April 13, 2023
Actionable Data Insights for Improved Business Results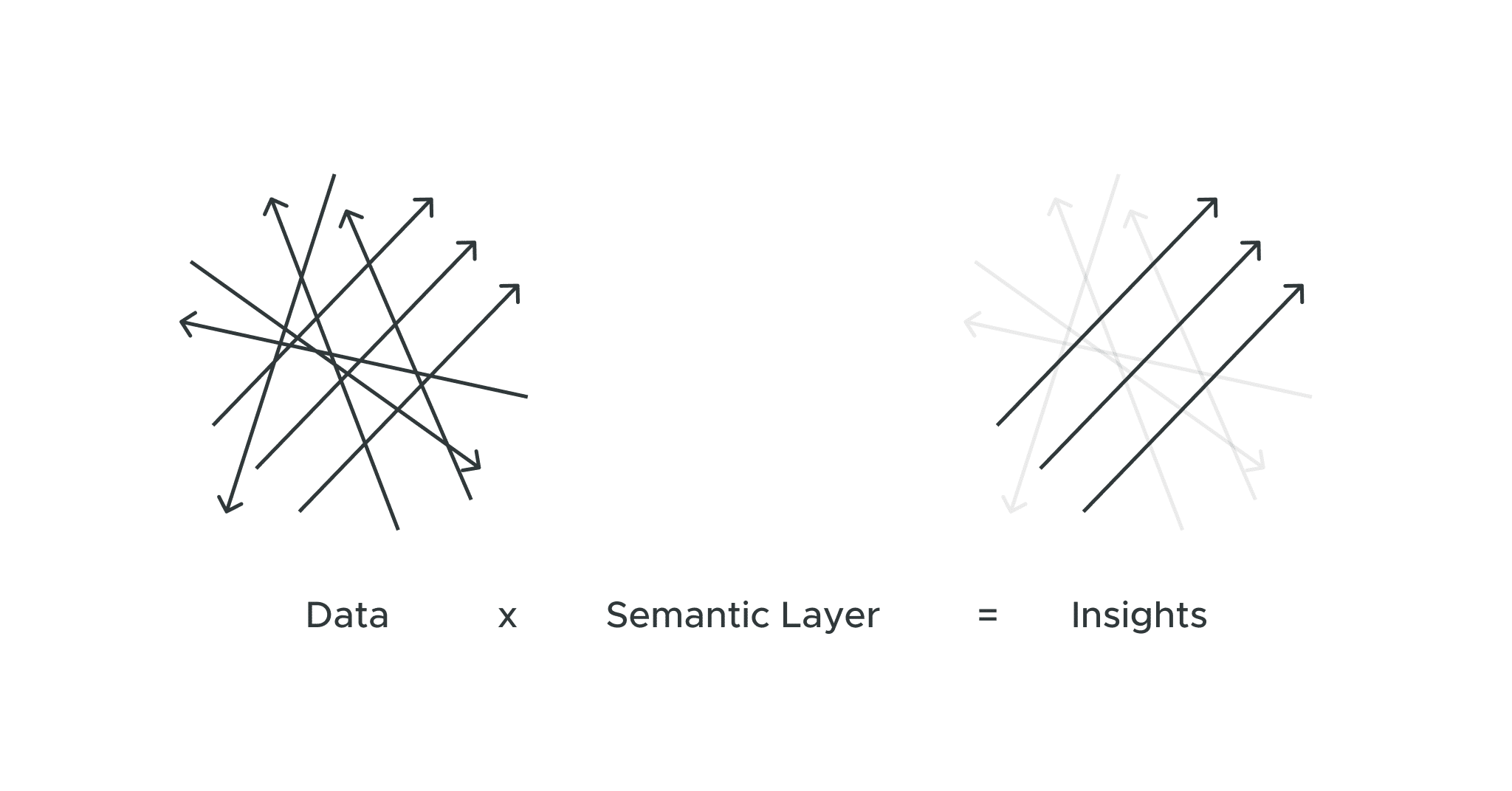
There are six key areas for effectively consuming data for business insights: data, access, model, analyze, consume, and insights. For organizations looking to advance in each of these areas, AtScale’s Data and Analytics Maturity Model Workshop explains how teams can build their skills and knowledge.
