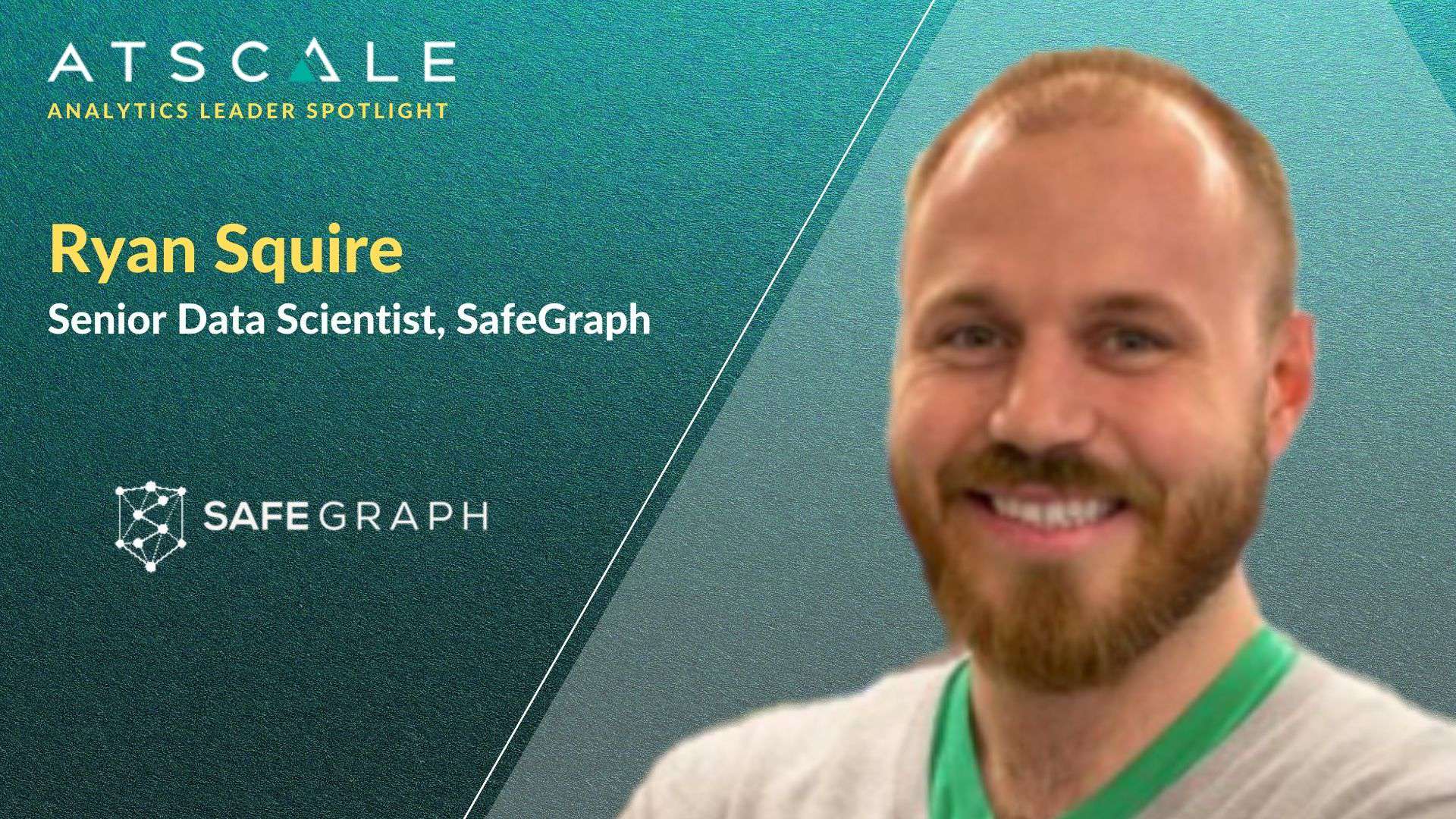
Welcome back to our “Analytics Leader Spotlight” series, where we get to share the stories of the people who are transforming their organizations and others with the power of data analytics. In today’s spotlight, Cort Johnson, VP of Growth at AtScale, interviews Ryan Squire, Senior Data Scientist of SafeGraph.
