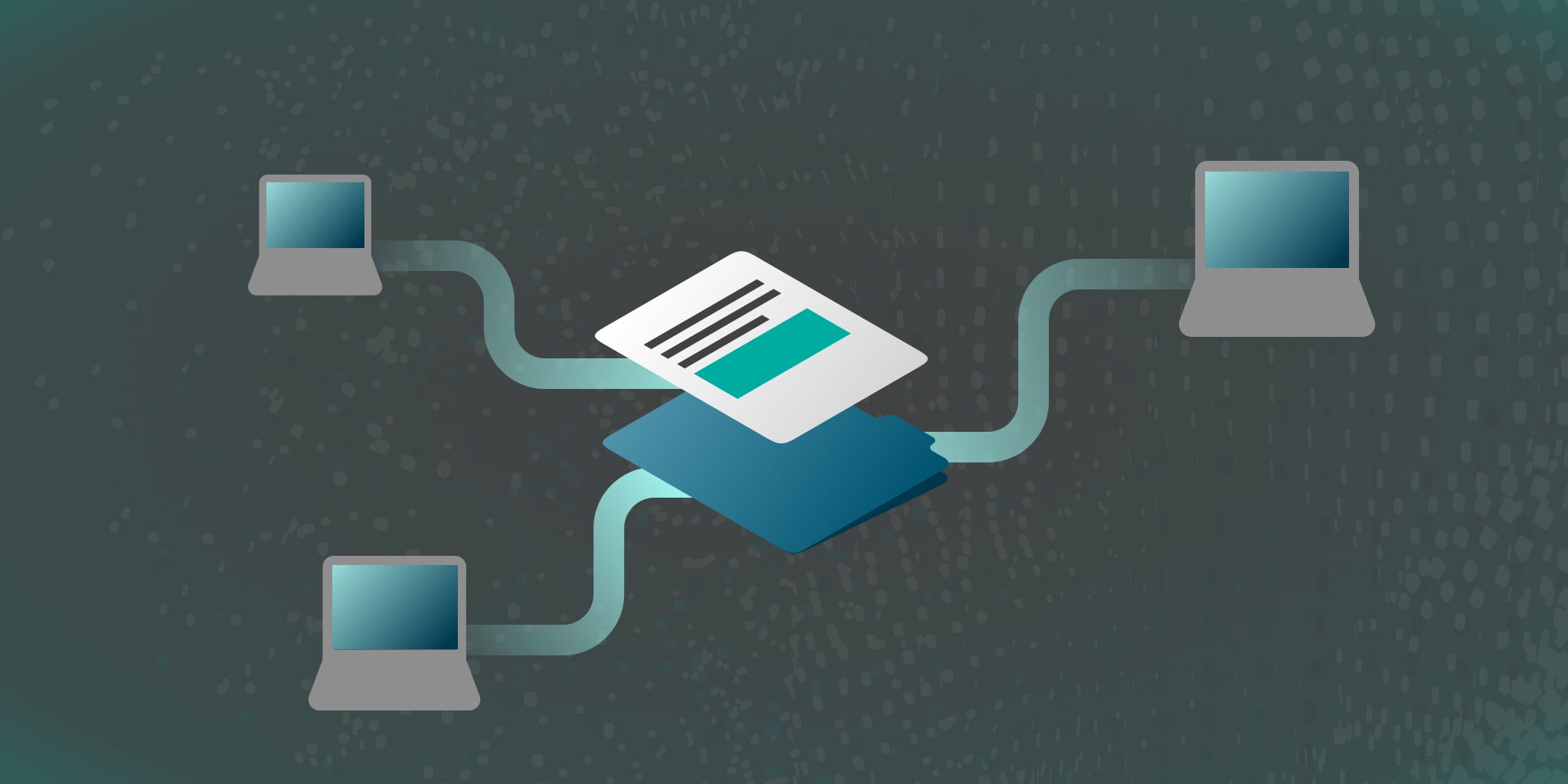
I was recently asked “what advice do you have for data-driven leaders on making smarter decisions at scale in their organizations?” I thought it was a fantastic question and there were so many things that came to mind from X [with self-service analysis stack/strategies, data empowerment, (list some stuff from Retail Analytics Notes)] to Y. But, what has particularly stood out in recent customer conversations with some of our more advanced data and analytics leaders who are making the most of their centralized data through different modernization and democratization initiatives – is a notion of ‘data-sharing’ with both internal and external datasets.
Before I jump into the concept of data-sharing and how the organization that I’ve talked to have operationalized it – I’d love to hear “what advice do you have for data-driven leaders on making smarter decisions at scale in their organizations?”
When I think about the winners and losers of data & analytics initiatives, a pattern becomes crystal clear: the companies who have invested in a self service, trust-based, data driven culture outperform their cohorts.
As we speed down the digital transformation highway, the culture of data sharing is becoming the new differentiator. Gone are the days when IT can spoon feed carefully curated data to the business and gone are the days where data is held close to the chest. According to Gartner, by 2023, organizations that promote data sharing will outperform their peers on most business value metrics.
In this paper, we’ll examine the ways in which organizations can create a data sharing culture both within the organization and with external stakeholders. We’ll also look at the technical requirements to promote and operationalize data sharing while encouraging technical stakeholders to align their interests with business outcomes.
3 Models of Data Sharing
Data sharing is more than just sharing files, reports and dashboards with your peers. There are different models of data sharing and we’ll explore the unique characteristics of each in the next section.
