ANALYST REPORT
2024 GigaOm Sonar Report for Semantic Layers and Metrics Stores
The GigaOm Sonar Report offers a comprehensive overview of the top vendors in semantic layer and metric store technologies. Download to learn why AtScale is positioned as a Leader and Forward Mover in Semantic Layers.
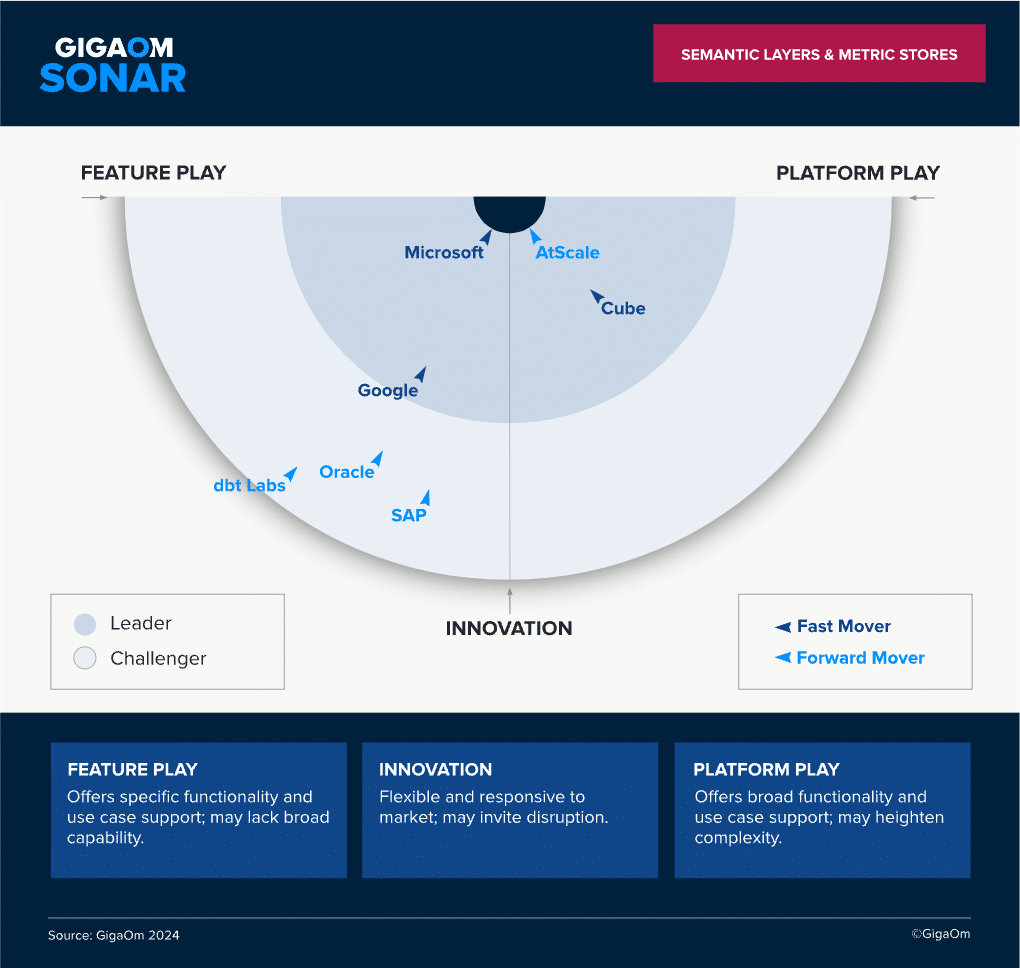