Ryan, we love sharing origin stories of how people have built their careers in data and analytics. Would you be able to give us a quick introduction about yourself and share how you got started in the space?
A: Absolutely. I have a background in science. I’ve always loved science and studied neuroscience at Oberlin college and then went to do a PhD in neuroscience at Stanford. When I was doing my PhD, there’s lots of things about science that I really love. I like working with data. I like designing experiments. I like communicating about results. But there’s some parts of science that I just didn’t love as much as others. And particularly didn’t love being in the lab, doing experiments all day. As I was trying to figure out what I wanted to do in my future, I had friends of friends that worked at tech companies and worked in this thing called data science. And, it sounded like maybe something that I could be excited about.
And so, just sort of serendipitously, I was able to join a startup after I left school. And, I think my career has mostly been focused on working at early stage startups. I think startups are a great way to get into data science and get into tech because there’s just so much opportunity for professional and personal growth. You get to try so many different things and learn so many different aspects of the business.
I worked at a company called Lumosity, which is a brain training game company, sort of a neuroscience company. That was a great experience and I got to learn a lot from my teammates. And then for the last four years plus, I’ve been working at this company SafeGraph. SafeGraph has been particularly a fun experience because it’s very data focused. SafeGraph as a geospatial data company, we sell data sets and our customers are data scientists and data analysts. It’s a really fun place to be a data science person. I’ve learned a lot and grown a lot there.
When you talk about getting started in science and going to school to learn more about that particular field, was there a subset of the science field that you got into first? Was it computer focused? Was it biology focused? Chemistry focused?
A: Yeah, I sort of came into it through biology but I rapidly got most interested in sort of neurobiology and neuroscience. I think part of the reason I was so interested in that was because the brain is this super powerful, computation machine. The brain is able to do these amazing things and I was just super interested in how that worked. In particular in grad school, I was focused on understanding vision, how does the brain process visual information and visual attention. So, how does the brain decide what parts of your visual field to pay attention to and what parts to ignore. I think the more I got into that, the more computational and data intensive that got, studying those problems exposed me to a lot of computer science and exposed me to a lot of high-dimensional data analytics problems. I think that also helped get me more into the tech and data mindset.
Going into neurobiology, how much do you rely on your ability to do analysis using computers versus doing lab based experiments?
A: The heart of all of science is collecting good data. I think one of the biggest differences between being a data scientist in tech and being a real scientist, a big part of the job is just the collecting of the data. In data science, one of the things that’s so interesting about working in data science is that usually you’re not the one collecting the data. Maybe your company is collecting that data for whatever reason, or you’re getting that data from some public data set or you’re buying that data. You get to just worry about what to do with the data. In science, a large part of the job and a lot of the innovation comes from the ability to collect the data. In some ways that’s a very different sort of skill set and different mindset.
Absolutely. It’s amazing just to hear some of that crossover between what we tend to see in the tech field, being more around statistics and computer science and in necessity to sometimes have those skills in order to be successful in the other aspects of the sciences.
A: Yeah. I think there certainly is a lot of crossover just in sort of data literacy. You want to understand, if you collect some data about something and estimate something, you want to be able to understand how confident are you in that estimate and what is your uncertainty about that estimate. These sorts of fundamentals of statistics I think are certainly important, both in academic science and data science in tech. I think there’s certainly a lot of mindset, thinking and statistical skills that overlap, but certainly lots of different skill sets too.
Let’s hear more from you about the data science work that you’re doing today. You let us know a little bit about the company you’re at now called SafeGraph. Why don’t you share with us what a “day in the life” looks like for you at SafeGraph and what are some of the daily responsibilities.
