April 13, 2023
Actionable Data Insights for Improved Business Results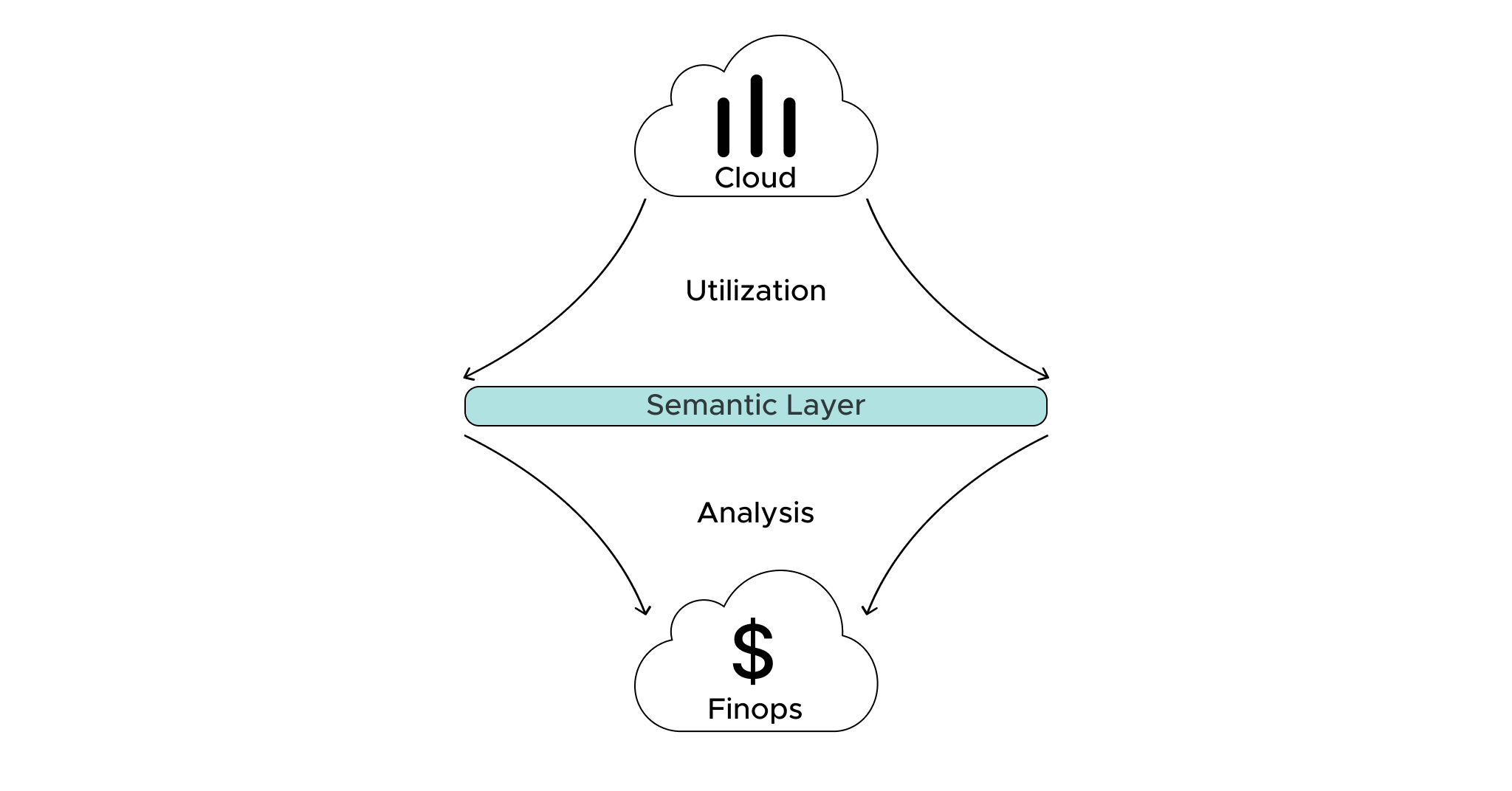
I confess. I had heard the term FinOps batted around for the past few years, but I hadn’t really absorbed its significance.
I started paying more attention after reading some research from Gartner Analyst Adam Ronthal. I was able to catch two of his sessions at the Gartner Data and Analytics Summit in Orlando:
- Optimize Your Cloud Spending Strategy for Uncertain Times
- Financial Governance and FinOps in Cloud: Avoid an Unpleasant Conversation With Your CFO
After connecting with folks from the FinOps Foundation, we spoke about the term’s history and how to apply its methodology to data and analytics. What I’ve learned has made me even more excited for our panel at our upcoming Semantic Layer Summit featuring AJ Nish from the governing board of the FinOps Foundation.
That’s when I got thinking. The semantic layer in the modern data stack can establish a new hub for understanding and managing cloud resource consumption, as well as the costs associated with data and analytics. In other words, we can use the semantic layer to analyze FinOps in organizations.
The Semantic Layer Positioned as a Hub for Gathering FinOps Data
The semantic layer sits at the intersection of the cloud data platform and the consumption layer. When implemented properly, the semantic layer is a thin control plane that coordinates data exchange between users working with various data products and data managed within a cloud data warehouse or lakehouse.
The semantic layer maintains business context by managing descriptive metadata and governed data models that properly blend disparate datasets with common dimensions. These dimensions include time, product, and geography.
