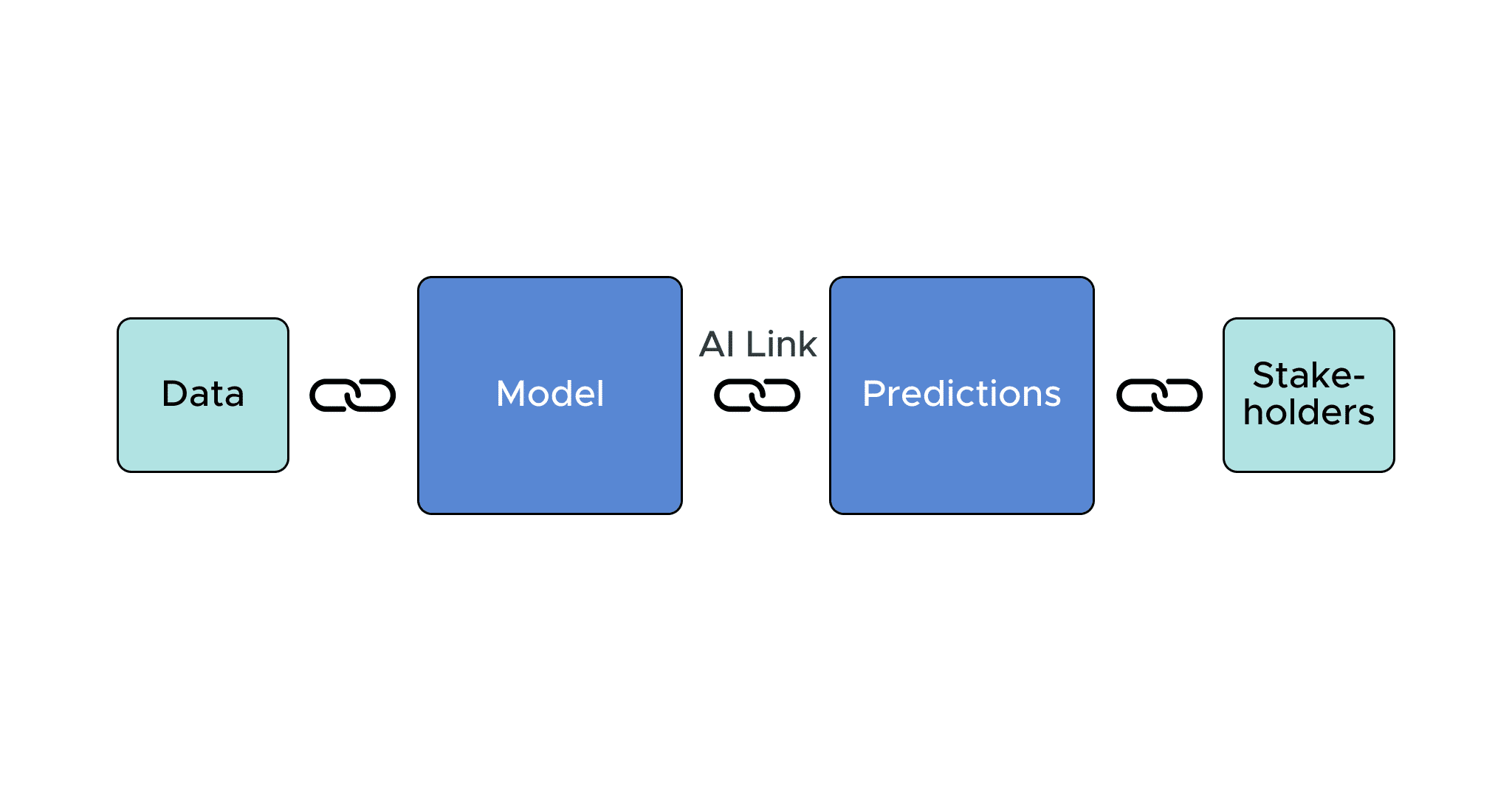
How AI-generated Insights with BI Tools Drives Successful Adoption and Next-Level Business Analytics, Data Analysis, and Decision-Making
AI Projects Fail Fast and Often
An estimated $500B in worldwide spend on AI in 2023, and seven in ten US organizations are devoting $1M or more of their budget to AI. Despite these facts, over 85% of AI projects are not yet generating business value nor are they expanding the potential of business analytics, data analysis, and decision-making.
