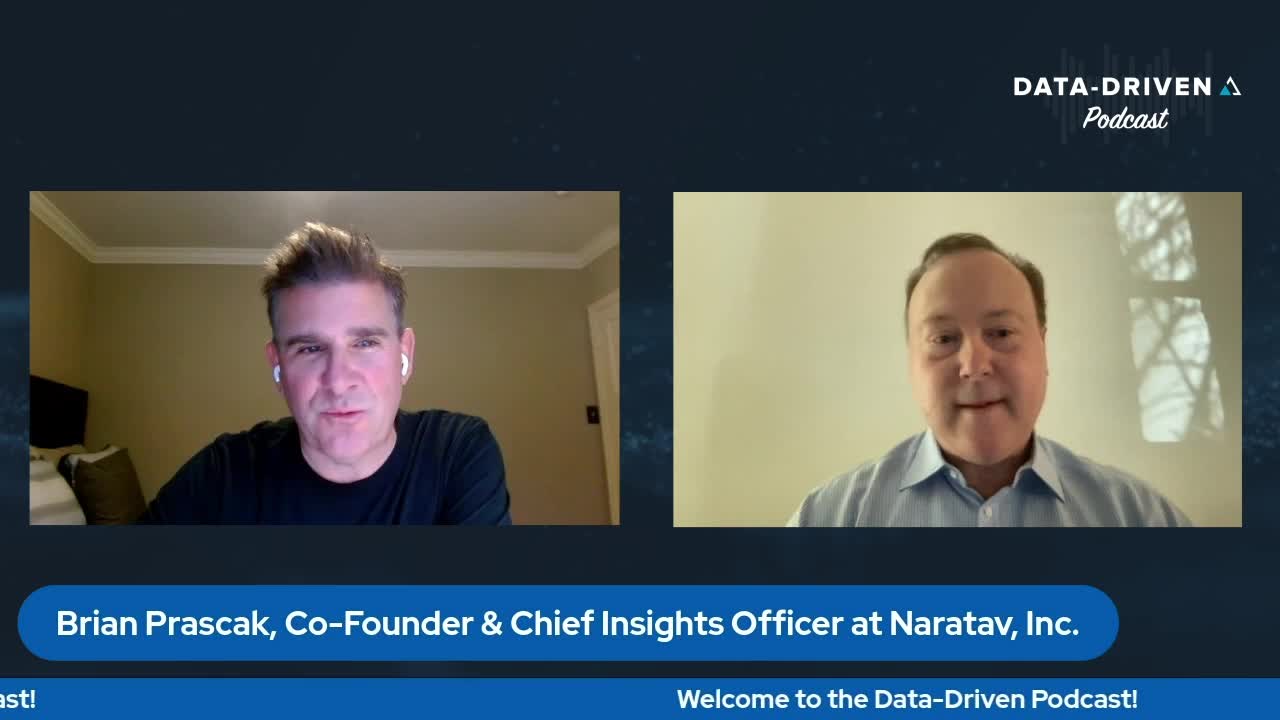
Listen to Brian Prascak talk about his experiences helping Enterprises become smarter, faster with data and analytics at JP Morgan, MasterCard, Diageo, and most recently Wawa as the Director – Advanced Analytics, Platform and Data Services. He shares his thoughts on why a semantic layer for data and analytics is incredibly important.
Transcript
Dave Mariani: Hi everyone. And welcome to the AtScale Data-Driven Podcast. And we have a special guest today. It’s Brian who’s the co-founder and chief insights officer at Naratav. Brian, welcome to the podcast.
Brian Prascak: Thanks Dave. Glad to be here.
Dave Mariani: Well, you know, I always love to start these conversations off with just, getting to know, getting to know you a little bit better. And, you know, a lot of people here are building their careers in analytics and data science, and so I’m sure they would love to hear about your journey. And so how you got to where you are today. Speaking with, speaking with me on the podcast.
