April 7, 2023
Using a Semantic Layer to Replace SSAS and SSAS Cubes![How to Liberate Your Data [Scientists] with a Semantic Layer](https://www.atscale.com/wp-content/uploads/2022/02/04_LiberateData_light.jpg)
Every business decision, keystroke, or mouse click is a potentially valuable data point. Most companies don’t have any trouble generating and storing their data these days, and there are mature technologies that help companies break down silos to get all their data functionally in the same location.
These advances were significant, but there remains a critical silo that needs to be broken down for companies to get the most out of their data and data science teams. Descriptive analytics teams and technologies are still kept entirely separate from their counterparts leveraging machine learning-based analytics.
The gap between descriptive and ML-based analytics can have significant business impacts. With either side presenting an incomplete picture, companies may make the wrong decisions. Potentially even more impactful, data scientists may need to spend significant time reconciling analyses between the two models that don’t match up. One solution to break down this silo and create cohesion between descriptive analytics and ML-based approaches is to use a semantic layer.
What is a Semantic Layer?
The value of the semantic layer goes beyond consistent views and language. Customers can define business metrics once and reuse them across any toolset, anytime. This feature allows critical metrics like KPIs to be consistent across workstreams and ensures data science teams traverse dimensions (like customers and time) in the same way. Automating the discovery process provides a level of cohesion that is nearly impossible to match by having individual teams come in and define these metrics manually.
The semantic layer works with the underlying elastic platforms where data is stored and leverages powerful AI to optimize query performance and cloud resource utilization. Taking care of these complicated tasks liberates data scientists, focusing their work on delivering business-driven value to the company.
Bringing Structure to Data Science Programs: Semantic Model vs. ML Models
ML models are primarily based on relations between features and output. Conversely, the semantic model is all about the relationships between the data and the business metrics definition. The idea is that, regardless of what tools teams use, if they are analyzing the same metrics, the analysis results should be consistent. The semantic layer emphasizes speed, consistency, and reusability. One of the main ways this is achieved is through conforming dimensions, which allows near-instantaneous integration of valuable third-party data sets and provides consistent results regardless of the underlying toolsets used beneath or above the semantic layer.
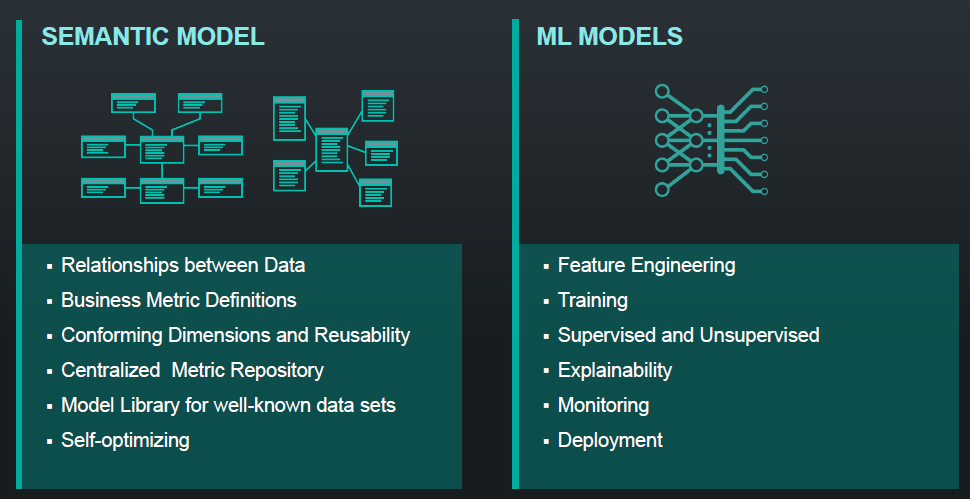
BI vs. ML Style of Analysis
For data science to empower business decision-making, the information and insights mined from ML methodologies need to feed seamlessly into BI analysis processes. BI style analysis is very formal, while ML style analysis is adhoc and exploratory. The semantic layer excels at breaking down the silo between BI and ML analysis styles. Data scientists can benefit from having business vetted data, logic, and calculations as part of their analysis tool bag, to ensure their machine learning models are aligned to a business need and outcome.
