April 13, 2023
Actionable Data Insights for Improved Business Results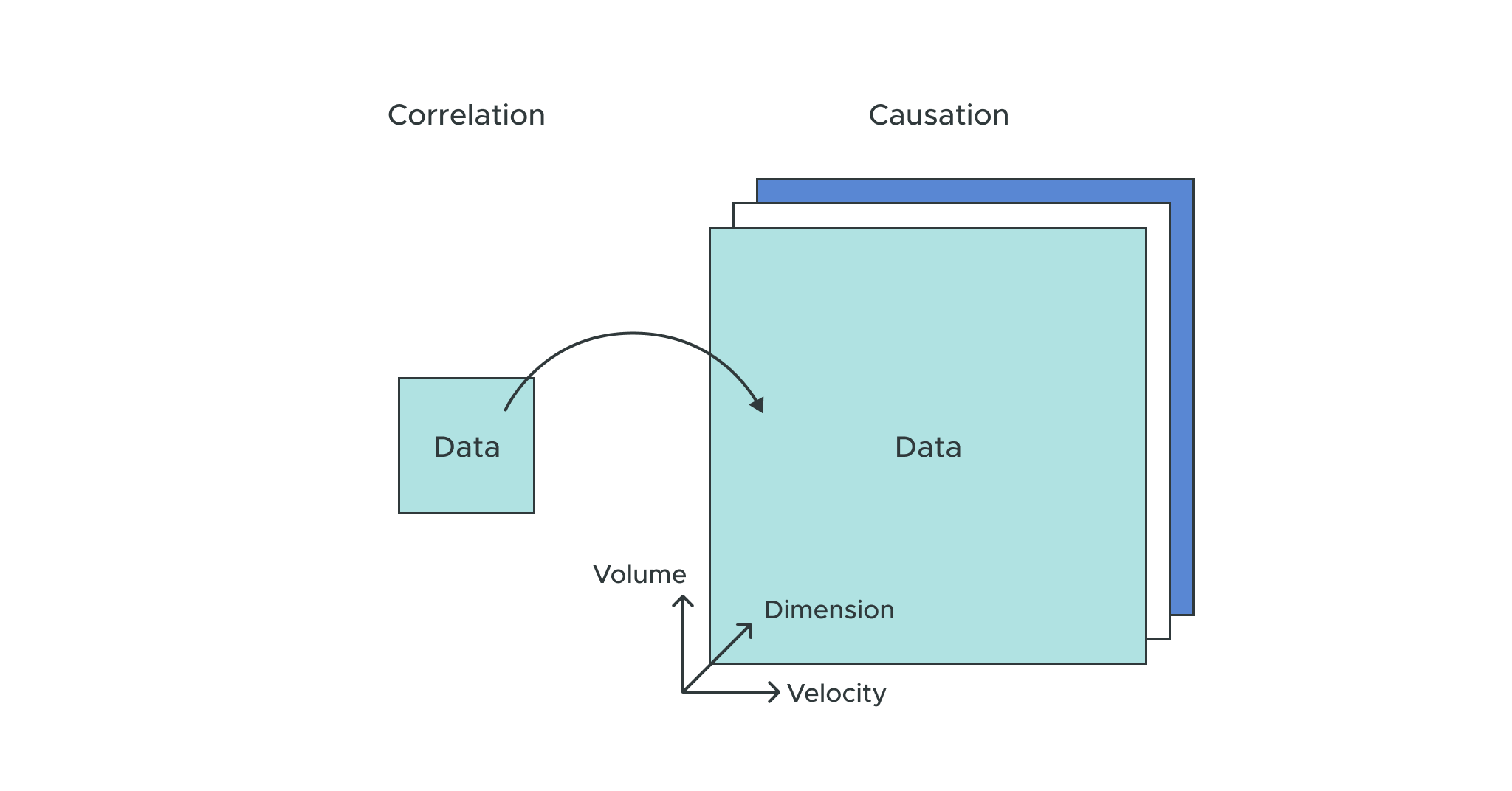
John K. Thompson, Analytics Leader, Innovator, and Author of Building Analytics Teams and Data for All.
Currently, when talking about Artificial Intelligence (AI) in the context of enterprise class, commercial companies, it is more of an art than a science. Why is this the case?
AI is a part of Data Science. It says it right in the name, Data Science. The science element of Data Science and AI is a moving and evolving target; that is not a bad thing, it is just the state of the art today and a fact that we should understand a bit better.
The art of analytics, including Data Science and AI, is due to a number of factors:
- AI is a relatively new discipline – the math, technology, and tools to build, manage, and operate an AI-enabled infrastructure are nascent and rapidly evolving.
- The skilled professionals who can effectively and efficiently conceive, build, and maintain AI applications are in limited numbers, and those professionals are trained in a variety of different schools of thought and approaches to the craft.
- The nascent approaches that are showing promise in research and academic labs need to evolve to work in commercial settings.
The amount of innovation that is being developed is promising and exciting, and provides a conceptual foundation for commercial software, robust models, and applications, but there is more work to be done before we can move from art to science.
Today, the effectiveness and efficiency in the art of Data Science and AI is substantially dependent on the skill and ability of the data scientists or machine learning engineers involved. Specifically, it is reliant on the ability of those professionals to effectively conceive, design, build, and execute the feature engineering phase of their projects.
Much of the success of current feature engineering work comes down to mastery of statistics in general and creativity with using correlation in specific situations.
Let’s discuss how Correlation is used today in AI and Data Science projects.
What is Correlation and How Do We Use it Today in Data Science and AI?