There are different approaches to implementing a semantic layer. AtScale believes data should not be persisted outside of the data platform. All transformations and queries are executed on the cloud, delivering the results of a user’s query only to the data product where it originated. This means the semantic layer “sees” all queries and run-time transformations that result from end-user analytics.
An effective semantic layer will support a full range of data products — from BI dashboards to data scientist notebooks, to embedded analytics and natural language analytics. Queries generated by the consumption layer tool are passed through the semantic layer to be “translated” and routed properly to the underlying cloud data platform.
The semantic layer orchestrates query execution on the data platform. It also has visibility into the frequency of queries, the size and structure of underlying datasets, the number of rows scanned, and the size of the datasets returned. In this way, the semantic layer also “sees” all cloud resources utilized while delivering a single query.
When the semantic layer enforces access control policies and integrates user credentials between the consumption layer and the data platform, it enables a rich set of active metadata on analytics usage that can support FinOps. The semantic layer now not only sees the bi-directional query traffic between cloud and data products. It can also track which data products drove what query volume, what users initiated queries, and what specific data elements (i.e., which KPIs) were the subject of the queries.
Gain Visibility into Analytics Usage and Cloud Consumption
Leveraging its unique position in the data stack, the semantic layer becomes a hub for analyzing usage and cost data associated with data and analytics programs. This essential data can be used to support FinOps initiatives.
Enabled with new financial data, data teams can set up tracking to better understand query volume (query frequency, queried terabytes) by user, by work group, by data product, and by consumption mode (e.g., Power BI dashboard vs. an embedded analytics widget). It’s difficult to have this visibility from the amorphous blob of cloud spend that most data teams must explain.
As costs rise, FinOps efforts can provide managers with a breakdown of how different analytics initiatives are contributing to overall spend. The semantic layer can make this a lot more seamless than keeping messy books and records — or outsourced FinOps.
Integrating a lens on FinOps in data and analytics will be more important than ever. Gartner analyst Rita Sallam wrote in Silicon Angle about the importance of relating business value to analytics investments. Plus, Gartner talks further about using an “Enterprise Value Equation” to create a consistent view of the “value enablers and stakeholder impacts to identify the total return to the organization.”
A consistent view of resource consumption with the fidelity to accurately assign costs based on actual usage would be a game changer for understanding the real return on analytics programs.
We also discussed the carbon footprint of data and analytics at Gartner, a topic rising in relevance and importance. Data centers account for around 2% of greenhouse gas emissions, with compute-intensive workloads (including analytics and AI/ML) becoming more of a concern. The same methodology used to track cloud costs by user, workgroup, and data product could be used to approximate compute cycles consumed in analytics delivery.
Building, Managing, and Enforcing Analytics Cost (and Carbon Footprint) Policies
We often talk about the semantic layer as enabling an important set of governance services — establishing a place to maintain consistent definitions of metrics and dimensions and enforcing access control policies at run time. A semantic layer platform like AtScale could also be used to manage and enforce policies related to cloud consumption that drive variable costs and carbon footprints.
What’s the key to effectively leveraging the semantic layer here? To use the data described above in establishing a baseline of acceptability for resource consumption. Building institutional knowledge on what is a reasonable expectation for cloud spend helps data product designers set goals for their user communities.
To be clear, this is not as easy as equating less consumption with better consumption — some data products may deliver so much value that the organization can tolerate surges in spend. Conversely, not all data products require “speed of thought” query performance.
Many analytics experiences are not degraded if results are returned in a few seconds. By intelligently allocating resources to those data products that require high performance and those that do not, data teams can better manage their enterprise value equations.
This data could also be used to optimize infrastructure to better support usage patterns. For instance, AtScale automatically generates aggregates based on usage patterns. Building aggregate tables consumes compute cycles but can radically reduce time and cost. That’s because “agg hits” reduce the need for costly full-table scans.
At the end of the day, data teams need to architect pipelines that support the full array of data products supported. Whether or not a team leverages automation, usage data can establish policies and prioritize initiatives based on better consumption. Taking a more proactive approach to managing cloud costs is exactly what FinOp practices are all about.
How Do I Put This Into Practice?
Good question. AtScale’s implementation of the semantic layer does much of what I described “out of the box.”
We use active metadata in the form of usage patterns to dynamically orchestrate cloud resources to optimize performance. Admins can watch the AtScale query log to see query frequency by user and better understand the effectiveness of aggregation logic.
We are actively researching the intersection of FinOps and the data stories told by semantic layers. In addition to a dedicated panel on the subject at Semantic Layer Summit 2023, we have another panel exploring the subject of Data Products and Data Product SLAs.
The idea of establishing Service Level Agreements (SLAs) for data products is important for scaling data and analytics. Data product SLAs should explicitly detail the response times and expected query volumes, as well as the type of data and governance needed. Leveraging the semantic layer as a hub of cost and usage data can facilitate governance and monitor SLA compliance. With a better understanding of the cost of delivering on an SLA, organizations can more accurately evaluate value creation across different data products.
A semantic layer unlocks game-changing insights and power when it comes to FinOps. We look forward to working with our customer and partner community on delivering solutions that enable smarter FinOp practices in data and analytics programs.
Want to learn more about the semantic layer can support financial operations? Join us for the 2023 Semantic Layer Summit on April 26th.
The Practical Guide to Using a Semantic Layer for Data & Analytics