A: I’ve been at SafeGraph for over four years and during that time we went from three people to now we’re around 40 people. I think in many ways, I’m a startup employee first and a data scientist second when you’re in an environment like that. My role has certainly changed a lot over those years, but as we’ve gotten bigger and as the company has matured, I’ve been able to focus more and more on core data science work. For example, today, a lot of my work is very project oriented. Week to week, I’ll be working on different projects that are either internal data analysis projects, like product or engineering has some questions about our product or about our data sources that we’re trying to answer. Or it will be externally focused, as I mentioned, SafeGraph is a data company. Our customers are often data scientists or data analysts. We don’t build software analytic programs. All we give them is data, that’s our product. We just sell them CSVs. There’s sometimes a gap between what they are trying to do and what we give them. A lot of my work is an education around what can you use the data for, what are the quirks of the data, what should I know if I’m getting started with the data? We try to help bridge that gap by creating guides and tutorials and demos that might be some sort of example of an analysis question you could answer using SafeGraph data. The ultimate deliverable is either some sort of published notebook or blog posts that our customers and prospects can consume.
As a data company, that is the IP that you guys are selling. So the more you can do to educate the audience on what they’ll be able to accomplish with that data can be extremely valuable. So it sounds like a lot of the work that you get to do is to be able to enable prospects and customers to understand some of the outcomes that they’ll be able to drive using your data. Is that a good summary of the work that you get to do?
A: Totally. What’s fun about that is that I get to try to dive into these customer use cases, understand what really is the problem they’re trying to solve. And, often we have similar types of customers trying to solve similar types of problems, and so we can build sort of centralized educational resources or guides that will hopefully help lots of people. It’s fun to prototype those things. And it also makes sure that we’re really close to our own data and understanding our own data so that if there’s problems, we can try to find those.
You bring up an interesting point. Through the course of doing these conversations with other folks in the data and analytics space, we’ve been able to talk to a lot of people who are identifying the problems internally at their company and trying to solve them. I think you get to sit in an interesting spot, similar to us at AtScale, where you talk to a bunch of prospects who come to you with that challenge or problem and try to understand how you can help them solve it. I’d be curious in your role, in talking to the prospects from the SafeGraph standpoint, what tend to be the couple of large challenges that you see your prospects facing that you’re trying to help them overcome?
A: I can give you a little bit more context about what SafeGraph data is to help contextualize that problem. Since SafeGraph is a geospatial data company, in particular, we’re focused on understanding places and points of interest. Places that you might put on a map, places that consumers would visit like businesses or coffee shops, restaurants, malls, hotels, etc.
Our customers come in different types of buckets. There’s lots of use cases in retail and real estate, financial services, and marketing and ad technology. But all those use cases have some common problems. A lot of them have to do with things like data interoperability and how I work with the data. One of the sort of common problems that we see often is that all of these businesses and areas that we’re talking about care about places for different reasons and in different ways. In many cases, these customers already have data about places in some form or another. That could be first party data that they have about their own businesses. That could be data that they’ve gotten from some other source. Ultimately you want to try to combine these data sets together to answer their questions.
But that’s very non-trivial. When you have a data set from SafeGraph about places and your own data set about places, trying to combine those can be very hard because there is not a common key or a common index for places to join them together. So that’s a common problem that we see. In response to that problem, SafeGraph has been a founding contributor to this new initiative, this open standard called “Placekey”, which is trying to solve that problem. It answers the question,“How can I take places data from different sources and make it easier to join them together?”
There’s lots of common problems around how people work with data, whether that’s combining data together, whether that’s just getting data into my hands, can I work with it? In Excel do I need to use Python? Do I need to use Tableau? What tools am I using? As a data company, we’re always trying to figure out what are the things we need to do about our data sets to make it as easy as possible for our customers to use them in whatever tool they prefer. But, depending on what that tool is, that can be easy or hard for what they’re trying to do.