Let’s define Correlation:
Cor·re·la·tion /ˌkôrəˈlāSH(ə)n/ – a mutual relationship or connection between two or more things.
Data Scientists work with a broad set of features that are correlated to the variable or the measure that they seek to understand, monitor, or predict. There may be thousands of possible variables that are correlates of the target measure. Actually, in some cases, there may be millions, and possibly billions, of correlates.
The art of the analytics process – and to be clear, there are very few people who are highly skilled at this part of the AI and Data Science procedure – is to determine which of those thousands, or millions, or maybe even billions, of variables will accurately, robustly, and reliably predict the actions and behaviors of the target variable or variables in close proximity to the observed behavior in the real world.
Over the past 37 years, I have worked with a small number of people who are world class in this area (less than 20). If AI and Data Science are to grow and be an integral part of every leading company, we cannot rely on a process that only a handful of experts can execute.
That is Why We Need to Move from Correlation to Causation
Let’s define Causation:Cau·sa·tion /kôˈzāSH(ə)n/ The relationship between cause and effect; causality.
Simply moving from correlation to causality does not change the AI and Data Science process into a repeatable, rigorous scientific process. It will take more than a change in focus or methodology to get us there.
We are at an inflection point where causal algebra is being proven in academic research labs today. Those academic efforts are being quickly followed by early-stage commercial companies that are building software and tools to leverage these research innovations so that companies can implement causal feature engineering.
Developing the appropriate and purpose-built math, tools, and technologies is what will enable the changes needed to replace the current fallible feature engineering process with a science-based process. In doing so, a significantly wider population of analytics professionals can undertake the task of developing casual based analytic applications on a reliable, repeatable, and scalable basis.
Casual algebra is being built into tools appropriate for Data Scientist and Machine Learning Engineers. These tools and applications will improve upon and replace the current process of searching for correlates on a hit and miss basis with a measured, controlled process to look at each possible feature and combination of features to ensure that our causal features, models, and application are the best that they can possibly be.
This new and improved process will look at every feature and every combination, and test them against the target to provide intelligence and transparency into the fit for the objectives of each project. The process will result in a set of features that we know to be the best available, not just the best we happened to find.
Why is Causality the Next Step in Our Data Science and AI Journey?
Causality gives organizations the ability to move from the current highly variable process that is dependent on a small number of highly skilled professionals in severely limited numbers to a scalable, repeatable process that is available to all analytics professionals. This produces scientifically verifiable casual factors in relation to the phenomena that we want to understand, predict, and influence. Now that is a game changing development.
Moving from an environment where we think we have done the best job possible in finding the optimum features, to one where we know that we have the optimum features is a defining development that will have an impact in every industry and company that is leveraging Data Science and AI.
We will still need trained and skilled analytics professionals, there is no question about that. But with the coming advances into Causal AI, we will be able to bring additional people into the analytics process and still maintain a high level of confidence that we are building robust, reliable, and flexible models and applications. We can grow the AI and Data Science fields at a much faster rate.
Causal AI not only provides the data science and AI communities with a verifiable scientific process, but it also brings us one step closer to Explainable AI (xAI).
xAI is the ability to explain what our AI models are doing, how they learn, and why they make the decisions that they make. This opens our ability to use our most powerful analytical techniques on all analytical problems across all industries.
Until we have xAI, we cannot use our most advanced techniques in regulated industries like pharmaceuticals, insurance, retail banking, and more. Causal AI will accelerate the development of xAI.
How are Correlation and Causation Supported and Improved by the Semantic Layer?
The current correlation-based feature engineering process is supported and extended by the Semantic Layer. Even with a handful of features, monitoring the changes in the source data sets, the derived data sets, the feature engineering process, and changes in model performance (including model degradation measurements) is a daunting task.
The Semantic Layer adds an environment where these metrics and measures can reside and they can be managed. Then they can be used to understand the past, current, and future state of our analytical models, applications, and environments.
When we move to a Causal-based feature engineering process, the number of factors will increase exponentially. Instead of considering a few hundred or thousand variables, our systems will be evaluating millions, or maybe billions, of variables and possibly even more since the environments will be automated. Without the limitations of people executing the feature engineering process, the possibilities can be considered limitless.
Considering the amount of data that will be ingested and analyzed, the Semantic Layer will be crucial to maintaining an understanding of the past, current, and future state of the analytical environment in total, and having a clear picture of the components of the analytical models, the source data, and features that those models are leveraging.
The Semantic Layer will be where the changes in data, models, and applications will be logged and maintained. It will also be the core repository for all the information that supports the scalable, automated, robust implementation of Causal AI, which will lead to xAI.
The Semantic Layer is the cornerstone in the foundation of these exciting developments in the AI and Data Science markets.
The Next Steps in Data Science and AI: Causality Supported by the Semantic Layer
Our data science and AI environments are scaling quickly and enabling the inclusion of an impressive breadth and depth of data for use in our models and applications.
The innovation of causal algebra and causal feature engineering will drive the scale and speed of Data Science and AI to even further heights. These developments are creating new opportunities to take Data Science and AI into new operational areas for all industries.
When the Data Science teams are asked about the velocity, variety, and veracity of the data being used, it can be challenging to answer the questions concisely and with a high degree of insight and confidence. The Semantic Layer is the perfect complement to this new high velocity, high volume, and high dimensionality approach to Data Science.
As we move forward with these intriguing developments in the analytical world, we will see the Semantic Layer there in lock step with these new and interesting applications.
ANALYST REPORT