Brian Prascak: No, thanks Dave. Well, it all started when I was five years old, I woke up one morning and told my mom, I know what I want to be when I grow up. No. And I said, I want to help companies become smarter, faster data insights and analytics, of course, to which my mom replied go back and work on your ETL. And maybe you need to work a little more on your ELT, but it wasn’t that early. It actually started pretty early though. I’ll be honest with you. You know, it started at university, I studied business and engineering and the engineering was industrial engineering and the area was called operations research and really operations research is the use of, probably distributions statistical techniques to model processes, simulate them and optimize them. And a lot of the core concepts today are used for data science, a lot of the probabilistic aspects and optimization and so on.
Brian Prascak: And fundamentally it’s about systems thinking and the idea of, modeling a system and learning from it. And we use data obviously to learn and that’s part of, kind of the process. So that’s where I got hooked. And then when I looked around, I could see that it was being used quite frequently in manufacturing. Of course it continues to be so today, but I thought, you know, it’d be really interesting to see more of this being used in, in business. And so that’s kind of how I charted my course and I, you know, said, I want to be part of, kind of that data insights and analytics. And that took me to working with a lot of different companies, again, like this idea of smarter, faster, and really learning from data. and so I kind of moved into a number of different companies. I started with AC Nielsen, IBM, JP Morgan, MasterCard creating information business there, Diageo in the packaged goods area and most recently at Wawa. So, and that’s taken me to Naratav, and obviously the opportunity I’ve had to work with AtScale and get to know what you guys do as well.
Dave Mariani: So Brian that’s like, those are all the companies that all have big data. So, it sounds like you’ve been involved with big data and dealing with complex environments from the very beginning.
Brian Prascak: Yes. One, one tour was through kind of the enterprise data warehouse world and kind of that, that world. And now it’s exciting to see where we are, looking at, you know, software as a service platform, as a service and really the cloud and the maturity that’s there. And we’ll talk today about, you know, how important it is to have the right strategies to make sure that the end of the day we use data to answer questions and how do we continue to make sure that we’re utilizing these technologies in a way that gets us to actionable insights, answers, and actions.
Dave Mariani: Yeah. I love that. Yeah. So, that sort of takes us into a little bit about your new venture, Naratav. And, and so why don’t we talk a little bit about the problems that you’re, you’re solving today as a co-founder and, and, and, and really an entrepreneur in this space
Brian Prascak: No, thanks, Dave. And I think, you know, just like your experience building, at scale and seeing the problems that exist in the marketplace and looking at where the opportunity is to help solve those with Naratav, it really starts with a simple premise, which is at the end of the day, what if our data could tell us what it knows so that we don’t have to figure it out. And if you think about it, we use data for one, really one primary purpose to answer questions, right. And how many of us know we might even know our data is And a lot of people are working on getting our data house in order, right. Cataloging our data, but how many people know what answers are available in their data
Dave Mariani: Well, or know how to ask the question I mean, for me, it’s like, I always thought it was really crazy that BI and business intelligence there’s really no, I in BI there’s no intelligence in BI it’s really, the human is supposed to be the intelligent person and the human has to figure out what questions to ask and what sequence to be able to get to something that’s actually useful. And I was just always thought that, that that’s just, that’s just the wrong approach, right Machines should be at least helping guide the user to what’s relevant, in areas where there’s something that’s interesting in the data and they should, the machines should be able to do that and help the customer or help the user to ask the right questions or at least to focus on the right areas. So is that what Naratav is doing Is that kinda what you’re thinking about
Brian Prascak: You nailed it. That’s exactly right. What Naratav is really about is creating what we call, autonomous insights, and automated data storytelling. So what it really starts with is you nailed the T the triad there, which is, it starts with the idea of thinking about data from an insights perspective and, and what is an insight and creating a taxonomy for insight. Again, the ID idea of a library has subjects of books, but we can have subjects of insights, which is really where we get action. And then we want to link them to exactly what you’re saying. We want to link each insight or the insights. We can see them and link them to questions and answers similar to what we do, kind of with search with Google, but to actually codify that in a way that we can actually now focus more on the actions we want to take, or the implications of the data, rather than trying to figure out what the data is telling us.
