March 5, 2019
What’s the best BI tool for Hadoop?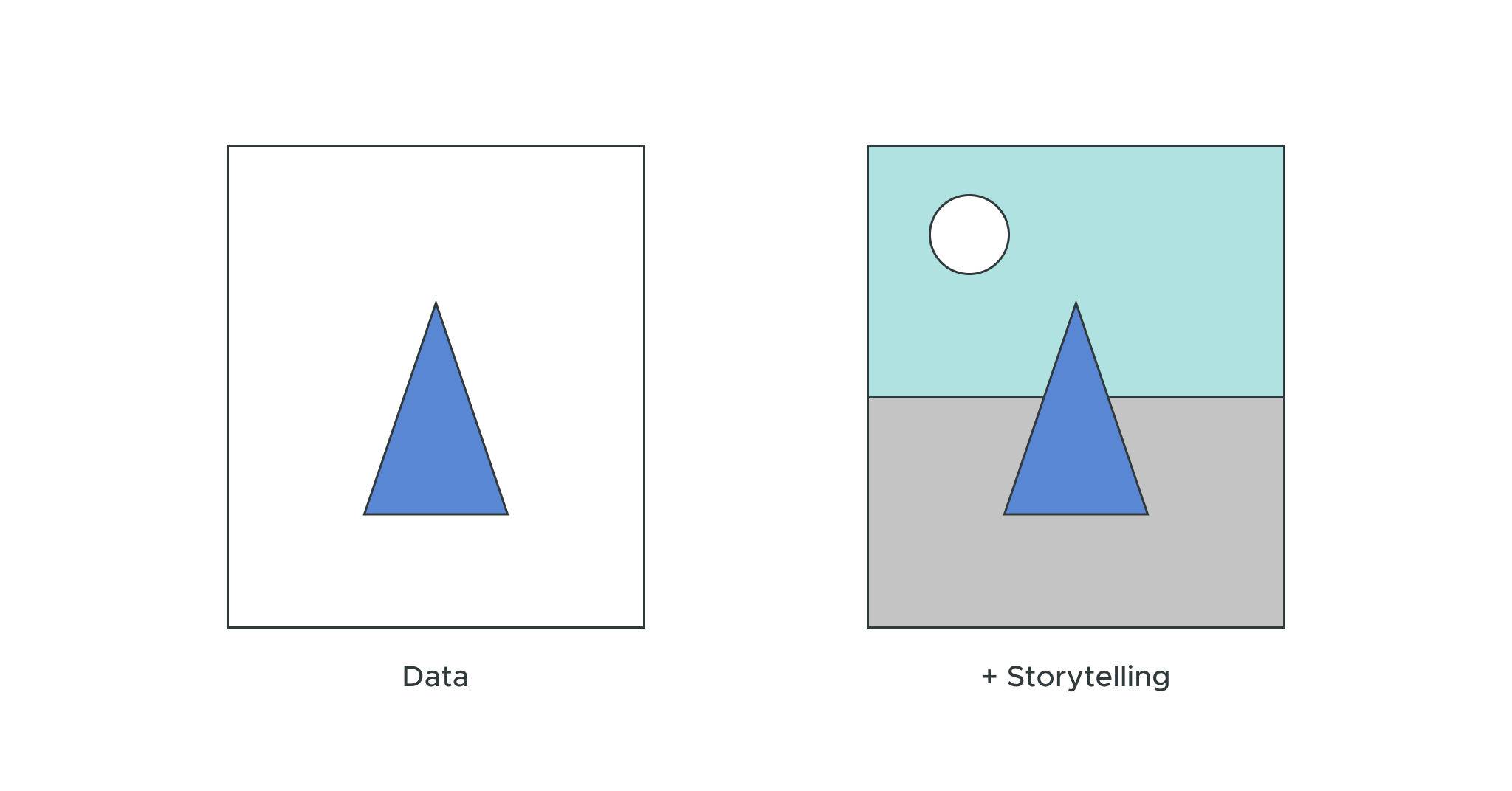
Effective data storytelling is integral to ensuring your organization leverages data insights. But how can you tell a meaningful data story that influences action?
An analytics-driven culture doesn’t crop up overnight — it’s built through effective data storytelling.
Implementing a new AI project or data model isn’t enough to motivate entire teams or organizations to actually use and derive value from data insights and analytics. However, framing data within a resonant narrative arc — with characters, a setting, a conflict, and a resolution — can inspire teams to make the most out of analytics applications.
In this post, we’ll outline why data storytelling is important to building an analytics-driven culture and run through the four essential elements of any compelling data story.
What is Data Storytelling?
Any organization can adopt a new technology or implement a new data model that generates what could become an “actionable insight.” While algorithms create and posit data insights, humans need to take those insights and actually apply them to the appropriate use case to create measurable business impact.
According to Harvard Business School, data storytelling is “the ability to effectively communicate insights from a dataset using narratives and visualizations.” It’s the way in which we argue with data to support a larger narrative and convey a message.
Data storytelling is the means through which businesses illuminate the potential value of data insights and demonstrate why it’s worthwhile to learn a new application or make workflow adjustments to achieve that value.
The Importance of Crafting a Good Data Story
Most organizations have no shortage of data analytics capabilities underlying every function of their business. But few are actually using these data insights to their full potential. That’s because taking data insights and analytics across the finish line takes work — people need to extract these data insights, apply them to a defined use case, and take the right action to create business value.