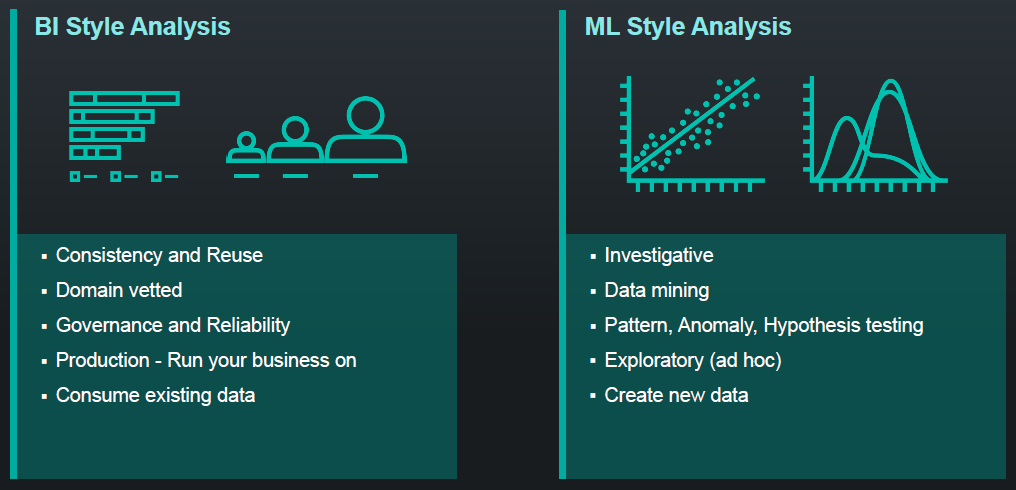
The Data and Analytics Flywheel
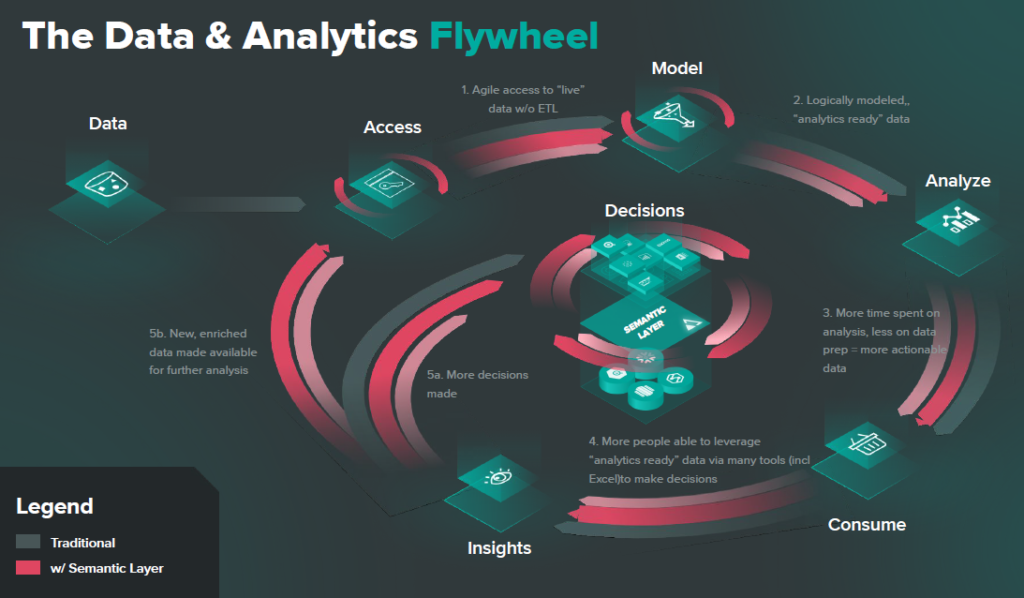
Data science teams can only work together effectively when everyone speaks the same language, which means using the same semantics. With a semantic layer like AtScale, complex problems like data access and data modeling become easy because individual teams don’t need to remodel the same issues and metrics in each of the underlying tools serving their business side. Furthermore, feature creation becomes streamlined as data scientists have access to the metric store their BI counterparts have built as part of their business reporting workflows. They can log in with the tool of their choice and drag and drop their dimensionality and KPIs with no additional coding.
Once predictive and descriptive analytics is working in tandem, discoveries and outputs made by either side can be modeled back into the semantic layer to improve both capabilities and create one central view of all relevant information. And once data science teams are spending more time on analysis and less time on prepping data and models, they will start to generate actionable insights for the business at a much higher rate. This will naturally improve the business’s decision-making velocity as a whole.
The Semantic Layer Transforms Raw Data Into a Structured Analysis Feedback Loop
With a semantic layer in place, it doesn’t matter where our raw data is stored or what underlying tools different teams in the business use to consume information. Everyone consumes from the semantic layer, and data science discoveries are written back into the semantic model to inform future analyses. Data science becomes platform and tool agnostic, and costly rework is eliminated, liberating data scientists to focus on analysis and ensuring consistency across models and metrics.
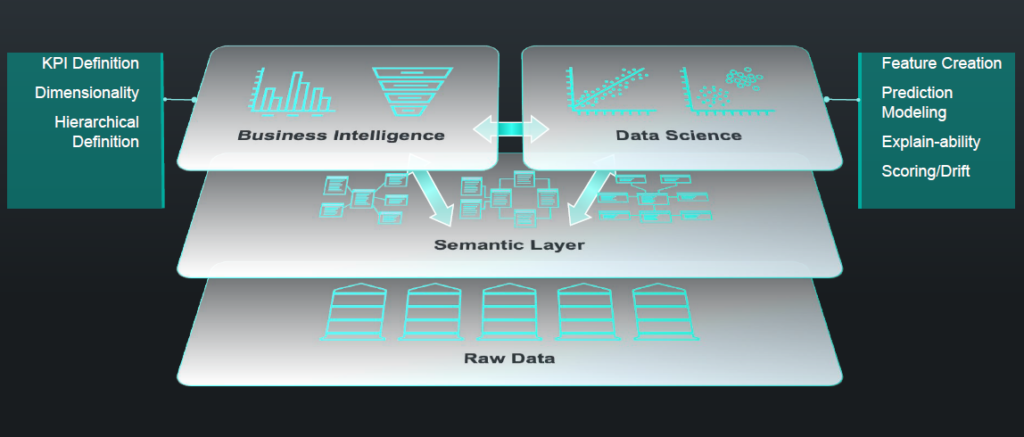
Demonstration
For a deeper dive, check out the full presentation.
ANALYST REPORT