Dave Mariani: Yeah, that’s really, that’s really fascinating, you know, that was, you know, what we did at, at, with that scale is like, it was just the, the whole idea of the semantic layer is just really to lower that, so that, you know, today we’re asking, we’re asking our, our, our business users and our data scientists and application developers to, to really, understand data engineering, and not just understand how to use sequel, but also how to physically model data and understand all the constructs. And it’s getting more complicated because now you have nested data structures, you have semi-structured data and unstructured data, and we’re asking so much of, of, of users, that this doesn’t seem right. It’s like, you know, our whole goal is to, is to really, we, our whole goal should be to simplify it, and put that and put data in the form in the language that people understand. So I love, I love that too. You’re tackling that problem, and really, really, really going the next, taking that next step in terms of making data more usable and approachable.
Brian Prascak: Yeah. And I think David, the second piece of what you’re saying too, I think is that, you know, the idea here really for Naratav is like you said, to create that idea of an insights, taxonomy and linking it to questions and answers. So we can focus more on the actions, but what’s really cool is, and what’s missing from the, I guess maybe the I or the we in business intelligence is that this is intelligence isn’t personalized. So if we know what an insight is, and we know what those insights are, that taxonomy of insights, well, then we can know what insights are more important to one person versus the other today. Everyone kind of looks at the same reports that kind of look at the same dashboards. That’s not the way it should be. We all are seeking different. We all have different questions and seeking different answers.
Brian Prascak: And why can’t we have our business intelligence personalized to us, what that ends up doing is of course getting rid of all the noise of things that other people are interested in and really focusing on, you know, what those things are the important to me. And also, like you said, machines can help us to understand what we may not be asking for, but we might need to know based on our role or what other people look for. So that’s kind of the second piece. And the third piece is of course, because we have those insights codified within the organization. We can obviously optimize how we process them. Right. Reuse them and share them and really scale them.
Dave Mariani: Yeah, I love it. So, so, you know, there’s, you know, enterprises have a lot of challenges today, right. and, let’s just talk, switch gears for a minute and talk about the state of analytics, in the enterprise today. you know, I know you’ve, you don’t, you’re a practitioner. like I was, but, but you, you know, you’ve been, you’ve been at it more recently at Wawa. so w w what do you think analytics leaders should be focusing on at this, at this time
Brian Prascak: Yeah, I think where we are now, if you think about it as a maturity level, we have kind of, the original kind of the base level is disparate data, right People are really kind of at a point where they’re at, I call the second layer, which that level, which is, you know, centralized, integrated data, we’re moving into the rapid insights area, we’re then going to want to move to predictive prescriptive, which is where that simulation comes in. It’s interesting. The metaverse right. And then really the ultimate goal is continuous, autonomous. Everything we’re doing in the world is kind of becoming more continuous and precise, and that’s great because we can learn more, but we gotta be ready for that. Right. And so today I still feel like the technology is enabling us to, to move from that disparate, to centralized and begin to think about an action, the rapid side of it.
Brian Prascak: and it’s what it interests me and the opportunity to have had when I was at Wildwood to work, talk to you guys and ask scale about what, what can be done. So I think the PR where the problem is now is getting, we’re getting our data house in order where we’ve being able to use the modern data platforms. and we’re looking to how do we now deliver more rapid insights And I think the challenge is that, and we’ll talk about this today is in the data context of the data strategy is making sure that we, we have the data we need, we know how to harvest it, and importantly, scale it, because what ends up happening is companies are starting with some of these big use cases, and they can kind of scale the use case. But suddenly when you look at many use cases and many different uses of data, and then you have the operational side of data, which is the everyday insights, that’s not easy. And I think where companies are really struggling is how do I build that refinery of taking right that data that is in the centralized repository and making it available to users based on the combination of sources that they need.
Dave Mariani: Yeah. You talked about, you know, you talked about the data strategy M and a, and a lot of, a lot of, a lot of, of, of analytics leaders are, are, are, are thinking about that. And there’s a lot of different models out there. And like, so in your, in your, sort of, in your mind, in your opinion, you know, what is, what, what is a data strategy and, you know, and, and why do, why do organizations need a data strategy Okay.