This necessitates some changes to people’s daily workflows. And behavior change can be difficult.
People aren’t moved by numbers or hard facts — they’re moved by emotions. Data storytelling takes the raw numbers and percentages provided by data and elevates them through an emotionally-focused, persuasive argument that is more likely to inspire action than a singular figure on a dashboard.
Without a compelling data story, the time and money invested in various data models or AI implementations go to waste. Data storytelling may seem like a peripheral soft skill in theory, but in practice, it’s a necessary tool for achieving business impact.
4 Important Elements of Data Storytelling
There are four elements to every good data story that data analysts or business leaders need to include to inspire action on the part of their teams. These elements of data storytelling are also the four components of any meaningful narrative arc: character, setting, conflict, and resolution.
1. Characters: Who Are the Stakeholders?
The first element analysts and business leaders need to consider is character: who does this data affect? Who should the audience relate to? Every good story centers on a character the audience can see themselves in.
Build a character profile that mirrors your audience. If you’re speaking to engineers, the data story should revolve around someone developing an app or software solution with the same KPIs as your audience. Make it clear how and why the data insights will impact their role, their performance, or their project.
This impact needs to be significant, or it won’t be moving. Fill out the details of the character and their goal thoroughly enough that the audience can see the big picture. Talk about what’s important to this character, why they’re invested in the project they’re working on, and how success or failure would make them feel. This emotional portrait is the engine that will drive your story forward.
2. Setting: What is the context?
Next, you need to ground your audience in a specific time, place, and environment. Explain why it’s important that your protagonists achieve their goals at this time and in this situation. Identify the inflection point that elevates this scenario to one of significance.
Outline a before, during, and after to answer the following questions:
- What happened before implementing this data model?
- Why did the character start using the analytics capabilities they’re using now?
- What will happen if this character is able to effectively leverage these data insights?
- What will happen if they don’t?
Again, it’s important to ensure the setting and context of this story are relatable to your audience. They should optimally be working at an organization similar to that of your character, during a time that is typically stressful or significant for the business, working on a project that carries some weight in relation to the character or the organization’s KPIs.
3. Conflict: What is the Problem?
Within this context, you need to create a point of tension. This serves as the conflict, or problem, of your story.
The stakes need to be high enough that the audience feels some kind of emotional investment in this problem being solved. At the same time, the conflict needs to be one that either has happened or could happen in the work lives of your own audience. Otherwise, the story won’t resonate with listeners.
This conflict needs to have only one pertinent solution: the data insights generated from the AI project or data model your team uses. Solving this problem through the application of data insights must result in some measurable reward for the characters.
4. Resolution: What is the Proposed Solution?
Finally, neatly wrap up your story with a satisfying ending. This is where you should prominently feature data insights as the solution to the protagonists’ problem.
The solution to the problem must feel inevitable and meaningful. Outline how the characters solved the problem in detail, what occurred as a result of this problem being solved, and what the entire organization gained as a result of this solution.
This part of your data story should include a reference to the bigger picture. If your audience zooms out, do they see larger value in solving this problem through data, either on a personal or professional level?
It’s also important to discuss what the audience can learn from this story. Talk about how leveraging data insights could provide similar value moving forward, and how the organization can measure the success of their efforts to integrate actionable data insights into their workflow.
This will make it clear to your audience what they should do with data insights, how leveraging data insights will impact their professional goals, and how it will impact the business at large.
Ultimately, a compelling data story helps to increase the data literacy of your organization, or their ability to understand and apply data to create business value. This will ensure any AI implementations or new data models yield measurable ROI.
The Roadblocks to Creating Data Stories
Crafting a narrative is only one piece of the puzzle when it comes to telling a compelling data story. The other challenges revolve around actually extracting meaningful data insights from data models or analytics systems.
- Getting the right data from disparate systems: Most organizations are using several data sources and analytics applications to glean data insights. While having a plethora of sources ensures teams have access to more granular, comprehensive data insights, leveraging disparate systems can also create additional complexity. Manually uniting data from disparate sources into a single, holistic view creates additional work across teams, which can deter people from taking data insights across the finish line.
- Duplication of effort when computing business metrics: In addition to complicating workflows, disparate systems can also lead to duplicative work for your teams. This work is especially infuriating since it necessitates redundant effort without any measurable added value. Limiting duplicative effort is key to motivating teams to leverage analytics platforms and data models and reducing the likelihood of burnout.
- Inconsistency of insights from siloed data sources: Because organizations so often use a variety of systems and data models, they also frequently receive conflicting information from different sources. These inconsistent insights can be misleading or confusing for the end user. Not having access to a single source of truth across platforms reduces certainty across teams about which insights to rely on.
Data Storytelling with AtScale’s Semantic Layer
AtScale’s semantic layer ensures businesses get consistent insights across disparate data sources and systems, eliminating complexity and confusion. This single, unified view of an organization’s data, analytics, and insights eliminates duplicative effort and ensures accuracy through a centralized governance model.
The semantic layer offers self-service capabilities that — when combined with sufficient data literacy — can help organizations craft compelling data stories that drive business impact.
Learn how to tell better data stories with a semantic layer.
See AtScale in action. Book a demo today.
NEW BOOK