You bring up a good point about first party and third party data. We’ve had conversations about this, we’ve done a webinar together about this. Maybe speak to why companies will look to third party data sets to enrich their data for analysis and what are some of those hurdles that people may be facing now where they’re not sure how to get to overcome them where maybe a third party data set or multiple third party data sets could be the answer to answering questions, in order for them to make a better business decision.
A: Imagine you’re a retailer. Maybe you’re a quick-service food restaurant and you have hundreds or thousands of locations across the country and you have very good data about those businesses. So, you’re tracking point of sale data. You’re tracking supply costs. How much beef and chicken are we ordering from week to week, month to month. If you’re doing a good job that data is being collected consistently and is being made available in a way to your analysts into your team so that it can be used. Even that is not accomplished by every company today. So just in terms of using your own first party data, I think lots of companies are trying to figure out the right way to do that, how to make sure that data is collected consistently, how to make sure that there aren’t gaps.
In some cases maybe there are gaps for whatever reason, maybe some of your restaurants use one point of sales system that collects data. And another set of restaurants doesn’t use that system. And so you don’t have data for every location. That’s one case where a third party could be valuable because it could help fill in gaps in your first party data.If you only have first party data on some subset of your locations or vendors or things like that, going to a third party dataset could potentially be helpful.
I think another reason that third party data can be valuable is that let’s say you’re doing a great job with your first party data. So you have consistency across all locations, consistency across all vendors, you have a great ETL, internal data platforms, your analysts can get the data they need when they want it. At the end of the day, you’re still only gonna have data about your universe, about your stores. You’re not going to have data about the competitor across the street. Obviously having data about your competitors or your compliment businesses in your neighborhoods can be very valuable.
Even in sort of a best case scenario, “I’m doing everything perfect with my first party data”, you can still expand your understanding of your local universe by looking to third parties, to get data about your compliments, your competitors, or just other macroeconomic trends happening in those regions. So, those are just some examples of how you can do great stuff with first party data, but you can also always augment and expand with other datasets.
That’s a great point. The interesting part about the overall ecosystem today is that it feels like it’s third party data, whether they’re public data sets or private data sets like SafeGraph are more readily available for folks to be able to incorporate. Obviously you guys provide a very well documented and structured data set, which makes it really valuable to your prospects. To switch gears, I’d be interested, as you think back on your career so far you’ve obviously worked at various places. You’ve done a lot from an education perspective when you evaluate the different things that you’ve worked on. What’s maybe one or two projects that you’re super proud of or super excited to talk about?
A: I’m really proud of a lot of the work that we’ve done at SafeGraph over the years. One of the things that’s interesting about SafeGraph in general is that we’ve taken a very hard stance to “just be a data company”. If you think about your data analytics value chain, there’s lots of different components of that value chain. One important, but relatively small component is the data itself. That’s important. But you also have the tools you’re using to work with that data, the technologies, the analytic questions, the analytics solutions, visualizations; there’s a whole long chain and SafeGraph has decided to really just focus on just this one little part of the chain, which is we’re going to try to be really, really good at the data, but we’re not gonna build an analytic solutions. We’re not going to build analytic software.
I think that’s been a really fun challenge to do because it really makes us laser focused on what it means to be really, truly great at just data. There’s a lot of benefits you get from choosing to try to focus on only a few things. More recently I can talk about this project that I’ve been involved in, which is this project called “Placekey”. I mentioned it a little bit earlier. Placekey is an open standard. It’s an initiative across many organizations. SafeGraph is one of thousands of organizations, contributing to Placekey. But SafeGraph is a major contributor and using our data to help power some of the Placekey API., I won’t go all the way into Placekey, but the short version is that Placekey is a common universal identifier for all the places in the world.
There’s been a lot of different efforts contributing to that. We had a big launch event recently. I gave a small talk at that event, sort of a 10 minute introduction to what Placekey is. If you’re curious, I would check that out on placekey.io/seminars. I’m super proud of what the Placekey the initiative has built. It’s really impressive. It’s really useful. I’ve had a very small contribution to that. So I’m proud of my little humble contribution, but overall I think that’s a really cool project. It’s free, it’s open. No one is profiting off of Placekey, it’s an open standard for the community. I think it should be really valuable and helpful for people.