Brian Prascak: Yeah. And so, David, I think traditionally when we think of transformation and of course data and analytics is transformation, just like we talk about digital transformation, we often talk about, and we should people process and technology. What’s interesting, again, is about what we use data for us to answer a question. And that question answering that questions can help us to take an action. And that’s the impact we’re looking to have. So the idea of a data strategy is really the tether, the idea of what the data can do for us to the impact it can have. And fundamentally, we’re asking kind of three questions, which is what are the questions and answers What are the questions we have And the answers we seek the second is, do we have the data we need and do we know how to harvest it And the third is, can we scale it So can we continue to deliver it in a way that’s effective and be able to refine it
Dave Mariani: Yeah. It’s like, that last piece, the can we scale it, we see a lot of customers who, had had the other two pieces. Right, right. And they made the investments. And, and then, you know, look, if, if, if you build a good product, people are going to use it. And so we see as a lot of customers who, you know, they, they move to the cloud and they open up that access. And then all of a sudden, they’re back with, dealing with scale, and, and, and dealing with the old problems of slow queries, you know, people not getting access to data fast enough. So, so how, how do organizations, Brian, how do they, how do they, how did they factor that in and how do they not run into the, the, the gotcha. When it comes to scaling out these operations
Brian Prascak: Yeah. I think, I think, first of all, I think the idea of, you know, what AtScale provides in terms of this idea of a semantic layer and kind of creating a way to define the data, virtually if you will, and also to make, be able to automate the process of actually translating the data into the features and for the consumption layer BI. So, so I think that some of the things that it’s really around automation, quite frankly, if we need to automate that process, and I think, fundamentally that’s where, you know, in Wawa other companies see the challenge is how do we automate that process was a lot of manual coding involved. Obviously when you have coding, you have maintenance. You know, if I want to add a new feature, I want to add a new dataset. You know, I need somebody to load that data and make it available.
Brian Prascak: So the process itself, really the process of kind of transforming the data to really, to insights and for consumption, you know, and we’ll talk today. It’s like a seven step process when you really think about it. And often we don’t, we, we do it, because different people do it, but I think that’s where the real headache is today is how can we make that process more automated How can we define data in a way that, that is, that is reusable That’s another piece of it. And then that’s, that’s important for governance, but it’s actually, how do I reuse the data without having to again, create another pipeline, which often happens because there’s not that awareness. So this idea of kind of automation, and this kind of extra call it virtualization or semantic where we can reuse it is incredibly important.
Dave Mariani: Yeah. Well, you mentioned, you mentioned, I love numbers. I love S steps. you mentioned that there’s this seven, seven steps or seven different things you can do. What, so let’s, let’s dive into that. So what are some of the things people should be thinking about, when they’re, when they’re thinking about their data strategy
Brian Prascak: Yeah. So, I mean, first of all, of course, you know, there’s, maybe there’s three areas really around the data strategy itself we talked about is, do I have the data Do I know what the questions and answers are that I have and linking those back to the use cases, obviously, do I have the data Do I know how to harvest it in third is I, I know how to scale it. The data strategy itself really is kind of, I said, seven there’s seven steps to actually transforming data, but there’s five components. I know I’m throwing out a lot of numbers. We love numbers, I like five, but there’s really five components to the data strategy. So that’s good. It’s not like right. There’s seven components to making the data actionable. We can talk a little bit about that. So it’s really are around, you know, direction and that’s our mission and emphasis.
Brian Prascak: The second is context, and this is super important. And this idea of linking questions to insights, to answers, to actions that discipline is super important. You know, what we want to do with Naratav is kind of codified that automatically, right? But effectively, we still need to, to know what we need to know. and it’s important to identify that, the third of course is about the data. Do we have the data we need? What condition is it in? And also we need to know a little more detail about what the subjects or the data are, what the structure of the data are? Features and dimensions are critically important because that’s how, how we get to insights. And again, today, we want to automate that more in the future. We want to automate that more, but fundamentally we need to link what we need to know with what the data can tell us, and we need to know how we want to structure it.
