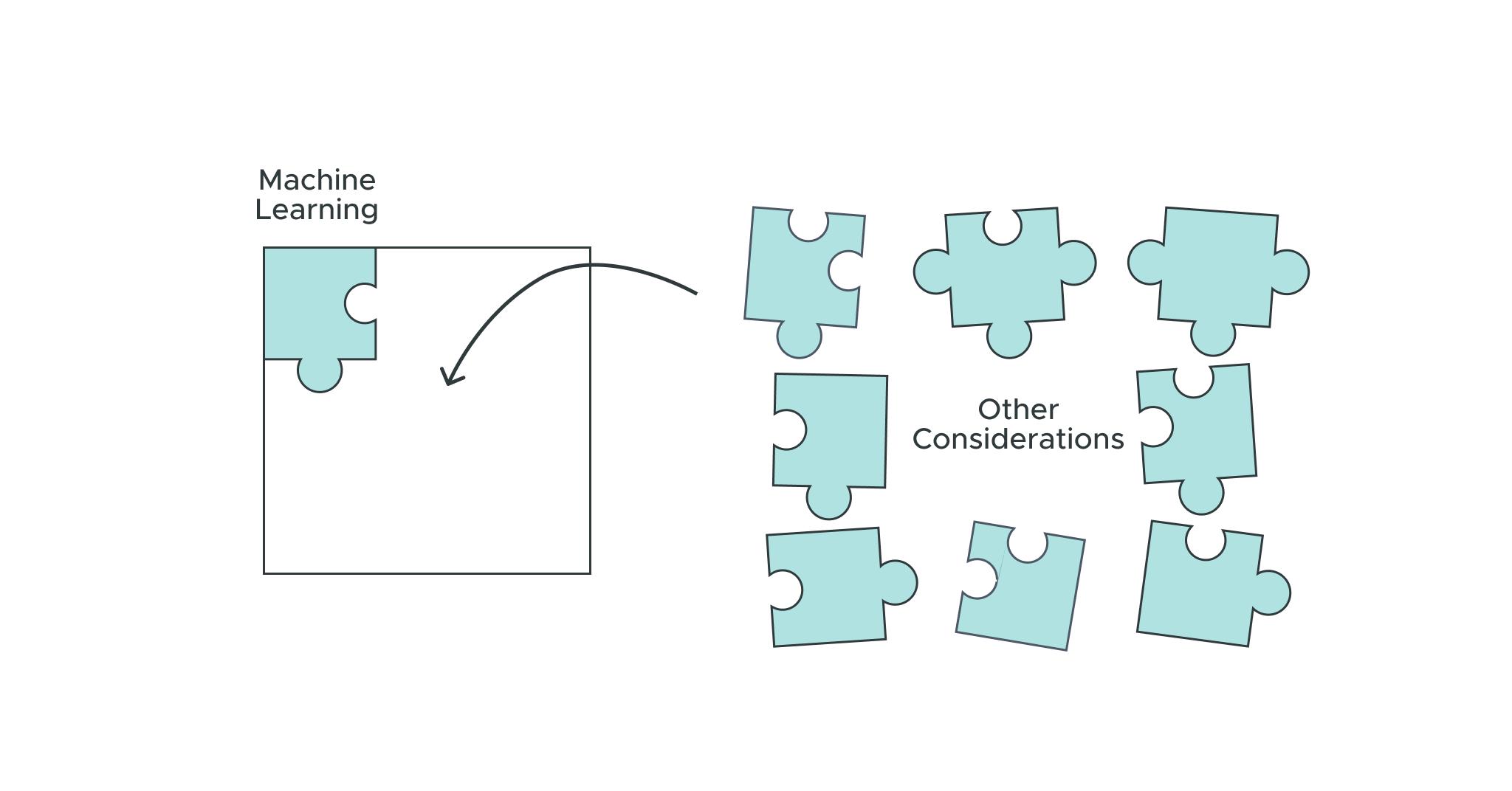
We kicked off our inaugural AtScale AI Innovation council today discussing a hot topic: Ops for MLOps: Best practices and processes to expedite model creation and reduce the need for significant re-training. We had a fantastic group of members dive into the core challenges related to advancing ML initiatives in the right way so that the attention of ML development and MLOps is centered around addressing central business challenges.
