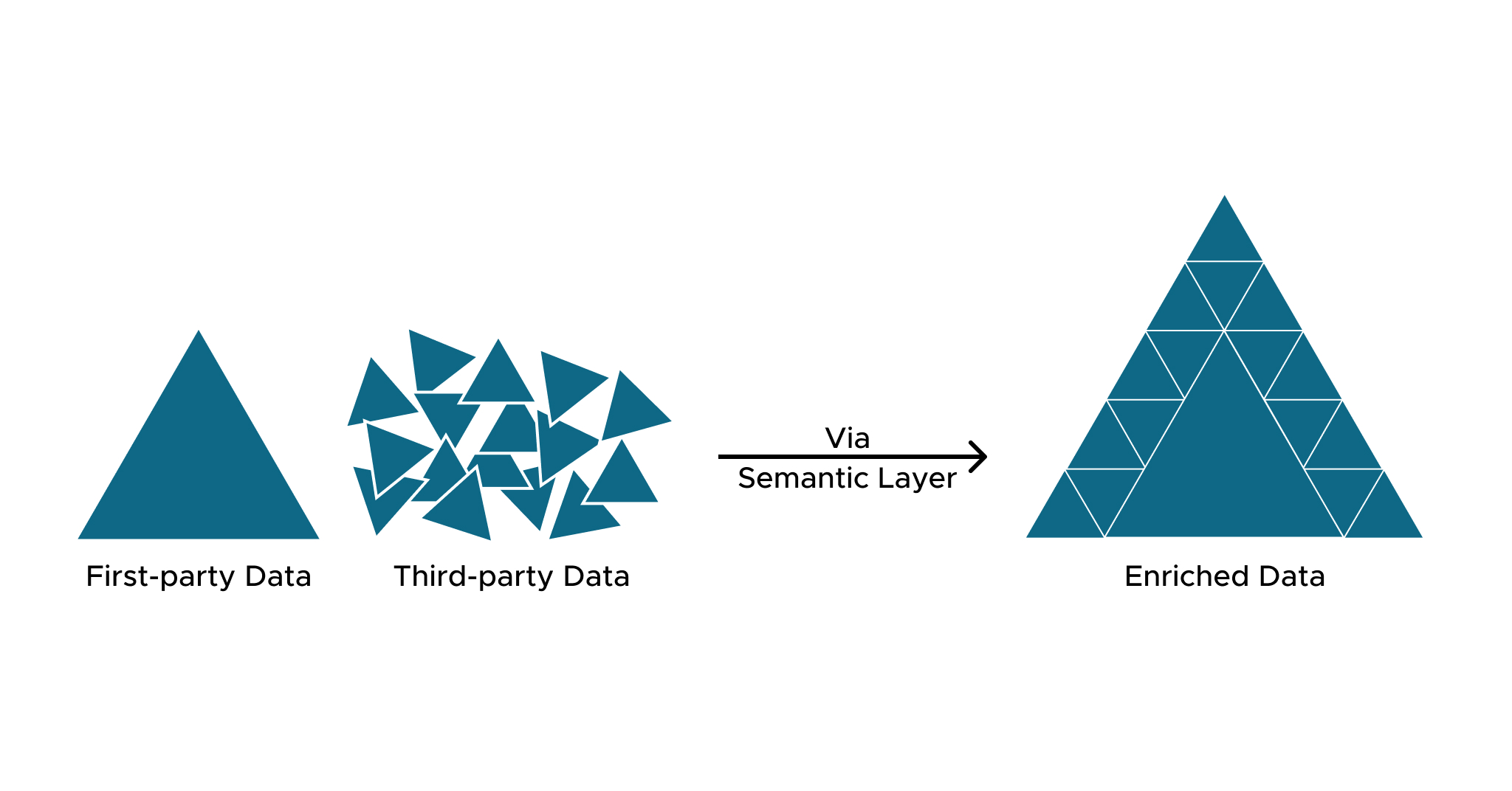
When it comes to effectively consuming data for business insights, there are six key areas: data, access, model, analyze, consume, and insights. AtScale’s Data and Analytics Maturity Model Workshop explains how organizations can build the skills and knowledge necessary to advance their capabilities in each of these areas.
The next post in this series covers module two, discussing how organizations that have achieved a centralized data repository can begin enriching this data with external sources. By further breaking down data silos – in this case the barrier between first-party and third-party data – organizations can create better business insights.
What is Data Enrichment?
Data enrichment means combining external third-party data with internal first-party data to improve analytics outcomes. First-party data is information that your organization collects from customer interactions and business operations, such as sales, website visits, and inventory.
Third-party data is information from an independent vendor that has collected statistical data or actual data such as foot traffic, weather, or demographics. These third-party data sets are available for purchase so that organizations can integrate them with their first-party data to get deeper insights.
During the pandemic, third-party data became crucial for many companies to understand market changes. For example, a number of AtScale customers were able to blend third-party data with their own first-party data to manage their inventory by better predicting demand.
The Data Sharing Landscape
The data sharing landscape includes a number of key players:
- Data Providers collect and package data sets for purchase and resale. For example, companies like Foursquare and SafeGraph offer location and foot traffic data sets for a better understanding of consumer behavior.
- Data Marketplaces provide a catalog of data sets from data providers. Marketplaces like AWS Data Exchange and Snowflake Data Marketplace enable organizations to more easily find third-party data sets and integrate them with their data warehouses or data lakes.
- Open Source APIs enable customers and data providers to share data directly with data consumers. Databricks recently announced Delta Sharing, which is an open protocol for making secure data sharing much simpler.
Challenges with Blending Datasets
Although data enrichment is crucial for generating deeper business insights, there are a few challenges preventing organizations from blending datasets.
Unfamiliarity: Every third party that collects data will package it with different metadata and schemas. Organizations will be unfamiliar with the data provider’s format and will need to spend time gaining an understanding of how these external data catalogs and data definitions can fit in with their own data.
Logistics: There are logistical challenges with acquiring, combining, and updating data from external sources. Organizations will need to determine how they will get the data into their data warehouse or data lake, how they will merge this new data with their existing data, and how they can efficiently repeat this process on a regular basis.
Modeling: It can be challenging to model the data when there are different granularities between data sets. For example, an external data set may collect data on a weekly basis, but an internal data set may contain daily numbers. This creates complexities when creating consistent data models for use throughout the organization.
Best Practice: Leverage a Semantic Layer for Data Enrichment
The semantic layer is a solution for overcoming the challenges of unfamiliarity, logistics, and modeling when enhancing your first-party data with third-party data. More specifically, AtScale’s semantic layer can apply governance rules and data virtualization to merge third-party data with the business model of the first-party data. This drastically reduces the time-to-value for data enrichment.
As the data sharing ecosystem has grown, there is now a wide variety of data sets available and likely a solution for every organization. When you combine external data with your existing data, you will have a much richer data set for analysts and data scientists to make decisions and predict the future.
Watch the full video module for this topic as part of our Data & Analytics Maturity Workshop Series.
SHARE
Case Study: Vodafone Portugal Modernizes Data Analytics