Brian Prascak: And then it becomes a matter of readiness. So is our data ready? What do we need to do to make it ready? And then finally we need to develop and deploy it. How are we going to continue to, how are we going to develop it, and deploy it in a, as you said, that’s where some of the that’s where finding some of the scale issues are. Because again, it’s not just one use case. It’s many, many, many use cases in many different uses, but those are the five elements of a data strategy. And having done this before, you know, it seems a bit abstract to say data strategy, because we talk about all kinds of different strategies and business, but it’s so critical to, to think about and really articulate, how are we going to use the data And it’s also very helpful for people that aren’t used to, they’re used to kind of, I want the answer, I want the data and really, they need to be part of that process to know how we’re going to take the data and refine it.
Dave Mariani: So Brian Mo so what’s the converse. What happens if you don’t have a data strategy What are some of the downsides there
Brian Prascak: Yeah, so the, the most common, the most common one is all the effort is spent, you know, to, to build a predictive model or, you know, to provide a set of insights. And those insights are usually with a predictive model and we don’t have the features, right. We, we, we’d find that, you know, the prediction, isn’t all that great because we’re missing some of the features that we need. And now we have to step back and look for a data source that might help us. Right. So not thinking about that upfront means that we, we, we don’t have the data we need. the second piece is somewhat around capability maturity. Some of it is around, what’s inside the data as well, but it’s, are we good at harvesting the data Right And, to make sure that the end of the day that the data is going to be actioned, right
Brian Prascak: So even if we deliver it, what are people going to do with it? And to really think hard about that. And sometimes it may be that the resource stuff, we need to do something about it, isn’t it, we haven’t thought about the business side of it as well. So it’s not just purely about data, but a big part of it is really around action. And then I think the third piece is, and I think what people are finding and what I found as well is we’ve done the first two pretty well. And that’s really good. It’s that scale part that’s really hard because again, that seven step process we haven’t got into a lab, but those, you know, everything from profiling the data to making it available for consumption and optimizing it for query like those aren’t trivial tasks. And they’re really hard to do when you’re doing them manually and you’re doing them across, you know, right. hundreds really we’ll try and to be hundreds of different users and use cases. So how
Dave Mariani: Should, so how so, how should analytics leaders deal with that problem then so how do you, how do you, how do you, how do you get ahead of the scaling issue?
Brian Prascak: I think a couple things, so one is we need to look at where the intersections of needs are across the data itself. So some of that has to do with the users and the uses, I think having a way, and that’s where the data strategy really helps. We can minimize the complexity by understanding where those relationship ships exist. So, you know, two different functions need that same set of data, the same set of features. I don’t want to create two different data pipelines. How can I create one? So some of it is just thinking through the needs and simplifying them based on right, how, how the organization needs it. So we don’t create extra work. The second area is developing a process like a refinery where people we know who’s going to do what to whom and how long it takes and creating the expectation with the business.
Brian Prascak: And then the third of course, is to me, Nirvana is automation. Every chance we get, we need to look for ways to automate that process, including self-service. I think self-service is ultimately the answer, governed self-service where the users can get at what they need. They can, you know, reuse the features if you will. And, and, you know, almost abstract the features from the data sources themselves, because that’s where some of the complexity exists as well. And that’s really, I think, you know, while we were, we were interested in talking about what AtScale provides, but that third layer, that layer of kind of automation and abstraction is super, super valuable, to really make, to ultimately make a difference.
Dave Mariani: You know, it’s like a, you talk about automation and, you know, there’s a lot of talk in with this whole concept of data ops and data ops is like, you know, it’s, it’s, it’s sort of analogous to dev ops where, you know, you have a continuous integration, continuous deployment, CIT CD kind of techniques that get applied to data, not just software, in applications. so have you seen, have you seen that Brian in, in use and have you in your, in your, in your experience, have, you’re starting to see more people, use those sort of data ops techniques to sort of deliver some of that automation and abstraction and consistency that, and scalability that, that, that we seek.
