October 18, 2019
Should You Build or Buy Your Universal Semantic Layer?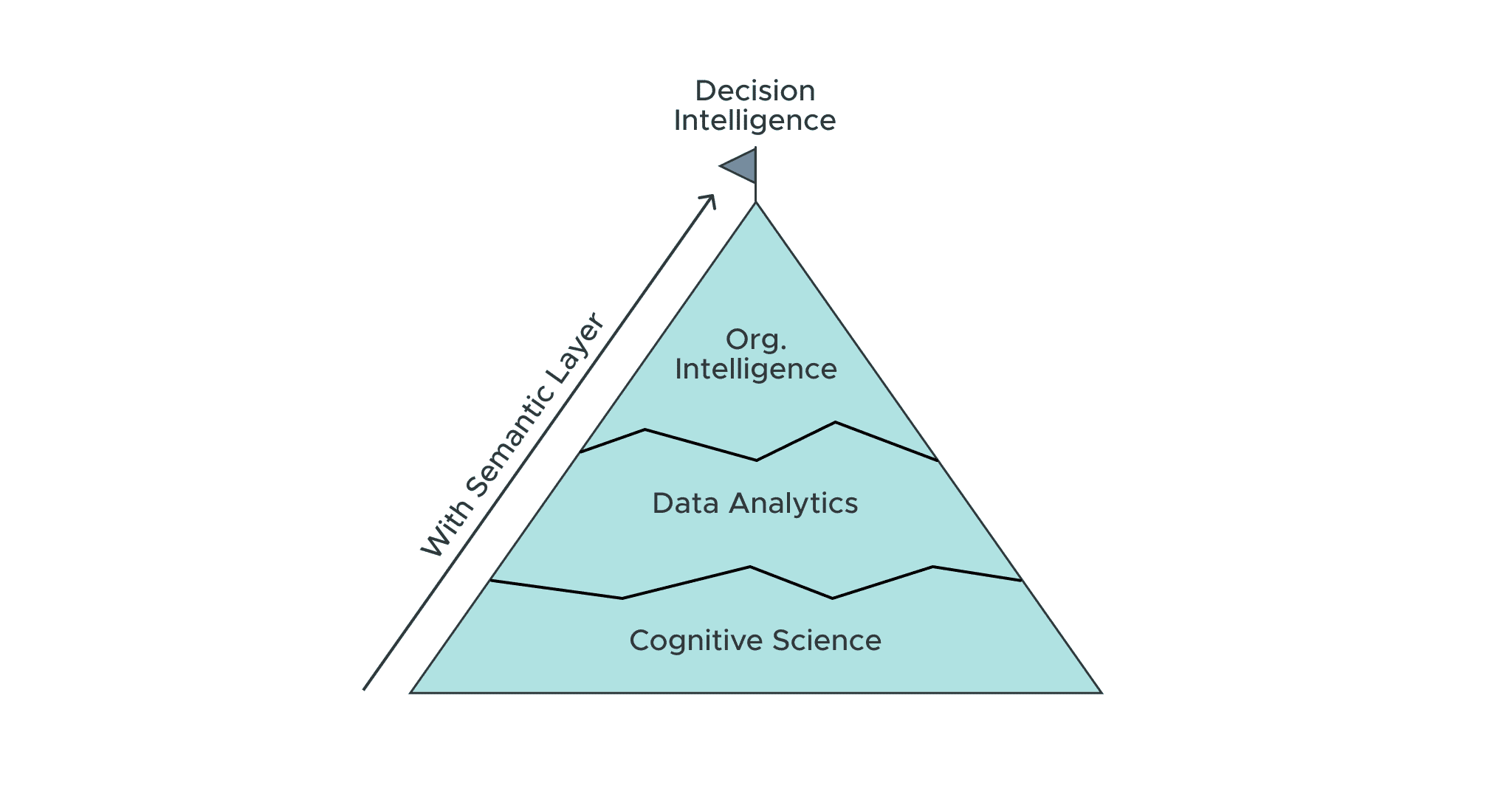
How To Implement Decision Intelligence in Your Enterprise With A Semantic Layer
On average, firms invest over $38 million in data and analytics. However, more than 79% of these firms end up with zero or negative returns on this investment.
Why is it rare for analytics projects to identify valuable insights for the target audience? Why do most teams fail to translate insights into actionable decisions? And, when initiatives manage to bridge the gap between data and effective decisions, why do teams struggle to repeat it at scale across the organization?
Chances are that most teams are missing decision intelligence (DI).
DI is the use of actionable data insights to deliver effective, impactful, and timely decisions across an organization at scale.
We previously discussed why DI is the most important data anlytics trend and we looked at three elements of every good decision to understand the factors that influence decision effectiveness. We reviewed the three disciplines that makeup DI, namely Cognitive Science, Data Analytics, and Organizational Intelligence.
This article covers a step-by-step approach to implement DI and highlights fundamental principles from each discipline with an industry example. We’ll also discuss how a semantic layer helps orchestrate DI at scale across businesses.
1. Cognitive Science: Better Understand the Decision-Maker and Their Audience
Decision-making is the cognitive process of selecting a course of action among several possible alternatives.
The two key parties involved in any decision are the decision maker (self or a third party) and the target stakeholder (those who act on decisions or get impacted by them). The discipline of cognitive science helps understand both these sets of stakeholders using techniques to decipher how the human mind works.
One must begin by understanding the decision maker’s goals, priorities, and pain points. Map the persona by collecting information about their demographics, capabilities, and access to tools. Understanding their typical decision-making process, historical decisions, and achieved outcomes is essential.