Successful adoption and implementation of AI is not the same as adoption of other modern cloud-based technologies. Beyond the concerns related to the presence of bias and the potential impact of AI deployment that can emerge as a result, there are many challenges that businesses face restricting how and where AI is used:
Time-to-Value: It takes companies 90+ days to deploy an AI model into production, months to create multi-source datasets, quarters to deploy a trained model, and years to see new value from this investment.
Ineffective Spend: While 92% of businesses with existing AI investments are increasing their budgets in 2023, many of them are devoting capital to inefficient computation, highly skilled AI experts, and data governance.
Friction Among Teams: Only about 50% of AI models make it to production because of disagreement over data source and metric definitions, as well as distrust and misunderstanding of output.
However, these shortcomings have produced valuable insights for others to learn from and achieve value from their AI investment.
- Businesses will need to leverage one source of truth for their data and metrics. Executives understand the need to bridge the gap between AI and BI, and the semantic layer is a crucial element to make data and AI speak the language of your business
- Data scientists will need to play an important role in the design and development of models. They will need to work closely with Business Analysts to make predictions available and well-understood. Business analysts outnumber data scientists nearly 14 to 1, meaning they represent a crucial stakeholder to AI’s successful advancement.
- Subject matter experts must be empowered with the functionality and low-learning curve tools that allow them to experiment with and use AI. We need to uplift those who have business knowledge and context but might not have the technical skills to consume and use AI in data analytics.
Put AI in the Hands of Domain Experts
AI predictions need to be consumable, relevant, and explainable so that domain and business analysts can drive more strategic business analytics, data analysis, and decision-making from the work of the data science and ML engineering teams.
Traditionally, ML prediction tables have been large-scale denormalized data tables that are typically captured as screenshots only interpretable by the model builder. These datasets are often too large and in an unsupported format that cannot be loaded and analyzed in common business intelligence tools.
Plus, they lack an immediately visible correlation back to the business problem: Business analysts need an easy way to easily access ML predictions and a quick way to evaluate data science model performance using the freshest data.
Consider this common business challenge: Understanding customer churn. Machine learning is a great tool for predicting the likelihood of risk of particular customers who may not seek renewal or expansion opportunities. A single point-in-time view of predictions based on historical data can generate a brittle table that does not enable the discovery of key insights needed to influence inform the right business strategies.
The business will want to know not only if customers are going to churn, but also:
- At what point in the year;
- In which geographies;
- For what product sales.
Dimensional exploration of large prediction sets is crucial to uncovering the value of AI, which a simple analysis and discussion of a table screenshot cannot provide.
Ultimately, there needs to be a way to inherit AI predictions and metadata in Business Intelligence tools.
The Key to Better Business Analytics, Data Analysis, and Decision-Making: Leveraging BI to Successfully Scale AI
As part of the latest news from AtScale, AI-Link 2.0.0 is introducing key new functionality to empower data teams to work together using AI to solve business problems, and businesses to make the right decisions from AI output.
AtScale AI-Link, now offering PySpark support as part of the 2.0.0 release, fosters a Semantic Predictions (formerly “Writeback”) capability that makes it super easy to get the results of large-scale AI models into the hands of business stakeholders. That way your teams can leverage existing data platforms and BI infrastructure to make key decisions based on proven ML models.
Here’s the bottom line: We offer the best, most performant mechanism to aggregate, normalize, and package AI models and predictions. That all happens through the semantic layer so companies can make AI tap into stronger business analytics, data analysis, and decision-making – and speak their business’ language.
This means:
- More models generated
- More models moved to production
- Greater value driven
Want to see for yourself? Sign up for a free trial of AtScale AI-Link by sending an email to ailink@atscale.com.
SHARE
RESEARCH REPORT