Brian Prascak: Yeah. We’re beginning to see that. I think we’re partly because we’re realizing where that problem really exists. It’s rearing its ugly head. And obviously we only have so many resources right. To do it. So automation in that area. So yeah. Data is code. I think we’re also seeing, you know, so much more use of the microservices kind of concept here. So I think it fits well. It depends on where company’s maturity is, but I think the more mature they get and the more they get into the rapid insights and they’re, you know, they want to really action their data, and many of them are already on the digital side using microservices. So the analogy I think fits really well there, and you’re exactly right. That’s I think Dave, where we’re going to end up. and so, you know, I think it’s really good to be thinking about that.
Dave Mariani: Yeah. I like, I think you’re right. It’s, that’s, that tends to be data ops is you’re, you’re pretty mature. You’re pretty far along the maturity curve when it comes to data and analytics. If you’re, if you’re using those techniques, you know what, I’ve also seen along with sort of, those mature customers who are using data ops they’re also, I also see them treating data as a product. I call it data as a product it’s like, in other words, it’s like, I’m the same way that you have a product manager who is working on the feature and the feature sets for an application. You have a product manager who’s working on, you know, the data features. and, and by doing a, and, and using that kind of discipline, it seems to, it seems to, to scale pretty well for these organizations, but again, they are, you know, you have to make the investment and you have to really change the mindset that, you know, it’s just not, data, just not something that is stored somewhere. And then people have to go and hunt and go get it. it’s really treating data as a first-class citizen. and as a first-class citizen, the same at the same level as the products that they produce and sell to their customers, it’s like data is, is, should be at the same level there. So it’s a real mindset change. and so, if you, you see that Brian happening and in some of the organizations that you talk to and work with, you see that.
Brian Prascak: Yes. So thanks Dave. When I was managing a data science team, I actually recommended that we hire data strategists and people were like, what and, and the whole point was that, you know, we, we do need to know how we’re using our data and a big part of that. I think what happens Dave is when organizations realize that they don’t have the data, they need a lot of time, especially with external data sources and that whole process of assessing that data and, you know, right. Making sure it’s right and integrating it, like that’s not trivial. So, you know, what tends to happen, I think is when organizations realize they don’t have the data they need, and they realize that that’s not just an isolated situation or the day-to-day have, isn’t quite, isn’t quite doing what it needs, because there’s more, that needs to be done to it from a, a feature and integration perspective.
Brian Prascak: And I think that’s the key too. The integrations that’s when I think you’re right, that this I’m a big believer. Absolutely. And having, thinking about it as a product, I think that is the way we need to think about it. And I think the way to do that is not only to think about, you know, who would be the product manager, if you will, the roles and organization. But I also believe collectively it’s really important to have a core team of data analysts and business owners be part of, kind of a, we had our own kind of council, if you will, where we were reviewing what we were doing with data, the new data sources, how w how we were impacting the organization. So I think it does fit in the idea of not only the product side of it, but also really the idea of organizing those, those keys, information, creators and consumers as a team to make sure that they have ownership for that transformation.
Dave Mariani: Yeah. I love that. and we’ve heard a lot about, so we have, in our, in our, webinar today where there’s a lot of talk from Tony’s different analytics leaders, about the importance of bringing the business and the technical teams together. and, we typically have been siloed in the enterprise, lots of, you know, walls and throwing things over the walls. And it sounds like, you know, you got the right idea about bringing those, those people together, and then the other sort of the other sort of topic, which is a tougher one, is, you know, how do you measure ROI and how do you, I mean, not just thinking about the word ROI, because that’s scary, but, so how do you know you’re, you’re, you’re improving. so have you, have you, have you done anything Brian, in your past, in your, in your organizations where you’ve been able, just to tell, like, you know, are we getting better at what we’re doing when it comes to delivering data to the business and making sure people are using it to make decisions
Brian Prascak: Yeah, I think, I think we can apply a discipline, like anything else, you know, it’s, it’s, there’s qualitative and quantitative aspects to it, but I think just understanding what those drivers are. so obviously, driving growth, driving margin, right Driving cost efficiency, capital avoidance. I mean, those are kind of the, the, you know, the typical financial side of it, but let me give me some examples. I think Dave, where I think they’re like, I call it the basic blocking and tackling, and I think a lot of organizations miss this, this is on the monitoring side, again, this idea of a system and right, and this idea of continuous feedback. So we’re able to, we were able to prove a lot of ROI, by basically creating mechanisms of measuring, productivity and performance errors, and so on or downtime, for example, which you would think, you know, some organizations may or may not be doing all that right now.
