July 18, 2024
Revolutionizing Digital Analytics: Snowplow Analytics’ Warehouse-Centric Strategy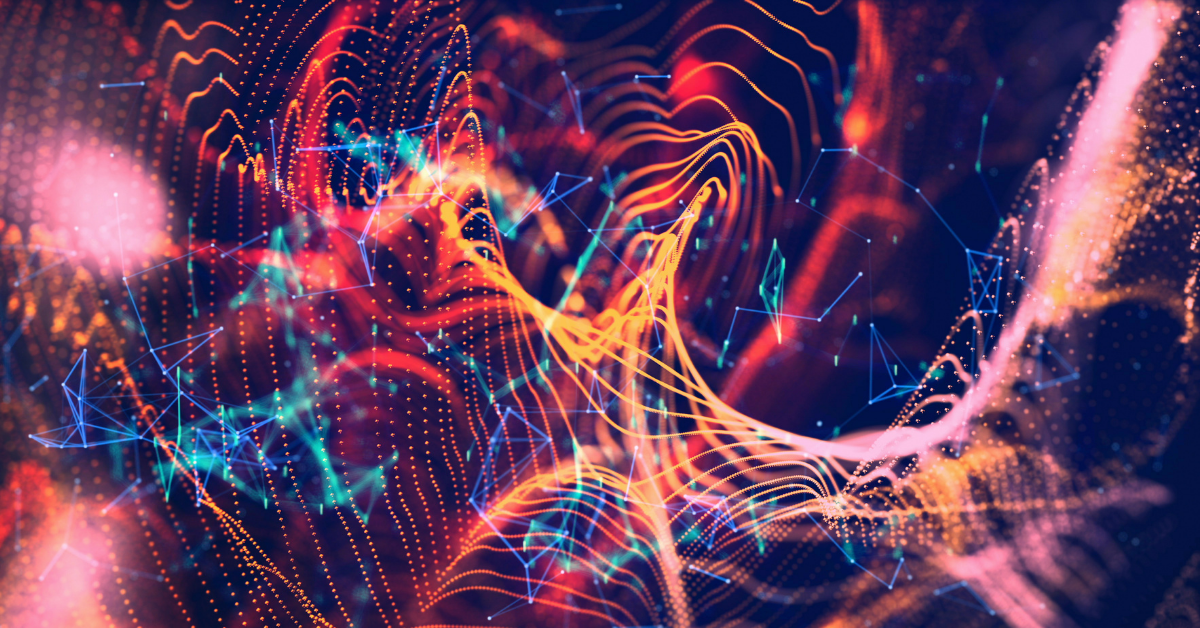
Modern organizations increasingly rely on their analytics programs to help them stay competitive. And, while most every organization is leveraging the massive amounts of data available from their enterprise applications and from 3rd party data providers, it is increasingly common for organizations to leverage data science teams and powerful enterprise AI platforms to augment analytics with AI/ML generated insights. The goal of this advanced data analysis is to provide decision-makers the tools to operationalize insights by taking more informed actions that generate real value.
Put another way: even if decision makers can see what is happening, gain insight into why it happens, and foresee how it will continue to happen, their organization still must figure out how it can leverage that data to actually change their future.
Prescriptive Analytics are a type of AI-augmented analytics that make discrete suggestions on specific actions to take to achieve a goal. And, by applying Prescriptive Analytics, organizations can gain context-driven intelligence to guide their actions.
The Spectrum of Augmented Analytics
Many organizations apply data analytics to help them understand, sort, and use their data to improve their business. And, after filtering and connecting data through a semantic layer, there are usually three types of analytics applied by most leading organizations.
These three types of commonly used analytics are:
- Descriptive Analytics, which provides a historical view of events, letting people in an organization understand what happened in the past. By parsing what has happened, with clear definitions, organizations gain insight into historical data from which they can draw comparisons.
- Diagnostic Analytics, which helps to explain why things happened the way they did, applying tactics like data discovery and data mining to surface correlations and explain why something happened. This is crucial to understanding an organization’s data at a deeper level, allowing organizations to dive deeper into their data to draw out proper causation.
- Predictive Analytics, which helps to predict what will happen in the future based on the current models and possible changes. Since predictive analytics works to project future outcomes based on collected data, it works in conjunction with complex statistical modeling to help showcase risks and opportunities.
These three types of analytics are fairly easy to understand, and are table-stakes for many organizations. They help organizations chart where they’ve been, where they are, and where they’re going, which provides valuable insight.
While these analytics are useful for processing and gaining insights from massive amounts of data, they still put the onus on decision makers to choose a course of action based on the analytics they consume. Deciphering your information still requires an immense amount of manual work, leading to a cognitive bottleneck––leaving your data under-utilizied and unapplied.
The Impact of Prescriptive Analytics
As data analytics have matured, we’ve gone from hindsight in analysis to oversight – and even foresight. While these types of analyses help organizations to chart the past, present and possible future, Prescriptive Analytics goes a step further, giving decision makers suggestions of specific actions to take.
For example, let’s say you have a model for predicting customer churn. While a Predictive Analytics model might allow you to plan for that high probability outcome, the Prescriptive Analytics model will suggest actions to reduce the probability of churn.
That means instead of merely predicting what can happen, your model can now work automatically to take the steps needed to change the outcome. For example, instead of only observing potential churn customers, a Prescriptive Analytics model would allow you to automatically send them a 20% coupon or take another relevant, next-best action to prevent churn.
By autonomously responding to your information in real time, Prescriptive Analytics empowers your organization to not just map the future but to actively change it.
The key to Prescriptive Analytics is that augmented intelligence can process your existing information and emultate cognitive decision making to take necessary actions or make suggestions to a decision maker.
How Prescriptive Analytics Changes Your Future
Unlike Predictive Analytics, which models out how a potential future might look, Prescriptive Analytics is designed to help you change it.
In the past, Predictive Analytics might allow decision makers to identify and flag a problem, but it was unable to recommend a context-driven solution. This means that ultimately the decision-maker would have to go with their gut, applying their best-practices or manually testing their hypothesis within the data.
Unfortunately, an educated guess is still a guess, and those human-choices come with their own biases. Despite the best in static data modeling and human talent, Predictive Analytics leaves gaps that Prescriptive Analytics moves to close.
The largest difference is that Prescriptive Analytics is directly tied to context-driven actions designed for your optimal outcome while Predictive Analytics merely models out futures and requires your team to interpret the signs themselves.
To better illustrate this example, think of the medical world, where predictions and prescriptions both come into play. An intelligent medical prediction can certainly be helpful––but without including a clear action to take, it puts the onus on a decision maker to chart their path forward, which may include bias and human error. In comparison to a mere prediction, a prescription can offer you an actual data-driven decision and solution.
In the same way organizations gain the ability to solve complex decisions with millions of decision variables, constraints and tradeoffs with Prescriptive Analytics to find their best solutions. And, by applying context-driven decisions at scale, Prescriptive Analytics can uncover interactions in the data and translate them into clear recommendations that would otherwise be too complicated for organizations to understand alone.
Ultimately, the strength of Prescriptive Analytics is that it can interpret the massive amounts of data already collected, process it, and turn it into actionable and context-driven recommendations to solve problems, not merely to chart them.
And for your organization, that’s sure to make a difference.
ANALYST REPORT