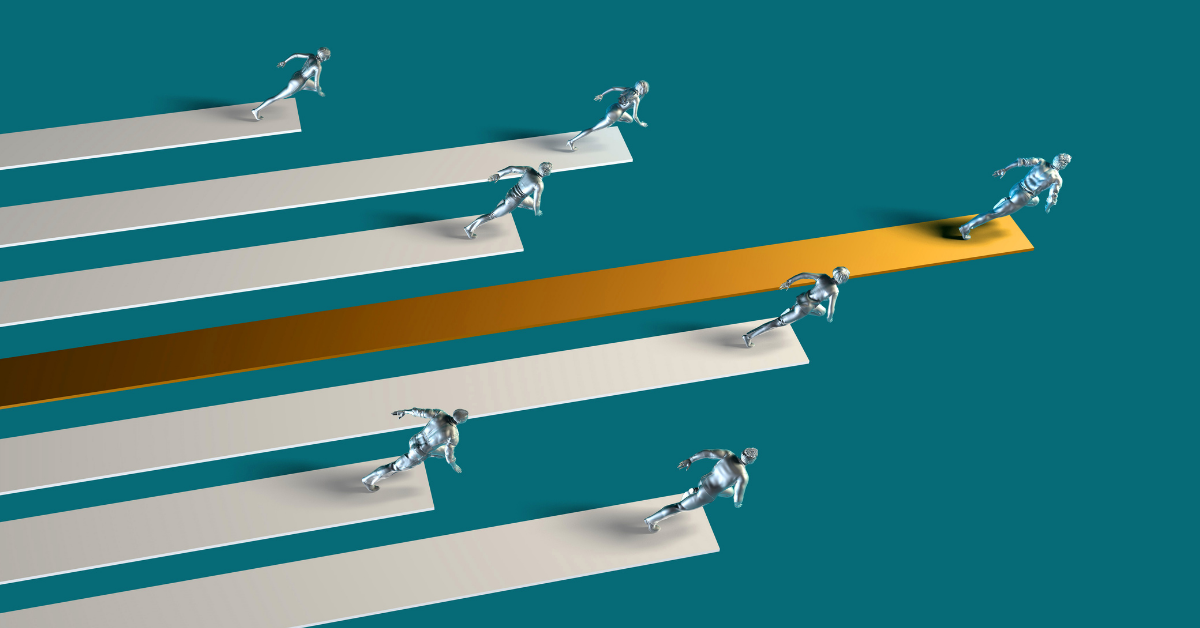
Data governance is a broad topic with a lot of players offering commentary and strategy across the data and analytics space. Governance isn’t only about security and access control, or who can access what; it’s also about how data is maintained and how it gets used. Data stewardship is the practice of ensuring that data is secure, usable, and reliable: governance is the implementation of these practices. Analytics stewardship and governance is the extension of the principles of data stewardship and governance with a focus on analytics, business intelligence and data science use cases. The understanding and application of analytics stewardship and governance is critical to creating a community of empowered, data-driven users who can intelligently leverage an organization’s data assets.
With the explosion in data, there are many technology providers proposing solutions to address portions of the stewardship and governance challenge. Data catalogs like Alation and Collibra focus on cataloging raw data and managing governance policies. Companies like Immuta and Privacera, meanwhile, focus on enforcing the security aspects of analytics governance, including cloud data and access control, by enforcing policies within the query path. Analytics catalog solutions like Digital Hive and Zenoptics focus on surfacing pre-built dashboards and reports. Given the variety in approaches, a semantic layer like AtScale is the natural place for analytics stewards to make raw data “analysis-ready” and to enforce the full spectrum of governance policies.
The benefit of implementing analytics policies at the semantic layer – for both access control and usage policies – is that users can be guided and coached how to interact with analytics in real-time. This empowers individuals to operate independently and with confidence that they are working with data in the right way. Since users can consume data through AtScale with tools of their choice (i.e. ad hoc analysis tools like Excel Pivot tables, BI tools like Power BI or Tableau, or scripting tools like Python), their policies can be maintained and enforced in a single location.
An Introduction to Analytics Governance
Broadly speaking, there are two categories of data governance policies: the first is focused on who can access what data, and the second on how those data assets get used. Traditionally, more attention is given to the security and access control. But the subject of how data is used is extremely important to building a culture of self-service where users confidently interact with data directly vs. requesting analytics from a centralized data team.
Analytics governance strategy for security and access control is table stakes for most any organization. Regulatory and compliance needs are the drivers behind many policies related to data privacy, consumer data protection, and securities regulations. Understandably, ensuring sensitive data is not improperly disclosed or exfiltrated from organizations is the concern of security and compliance teams.
For those reasons, access control is a key tool in ensuring proper protection of sensitive data assets. Extending access control policies from raw data to analytics is complicated. Enforcing policies at time of analysis is even more complicated. A semantic layer that integrates with active directory and data catalogs and BI tools can ensure that policies are reliably enforced.