Brian Prascak: We have a lot of information from logs, et cetera, that I think we’re not utilizing. So one thing I would encourage folks to think about as we get involved with the big use cases, and we should write the big transformations, do not underestimate the power of helping somebody see when something’s not working so that they can fix it. And also whether or not it comes back. So I’ve done a lot of work. We’ve done a lot of work with just quick reporting on channel downtime, labor productivity, some, some manufacturing process stuff, that has actually paid in space. And it also broadens People can actually see the business and can actually say it’s hard. It’s right. When, when an executive sees that, you know, what’s happening, and whether or not it’s repeating people do something about it, or they’ll always say something like, if you measure it, you can improve it. So I would absolutely encourage that one way to build your use case. One way to build your value is not just on the big use cases. I think about the low-hanging fruit around everyday monitoring. Yeah.
Dave Mariani: Yeah. I like that monitoring is key. That’s a great one. you know, some of our, some of our leading customers, what do is, I mean, they, they measure, they measure everything cause it, with a semantic layer, since the, all the queries are coming through it, it’s pretty easy to manage, to, measure how many queries per day, how many, what’s, what’s the speed of those queries and how many users are, are, are using and, and generating queries and from which tools. And so with that, you know, you should see up into the right, in terms of curves, right. Should see more people, who are asking more questions and getting more value, and also getting, and getting, as good or better performance with, you know, the more questions that they ask. I know those aren’t really sort of crude measuring sticks, but you’ve got to start somewhere. and so monitoring is, in, monitoring that activity is I think, a good place for, for companies to start at least. So I really liked what you said there,
Brian Prascak: And I would just add two other areas, I think, cause I think we want to make sure we kind of get the gamut of, you know, not just a monitoring, but I think even in the, what we call our basic business intelligence, it’s really making sure that the scorecards we create are really actionable. and particularly around the idea of what’s changing. So, you know, we’re really looking for what’s changing and when we need to change, obviously with COVID, that’s a great example where right. We obviously had things changing. We knew we needed to know. So the importance of our traditional business insights was really lifted. And then the other piece I would say is certainly around predictive analytics. my experience is sometimes, you know, we were predicting when a customer would pay. and we were getting like 93% accuracy in the model.
Brian Prascak: the tech, the algorithms today were amazing random forests and so on and so forth are phenomenal. they do need to be trained and retrained, refresh and retrain quite often. But, you get that kind of accuracy. That’s amazing. But what I would find with the business, sometimes it took them a while to trust the model. Right. And that’s okay. I say to them, don’t do anything just because it’s going to happen. Right. It’s a system that’s okay. We’ll, we’ll predict it again, you know, next month and we’ll tell you what happened and we’ll tell you how well we did. It’s okay. And it, you know, it, it, it took three or four months and then it was like, Darren, we, you know, let’s go, we need to do something about this. Right. And then that’s when the fun process starts because they start to really understand how the system’s performing. And that’s the one thing I would encourage everyone is, you know, it’s kind of a higher level way of thinking, but think about your business as a system and you have this monitoring, you have this knowing when to change and you have, what’s the future, the near future going to look like, and that’s provided that that’s the way to really think about how to get the full impact, but you can start in any one of those three areas.