Most teams skip this step and instead jump straight into collecting the data or analyzing it for insights. This is like choosing a birthday gift without knowing who you’re buying it for. Just as there is no ideal gift, there is no best decision without contextualizing it to the humans involved.
These details are crucial not just to make a decision, but also to choose which decisions need to be prioritized in the first place. This helps understand which decisions really matter and for whom.
Business Application:
Let’s take the example of a large electronics manufacturer that attempted to grow revenues by improving customer experience (CX).
The organization began collecting information about the product manager (the decision maker) and customers (the target audience). The team mapped the stakeholder persona, their technology understanding, and access to tools available.
The team then analyzed product managers’ roles and responsibilities. They looked at how product managers interacted with customers, responded to their queries, and acted on the historical voice of customer (VOC) feedback cycles. They also studied the historical customer onboarding, engagement, and exit, and spoke to customers to conduct secondary research to understand customer preferences and buyer behavior. These are all examples of strategies used to better understand decision makers and their audience.
2. Data Analytics: Answer Business Questions With Actionable Insights
With a window into the minds of the key stakeholders, it’s time to understand the business challenges they are grappling with. To do so, it’s important to frame the questions they are trying to answer, identify the insights needed to arrive at the optimal course of action, and identify the possible choices they are faced with.
Once the important questions are framed, identify how they can be answered using data analytics. Collect the data needed and choose the relevant analytics techniques to identify insights. Validate whether the insights can be actioned with business decisions and if they properly address the questions identified above.
In this step, many teams make the mistake of not translating the insights into a format that is consumable by the audience. This leads to that last-mile disconnect that fails initiatives. Data storytelling is a technique that makes data insights easy to understand and engaging.
Business Application:
The product manager of the electronics manufacturer we examined earlier was facing the challenge of low customer engagement and lost revenues. They wanted to understand what drove customer satisfaction and what made customers a promoter or a detractor.
The product managers wanted insights that would help improve the net promoter score (NPS) and inform decisions that would grow the company’s share of the customer wallet. The customer experience analytics team identified data sources such as customer transactions, service tickets, voice of customer survey responses, and social media comments. The metrics and analytics computations were performed in a business layer to ensure standardization and repeatability.
By applying statistics, text analytics, and machine learning, the team identified the top three drivers of customer satisfaction. They found that prompt resolution of customer service issues had the greatest chance of converting a neutral customer to a promoter. Customers who rated the company high on product features had the highest propensity to spend more.
The insights identified were converted into data stories for easy consumption by the product managers.
3. Organizational Intelligence: Contextualize Decisions and Influence Outcomes
Decisions are only as good as the actions they impact. While the earlier two disciplines are critical to arrive at a decision, this discipline ensures that the actions are taken and targeted outcomes are achieved.
Decisions must be adapted to the organizational context to ensure relevance for users. They must be institutionalized with processes to make the entire decision lifecycle repeatable.
Organizations must evaluate whether users are enabled with the right skills for data-driven decisions. In many organizations, decisioning with data introduces risks for the users due to business-as-usual practices that inhibit change. Leaders often overlook the cultural resistance that could derail data analytics initiatives. It is the leaders’ responsibility to tackle such dysfunctional behavior and drive change.
Organizations must empower teams with the right technology, platforms, and governance to facilitate decisioning with data. It is important to build in a feedback loop with objective measurement to assess the quality of decisions and continuously course-correct on decisioning.
Business Application:
In our example, the organization brought in interventions across people, process, and technology areas:
- People: The product managers were enabled with data literacy training in addition to product training. They were provided extended support in the Go-Live phase by shadowing application usage and addressing queries. With strong executive sponsorship, the initiative got high internal visibility, and teams were incentivized to act on the new data-driven insights.
- Process: The customer engagement workflow was redesigned to leverage insights from the data and analytics platform. Product managers made major decisions after the quarterly VoC cycle. A feedback loop was institutionalized to assess the effectiveness of decisions and tailor strategic decisions in the subsequent quarter.
- Technology: The data and analytics platform was integrated into the technology architecture and process flow for automation, repeatability, and scale. This made it easy to expand the CX analytics by ingesting newer data sources such as competitor surveys and adding newer business lines or product teams.
These interventions led to a jump in net promoter score of 5.5 points with an incremental annual customer revenue of $50 million.
The Role of a Semantic Layer in Institutionalizing DI
We’ve seen how to implement decision intelligence by rolling out actions across the three disciplines systematically. The business example highlights how this can materialize in the real world.
Let’s summarize the three ways a semantic layer helps scale DI across the enterprise:
- Data Integration: A semantic layer supports data blending across multiple data platforms and data sources. It helps understand the decision maker and target stakeholders by collecting data about them, such as demographics, transactions, and usage behavior from various source systems.
- Semantic Modeling: To perform data analysis, teams need access to the business meaning of data and an understanding of how data relates to other data. The business metrics should be easily accessible from a central layer. A semantic layer achieves this by avoiding costly (re)computations by individual applications or distributed teams.
- Consumption Integration: For contextualization of decisions and easy actioning by users, the consumption layer must be integrated with all relevant business applications. The performance feedback must be fed back to the analytics applications to enable validation and course correction. A semantic layer enables democratized data governance and access to the right data by the right person at the right time.
Note: This is the second post in a blog series about Decision Intelligence. Check out the first post in this series: Why Decision Intelligence is the Most Important Data Analytics Trend of This Decade.
ANALYST REPORT