In this blog post covering module nine of the workshop, we discuss the four levels of data insights and how a semantic layer helps organizations leverage them.
Insights in the Data and Analytics Maturity Model
As we’ve mentioned throughout our workshop, the insights category is the final step in the data and analytics maturity model. Insights give context to data, making it usable for informed decisions and business growth. Predictive and prescriptive insights, in particular, empower businesses to predict and change future outcomes, based on what’s happened in the past. Data insights fall under four different categories:
Descriptive
Descriptive insights are the most basic of the four categories at Level 0 of the Data and Analytics Maturity Model. They simply describe what’s happening in the data. Generally, businesses use a query or text-based tool to gain descriptive insights. They can answer a question like “what was our total revenue for Widget A in 2021?”
While descriptive insights used to be foundational to data science, they are often irrelevant for drawing conclusions and making informed decisions in today’s world. They only focus on past events, with no way of forecasting what will happen in the future.
Diagnostic
Diagnostic insights seek to answer the question “why?” Many companies started to gather diagnostic analytics after the introduction of BI tools like Crystal Reports. Diagnostic insights focus on visualizing data with graphs/charts, then drawing conclusions by “slicing and dicing” data with these various tools. Diagnostic insights can answer the question, “why was our total revenue for Widget A in 2021 higher than our total revenue for the same product in 2022?”
Diagnostic insights play an important role in today’s organizations but are still only backward-looking. So, they need to be paired with other types of insights, in order to truly provide value for an organization.
Predictive/Augmented
Predictive/augmented insights, in Level 2 of the Data and Analytics Maturity Model, require augmented analytics to succeed. This means that they rely on AI/ML technology to draw intelligent conclusions. Predictive insights, in particular, leverage machine learning to review what has happened in the past and forecast possible outcomes. They seek to answer the question, “what is likely to happen in the future?”
An example of a predictive insight is “what is our estimated revenue for Widget A in 2023?”
Prescriptive/AI
Prescriptive/AI insights is the most advanced category. These analytics use data to create educated suggestions on the best next steps for a given situation, answering the question “how do we make something happen in the future?” Just as with predictive insights, augmented analytics are required to enable prescriptive insights. These insights could answer the question, “What next steps should we take, to make our revenue for Widget A in 2023 higher than our revenue for this product in 2022?”
These types of insights allow for autonomous decision-making and reusable data, as they rely less on human intervention and more on advanced ML to draw intelligent conclusions. Prescriptive insights create immense value for fast-paced businesses by enabling automation for next best steps and changing outcomes for the better. They go beyond just identifying what will happen (e.g. “we will likely experience a 20% decrease in sales for Widget A in 2023) by taking action steps to change that probable outcome (e.g. we will likely keep sales for Widget A up by offering promotional deals to X specific customers).
How a Semantic Layer Helps with Data Insights
It takes multiple types of insights to answer the different questions that a business asks on a daily basis. But, there’s one component that’s important, regardless of your level in the Data and Analytics Maturity Model or which types of data insights you are leveraging. That factor is data accessibility. Teams need to be able to access the data and analytics uncovered by data insights. It’s especially important for the data from augmented analytics to be open and accessible. AI/ML technology is a significant investment, so it’s in your company’s best interest to make it available to any employees who need it. If some of your teams can’t see the data, they can’t use it to make better decisions.
This is where a semantic layer comes into the picture. A semantic layer creates common terminology for data. It empowers various business units with different objectives to access and use the same data. The semantic layer accomplishes this by standardizing how data insights get stored, labeled, and tagged in the cloud.
A semantic layer ultimately makes descriptive, diagnostic, predictive, and prescriptive analytics available to every role that needs them: from data science modelers to decision-makers and everyone in between. It democratizes backward and forward-facing insights, empowering all departments to use the powerful reporting, predictions, and suggestions that data insights tools provide.
Watch the full video module for this topic as part of our Data & Analytics Maturity Workshop Series.
ANALYST REPORT