We were joined by:
- Dr. Amita Kapoor, Head of Data Science at Diggity.io;
- Anurag Singh, Senior Artificial Intelligence Engineer at Kimberly-Clark;
- Cheryl Howard, Principal Data Scientist at IBM;
- Brian Prascak, Co-Founder, Chief Insights Officer at Naratav;
- Greg Joondeph-Breidbart, CTO & Founding Member at Vanna Health;
- Jett Oristaglio, Former Data Science and Product Lead, Trusted AI at DataRobot;
- Danny Chiao, Software Engineer at Tecton.
Here are some of the takeaways from our discussion with these data science and AI experts.
Establish Key Goals and Baseline Measures
We started by articulating these two hard truths: machine learning initiatives take time, effort, and investment and machine learning is not the solution to every business problem.
There is a tendency these days for the enterprise to invest in what is still seen as ‘high risk, high reward’ efforts by using machine learning to solve an inherent problem a business is facing because:
- Businesses want to find the next key insight that will help them increase their competitive edge;
- There is continued market pressure for every business to institute some sort of ML practice in fear of falling behind. Even despite this pressure, Gartner predicts up to 85% of AI projects will not deliver.
This often results in businesses trying to force fit ML models to address a problem, leading to diminishing returns or a lack of positive impact to the bottom line.
Key Finding: It is critical for businesses to clearly articulate the business problem and key goals. Without this, data scientists cannot effectively define the agenda, timelines, or solution to the problem with an ML model. This lack of forethought results in projects getting stuck, sidelined, and not implemented. Business teams become frustrated by the lack of impact to their bottom line, not knowing they actually obfuscated the problem in the first place.
“The number one issue is clearly defining the business problem.”
– Cheryl Howard
Key Finding: Once a problem is well understood, and the agreed approach may be to use ML, that doesn’t necessarily mean it’s the long-term answer; data and the constituent business challenges inferred are constantly changing. Data scientists need the runway to experiment and create a proof point model demonstrating that the technology is well-suited to address the problem.
“There has to be a baseline model which can prove that this hypothesis, prediction, classification, or clustering using this sort of data is possible. These sort of baseline models are the sort of proof of work before going to any sort of higher level.”
– Dr. Kapoor
Key Finding: This initial model proving the technology won’t matter how and where it’s implemented if there’s no baseline measure to compare it to. If there are no existing metrics, KPIs, or the data is insufficient, there is not a clear way to benchmark and articulate progress pre and post-model development. So even with performant models, without a shared understanding of ROI and its measure, ML projects will die on the vine.
“If you don’t have a baseline of how well the process is working right now, you can’t measure whether your ML models are making a difference or not.”
– Cheryl Howard
“ML initiatives can take a year to get into production and deliver business value. Business leaders will be looking for how you can deliver value in the next 3-6 months; that baseline can actually prove some of that business value for the organization to show that this data science team can be trusted to build something that will generate business value.”
– Danny Chiao
Enable Data Preparation Efforts
Data preparation still remains a crucial element of successful ML development, but it is often the most tedious, unglamorous, and therefore glossed-over aspect of the ML workflow.
“Data engineering is the building block for data science”
– Anurag Singh
Key Finding: Data scientists need to be given the right runway to focus on data preparation and exploration. This effort still constitutes about 80% of the effort in the ML workflow, and while new automation tools are helping to address this, the time it takes for objective preparation and investigation of data is still not well understood by business decision makers.
“The sexy part of algorithms is such a tiny piece of data science; it’s what data scientists dream of doing but it’s only about 10% of the work.”
– Cheryl Howard
“Educate the business; maybe some of these problems may take a year or longer to solve by really understanding the data and the context to make meaningful progress.”
– Greg Joondeph-Breidbart
Key Finding: Data scientists need the right support vehicle around them to do data preparation the right way. While the data scientist is the center of attention when it comes to the model building process, the fact of the matter is that they need the right team construct from other roles in the organization – data engineering, data governance, analytics operations – in order to optimize their efficiency and efficacy.
“It’s really important to ensure you have the right skills supporting the data scientist because if they’re spending all their time doing the data wrangling, they’re going to be disappointed thinking they’re not doing what their actual job is: data and ML modeling.”
– Brian Prascak
Focus On Solving the Problem, Not New Technology
Because so much attention has been devoted to using ML to solve business problems, there’s been a lot of attention and investment devoted to creating the newest generation of ML algorithms.