When it comes to usage related governance policies, the importance of a semantic layer becomes even more apparent. Analytics are composed of metrics (like values, measures, KPIs) and the dimensions used to categorize, filter and sort information. Analytics governance includes the proper definition of metrics, including simple metrics like counts of unique occurrences or sums of values. It also includes properly defining key business metrics like revenue, bookings, costs and the calculation of more complex metrics like ARR, ASP, COGS, Margin and others. Analytics governance also includes definitions and hierarchical relationships for fields used for dimensions (like Geography, Time, Product Family) to better understand your data and to summarize data consistently.
Unfortunately, it is impossible to control the conclusions that data consumers draw from data assets. It is also impossible to guarantee that data consumers have the business savvy to generate true insights from data assets. It is possible to radically simplify the definition of data and to reduce the chance for misinterpreting key business metrics or analytics dimensions. By standardizing the definition of data assets and ensuring they are presented to consumers in a simple, business-oriented way (vs. a confusing set of field and table names only accessible through SQL code), a semantic layer can enforce these usage-related governance policies defined by data stewards.
Considering Governance of Augmented BI and AI-generated Insights
When we expand our consideration of data governance to analytics governance, we also need to think about data products that incorporate the predictions, recommendations, and relationships generated by AI/ML models. As organizations scale data science programs, more of these augmented analytics are available alongside data products based purely on historical data. Considering how to maintain and enforce governance policies for these AI-generated insights is becoming more important. By expanding the concepts of data governance to analytics governance, organizations can both protect and get more value from their augmented analytics. When access to AI-generated insights are also made available through a semantic layer, organizations can leverage the same infrastructure for enforcing both types of governance policies.
There are clear security and access control implications for many AI-generated insights. Sales forecasts for public companies need to be protected along with other summary statistics that could constitute inside information. Automated analysis of workforce productivity may have HR related implications that need to be protected. Insights generated from proprietary algorithms or data may constitute intellectual property that needs to be kept from competitors. Furthermore, applying data stewards alongside data security and compliance teams can ensure access control policies for these insights are maintained and enforced at the semantic layer.
Ensuring consistent and informed usage of AI-generated insights can also be a challenge to manage. While managers may be eager to leverage the outputs of AI/ML models, they need to be able to easily find, understand, and consume these augmented analytics. The same usage-related analytics governance principles apply here. Data stewards can then ensure that these insights are intuitive to understand, that analysis dimensions are consistent across historical vs. AI-generated insights, and that historical vs. modeled metrics can be compared “apples-to-apples.”
Real-time Enforcement of Governance Policies
To remedy these challenges, AtScale’s semantic layer solution sits within the query stream that opens up into much broader consumption tools. This means extra steps of applying governance to the semantics themselves.
By implementing a semantic layer within the query layer itself, organizations get real-time, accurate, governed data assets. This isn’t about just managing who sees what; the semantic layer manages what the things are, providing clarity and confidence to your facts. In other words, it is not just about defining policy, it is about using policies to shape how data is consumed.
By ensuring that all data consumers access data assets through the semantic layer regardless of the tool they use, organizations can drive consistency and predictability across the organization. Even analysts performing ad hoc business exploration analysis or data scientists experimenting with new models benefit from this level of control.
How Better Governance Empowers Your Company for Self-Service BI
In the semantic layer, data isn’t just protected––it’s defined.
Take a business problem that could be hard to define: say, the total revenue for the United States. What is revenue? What even is the United States? Are you including Guam, Puerto Rico, Washington D.C.? Where does this data come from? And when does this data come from? Is this from July 13th or August 4th? Is it a snapshot in time or dynamic?
This tail end can throw your data off a few points and introduce doubt. But in a semantic layer, those terms are defined by one person: the person who builds the model. Nobody else has to calculate complicated revenue, they just use it. The revenue number is coming from the AtScale layer verses from the calculations against the Snowflake model.
This empowers people to get better access to their data, applying it without bottlenecks, errors, or duplication of efforts. Without a Semantic Layer, even if content was governed from a security perspective, it wasn’t actually designed to maximize success.
Previously people had to manually check their results against each other. There’s been a bottleneck of data science teams doing their own work to parse data. That slows you down and introduces opportunities for human error.
With a semantic layer providing governance, you’re able to protect your information and data, not just from bad actors but from your own human error, instead empowering you to take BI into your own hands with clarity and confidence.
Doing More With Governance
Ultimately, governance isn’t just about protecting data; it’s about empowering people to use that data. Similarly, self-service BI isn’t just giving people access; it’s confirming they can’t mess that up.
Together, the AtScale semantic layer approach meets security and compliance needs while removing the bottlenecks and the doubts around actually applying your data.
To learn more, you can contact AtScale today.
SHARE
WHITE PAPER