First Party Data Sharing
First Party data is the information your organization collects directly from your business activities and customers.
First Party data sharing is probably what comes to mind to most when you hear the term “data sharing”. First Party data is internal, proprietary data that’s not meant for external consumption.
At first glance, it should seem obvious that First Party data is shared freely within an organization. However, internal data tends to be siloed and stored in multiple, business process specific data stores. For example, financial data may be locked away in a ERP system, sales data in a CRM system and employee data in a HR platform.
On top of the physical data silo challenge, for many enterprises, business units drive the decision on tool and technology selection. While this level of autonomy accelerates progress in the early days, the business often buckles under the pressure of data volumes and lack of data engineering expertise as the business scales. This data balkanization creates barriers to sharing data across departments and business units which runs counter to a data sharing culture.
The lack of data sharing has a direct, negative impact on business performance and makes organizations less agile and less adept at responding to market changes. Conversely, adopting a “data as a service” strategy promotes autonomy through self service and frees up business users to focus on improving business performance rather than wrangling data.
Second Party Data Sharing
Second Party data is First Party data that two or more business partners decide to share on a “private” basis for mutual benefit.
In today’s environment, internal data sharing is table stakes, a given. Increasingly, the most successful organizations are finding ways to share data with their business partners and stakeholders.
The retail industry has demonstrated the powerful impact of sharing data with their business partners. By sharing inventory data with their suppliers and vendors, retailers can enable their business partners to effectively manage inventory on their behalf. The two parties incentives are aligned: the retailer wants shelves stocked with the right products for the right markets and the supplier wants to push as much of their product as possible through their channels.
The marketing automation software industry is another great example of the power of Second Party data sharing. In today’s e-commerce world, consumers leave digital breadcrumbs across the webosphere. This data is captured by dozens of third parties and stored in thousands of proprietary databases. Marketing software vendors often share this data with other marketing software vendors so that their joint customers can construct consumer profiles to improve targeting and sales.
By sharing data with partners, organizations can scale their reach beyond the corporate walls to better serve customers and drive operational efficiency.
Third Party Data Sharing
Third Party data is information that is collected from a variety of websites and platforms and is aggregated by an outside entity.
Leaders in data and analytics are finding ways to integrate Third Party datasets to enhance their First Party data to create unique, competitive advantages with data. Gartner highlights this trend, predicting that by 2023, 85% of data sharing strategies that include external data sources will drive revenue generating digital business outcomes.
During the time of COVID, we’ve seen a wide range of Third Party data consumption via data marketplaces like the AWS Data Exchange make a real difference in gauging demand and predicting sales. From retail places data from Safegraph to Weather-Driven Demand for Ice Cream from Planalytics, there’s something for every business. These new data marketplaces make it easy to browse available datasets like you would browse products on Amazon.com, with free and”try before you buy” options available.
When organizations combine these “data enhancements” with their own data, they can create new business insights and improve their ML and AI models.
How to Create a Data Sharing Culture
There are a number of barriers to sharing data as you can see in the Gartner survey below.
Image 1: Gartner Data Sharing Survey
These barriers fall into 2 main categories:
- Data Sharing Mindset
- Data Sharing Information infrastructure
The Data Sharing Mindset
There’s a natural tendency of most organizations to be fearful and suspicious of sharing data. IT has traditionally rabidly protected access to data for security, privacy and regulatory reasons. However, sharing data, whether it’s interdepartment, with strategic partners or with data marketplaces, is becoming a key capability for creating enterprise value.
In order to break away from the tendency to protect data access at all costs, organizations must recast their data management practice from an IT function into a business function. By making data management a key business capability, enterprises can transition into what Gartner calls a “Must Share Data Unless” data sharing model. In other words, organizations should assume that every piece of data may be exposed to others,either internally or externally, so proper safeguards and processes need to be in place at data collection time. By creating a default, “share everything” mindset, the business can decide whether, what and when to share data to create a data agile enterprise.
The Data Sharing Information Architecture
The more obvious barrier to data sharing is a lack of technical infrastructure suitable for data sharing. For a successful data sharing strategy, it’s imperative to abstract away the physical location and format of enterprise data so that analytics consumers, internal and external, can access and blend data that spans across multiple business processes. There are several data integration strategies for accomplishing this feat from data virtualization to creating a centralized data warehouse. In either case, it’s essential to create a business-friendly semantic layer with integrated security and governance.
The diagram below illustrates how a universal semantic layer can be a fundamental building block to enabling all three methods of data sharing.
Image 2: A Data Sharing Information Architecture
Equally important is driving data literacy. Without a map to where the data is and what it means, it’s tough to share data with anyone. Tools like enterprise data catalogs can help drive data literacy by documenting data sets, standardizing on business terms and creating a centralized glossary.
Taken together, a universal semantic layer backed by an enterprise data catalog can serve as the data sharing hub for the enterprise. With this single data control plane, data can be shared with the requisite controls in place to create the confidence and trust needed to drive a data sharing culture.
As you can see, data sharing can take many forms and the benefits are transformational. Whatever mode of data sharing makes sense for your organization, it’s imperative to create the right culture and infrastructure to support sharing. By treating data as a core asset and competitive differentiator, enterprises will not just survive, but thrive in difficult business environments like we witnessed with COVID-19.
Power BI/Fabric Benchmarks