Brian Prascak: So that’s a really, that’s a really interesting use case. So how did you get the business to trust the prediction of when people will pay Because obviously you wanted them to do something once they know and have a prediction of when people will pay you, you know, you don’t want to do something about that to improve it or make it better or, or take some other action to make the, make it better for the business. But, so how did you, how did you drive that trust? And it took, you said it took them four months or something like that. So how, how, how, w what’d you do to drive trust
Brian Prascak: Yeah, so, well, first of all, we worked for them obviously on where we’re gonna apply it and you know, how we set up the POC. but what ends up happening is we created a business intelligence dashboard. We could show them every, you know, every month what we were predicting, but we were also saying, what if we changed? So we started with the obvious there’s customers that aren’t paying us at. All right. And that’s low hanging fruit. That’s, I mean, it’s hard to walk away from that. So if every month we’re telling you that that customer is not going to pay and we’re 93% accurate, that’s, you know, what, if the 7% you kind of still don’t ship them a lot of product, you know, you’re not really going to upset them. Probably there may be some risk there, but that’s a pretty big one that’s, you know, that’s a pretty high accuracy for avoiding right losing money.
Brian Prascak: That we kind of, we kind of picked on the spots where to look at it. This is low-hanging everything right. I believe in the parade of principle, it’s impossible to avoid, right The 80, 20 rule, there’s stuff at the top of that curve, start at the top of the curve, look for the low-hanging fruit and, and inevitably anomalies are part of that. And inevitably there’s something that’s contributing. So we think, again, the 80, 20, 20% of the customers, 80% of the late payments, if that’s, what’s going to happen. So think strategically those patterns are, are really present. They’re always gonna be there. So drop that pattern of contribution and then focus on a couple of the things that are, I would call obvious to the business where, oh, they’re like, oh yeah. And, and then begin to build trust by having them actually fix it.
Brian Prascak: Right. And what they ended up finding was that there were policies that weren’t necessarily codified and thought well thought through, around when, when they were being asked, the customers were being asked to pay and what time of the month it was being paid. And there was also things around, oh, wow. There’s people who thought that maybe it was around customer service, that they weren’t paying that, you know, the products were being delivered late. It was actually economic. Right. so we went through that whole process. And if it’s economic, then can we get smarter about understanding the changes in economic conditions Of course we can. So it began to have a really rich, really rich conversation. But to your point, we started with this idea of the 80 20 rule, find those things that are quite obvious and impactful and build credibility from there.
Dave Mariani: Yeah. I love it. It’s like, too many people, including myself, can try to boil the ocean and you don’t get anywhere and you spend a lot of money and there’s this frustration all around. I love, I love this picking, you’re picking your battles and proving, proving the value of those investments. I mean, that’s very, that’s a really obvious value. So, so, so Brian, so, you know, so what, what are, you know, what are some of the other things, what should be just to sort of like maybe wrap this session up It’s like analytics leaders are out there. There’s a lot of things that they can do. so, you know, what would you recommend to an analytics leader, you know, to how, where, where should they spend their time and implementing an effective data strategy
Brian Prascak: Yeah. So number one is obviously make sure we know what, what are the core needs of the business from a, from a change perspective, B be tethered to those changes, make sure people know, the role that data and analytics plays. You said, pick your battles, make sure that you pick something that’s gonna make a difference financially that, you know, you can get started with, if you’re just starting the journey. And then I would say, be really thinking about how you’re going to scale, you should expect to be successful, right. and when you get to that point, and that’s kind of where I was getting my experience is finding that we’re having, you know, that obviously as scale knows this, you know, that you start to become successful and you’re getting that momentum. You’re having a lot of people knocking on the door, which is what you want. You want to build that in. And absolutely. So think about, think about this idea of automation and think about the process, think about how you can simplify it and automate it and optimize it. I would say those three words for any leader, you know, you’re going to get started, you’re going to be successful. Proving it. You do, you have to do that, but in the back of your mind, simplify, automate, optimize will make all the difference. Okay.
Dave Mariani: I love that. That’s very well said. So, Brian, thanks. Thanks again for joining us here. and you got some, some, some, some great experience and insights for everyone out there to be a better analytics leader and, be better and more data-driven. So thanks a lot for joining, joining me today. And, everybody in the audience out there have a great day and to you, Brian, thanks so much.
Brian Prascak: Thanks, Dave. Thanks again.