When you launched that I was reading more about it. It sounds like it’s going to be a great opportunity for all folks who are doing location base or point of interest based data, just to make it easier to do that type of analysis. Kudos to you and the team on that! When you’re staying up on the space and trying to just keep abreast of what’s going on and any other interesting initiatives that are happening within the data analytics ecosystem, where do you tend to go to get that insight or educational material? Are there any specific blogs or people that you follow, or just publications that you read?
A: There’s so many amazing resources available to learn and I’m not sure I necessarily have optimized that for myself. I follow a lot of data scientists on Quora and Twitter. I’m a big Quora user. I really like Quora. Especially early in my career, I found Quora to be a really useful place to sometimes learn technical information, but often to learn what I would call more “meta knowledge” about data science. Like, how do people think about the problems? How do they talk about the problems? What are the topics and concepts that I should try to learn about? I still think that Quora is a great place to learn about data science.
I find Twitter to be a little bit lower signal to noise, probably. There’s the occasional good nugget, but I can give a stronger recommendation for Quora.The other thing I would say is that I can’t even count how many times I’ve read articles on “Towards Data Science” blog. They’ve created an insane library of content. It’s not a blog that I read every post, but I can’t tell you how often my Google searches end in a “Towards Data Science” article. I’ve learned a lot from those posts. The last thing I could say is that SafeGraph is really a geospatial oriented data company. I was sort of new to geospatial coming into it four years ago. There’s a podcast called “The MapScaping Podcast”, which is sort of a general geospatial interest podcast that I really have enjoyed listening to and learned from.
What advice would you have for others who are getting started and maybe folks who are like you, who are maybe pursuing a specific field of study and are maybe interested in data science and analytics as a whole, or just data in general. What advice might you have for them as they pursue their career in data and analytics?
A: Here’s what I would recommend as the top-level takeaway. There’s so much to learn in data science. Data analytics and data science is a huge umbrella of topics and there’s sort of an overwhelming amount of topics to learn under that umbrella. You’re never gonna know everything. With data science and data analytics, it’s a commitment to a life of learning and you’re going to be learning your whole career. If we accept that as true, then I think some advice follows from that, which is that, try to just try to start working as soon as possible. I think there’s a temptation to study more, learn more. I’m still learning to become a data scientist, but in reality, if I’m in the right job where you can do some sort of data work, an analyst job, or some sort of data science job, that’s going to be a huge platform for learning for you and you’re going to learn as much on the job as you would trying to study at home.
The strategy there really is finding a job that will give you opportunities to grow and learn and finding a job where you’re not the expert at the company. Especially if you’re getting started, you don’t want to join a company where you are the person that knows the most about statistics at that company, because you’re not going to learn as much as if you work at a company where you had a team of people who you could learn from. Ultimately, we should think of work as a platform for self-development. So that means that you should prioritize finding jobs that you can learn and grow in. Don’t let the feeling that you don’t know everything hold you back from getting started because no one knows everything and I’m still learning and we’re all still learning. It doesn’t mean that you can’t create value today for your company and also keep learning while you’re doing that. That’s where there’s lots of fun to be had. So, I encourage people to try to get that first job and get into it and keep learning the whole time.
Thank you Ryan for joining us! If you’re interested in hearing more of these conversations through CHATSCALE, you can visit atscale.com or find us on SoundCloud. If you would like to hear more from Ryan, you can also find him on Twitter, @RyanFoxSquire.
Want to hear from more analytics leaders? Check out our past interviews:
- Jen Stirrup, CEO, Data Relish
- Daniel DeFuso, Lead Healthcare Business Intelligence Analyst, Populytics
- Aamedh Bhargava, Senior Analyst Supply Chain, Home Depot
SHARE
Guide: How to Choose a Semantic Layer