“We call that the airline magazine syndrome.”
– Cheryl Howard
There’s an easy tendency for anyone to get excited about the next generation of ML algorithms, but just as important as articulating the business problem is using the data of your business to guide the choice of the right algorithm to solve the problem.
Key Finding: Sometimes the simplest model is enough. When the technology has proven to solve a business problem, it’s crucial for data science teams to be coached by those who know the data and know the foundation of the problem: focus on the aspects of the data that influence model training, testing, and inference rather than devoting effort in trying to implement a complicated model. Adopting a data-centric vs. model-centric approach to data science team efforts will yield more successful results for the business.
“Making sure the data science team is super aligned with the value of the data coming out of the model; what’s the simplest model, what’s the least amount of data that we can build to get a real serious lift over our current processes.”
– Jett Oristaglio
Support Data Literacy and Transparency Across Teams
It’s often the case that the need for delivering results fast is detrimental to the efficacy of an ML project. The data scientist is seen as the master of keys for new solutions to address business challenges, but their success is encumbered by two key problems:
- Data scientists don’t control access to the right data and its associated context (lineage, associated DevOps protocols, metric definitions).
- Data scientists are the centerpiece of all ML initiatives, but their work is often performed in a silo.
Key Finding: Data scientists need help from their peers to leverage the same view of data that would be used to train an ML model as it would be to train a data model for business intelligence. The traditional tendency for a data scientist is to take extracts or copies of larger datasets so they can train and test models without overpowering the CPU on their local machine. These siloed views of data can start a snowball effect in the standardization, normalization, and featurization of data needed for ML training. This yields models that will require lots of effort to re-tune once it is performing inference on live data.
“I’ve worked with customers where two different groups will be briefing the same leader in the same meeting and they each have their own set of data on their Sharepoint or laptop… they’re not working from the same set of data even though they work for the exact same enterprise.”
– Cheryl Howard
“Too often I see the data science team react to the business not understanding what we do, we don’t have the business context, so we’re going to go over here and play in this sandbox… which ends up not being super valuable.”
– Greg Joondeph-Breidbart
Key Finding: Businesses and data teams need to develop a mutual, shared empathy about their responsibilities and the appropriate handoff so that there is as much transparency in the articulation of the business challenge as there is to the business problem. In addition, it is just as important for the data teams to educate the business on the economics, effort, and key development milestones so that progress is measured not just by the creation of a model. Business and data teams need to operate as an ecosystem, not in siloes.
“Communication is the key. Very often we need to have a few calls tied to data literacy. Businesses come up with use cases, but most of the teams work in silos…We have these experts who are there to help you with your use cases. So that data literacy has to come from the people involved.”
– Anurag Singh
“There is a data science and platform team disconnect where they don’t necessarily understand the challenges they are facing. It all plays into the ability for this investment to pay off for the business as a whole.”
– Danny Chiao
The Best MLOPs Practices Point Back to the Business Problem
MLOps, based on best practices from ModelOps and DevOps, has become a key center of attention for businesses implementing ML. As businesses look to scale these initiatives, the teams of practitioners become viewed more as a cost center.
Therefore, optimizing efforts, practices, and efficacy has become critical for the business. This is fantastic for the longevity of ML as a foundation of the modern business, but its manifest in many software tools out there has not yet truly solved the problem.
Key Finding: MLOps dashboards don’t tell the full story. While they monitor models at runtime to tell data scientists about accuracy, precision, F1 score (key indicators of model performance) there needs to be a way to correlate ongoing improvements to model performance and key findings that influence business decisions.
“Automated alerts for something that is going on is important, but I’d be interested in an MLOps system that automatically builds insights about the business value that they’re generating; they can define the business problem in some way, even if it’s just documentation so they can see that when they go into the data and analytics page… automated reporting for how this fits into the business problem itself.”
– Jett Oristaglio
Key Finding: ML still continues to only tell one side of the story.
“Explainability is really critical, traceability is really critical, and cognition is really important too. There needs to be a way for people to feel comfortable actually taking action from predictions and how the analytics blend in with other insights in the business. It’s not an island; predictions are part of understanding the business and where the marketplace is going.”
– Brian Prascak
Stay tuned for upcoming meetings and intellectual capital from this team. If you’d like to participate in the dialog, don’t hesitate to reach out directly, zach.eslami@atscale.com.
RESEARCH REPORT