June 21, 2022
Rethinking Feature Stores to Better Align AI/ML to Business Impact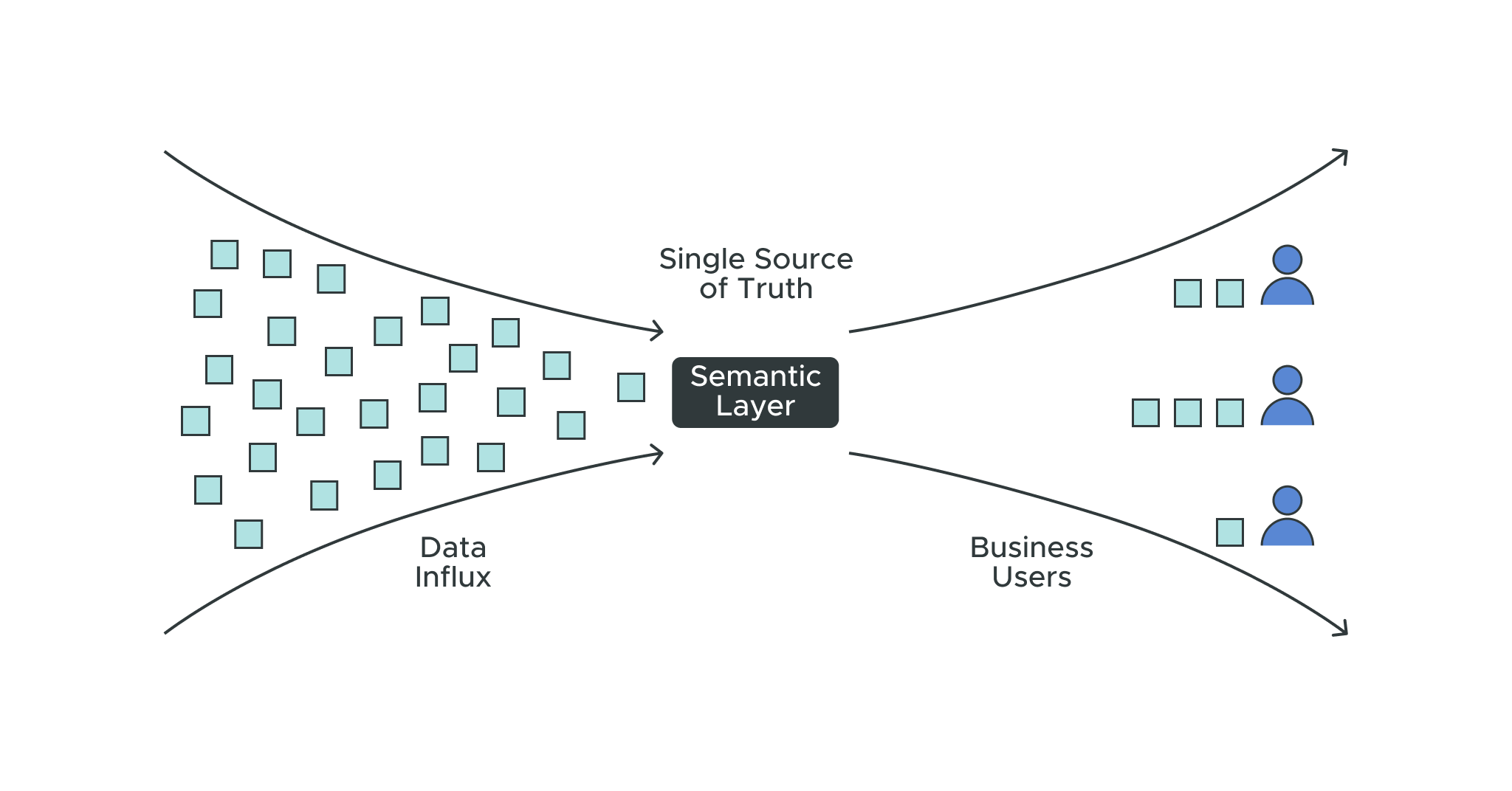
The influx of data has increased exponentially over the last decade. As illustrated below, data creation is expected to grow by 86% by 2025 due to an increased digital footprint during the pandemic.
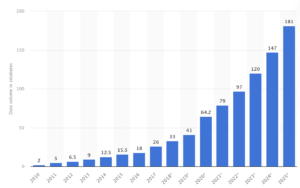
Source: https://www.statista.com/statistics/871513/worldwide-data-created/
Many organizations have become data-rich and want to gain a competitive edge with a deeper understanding of their customer base. Traditionally, organizations have relied heavily on BI tools and techniques to generate insights into customer behavior and preferences. Such insights often enable organizations to discover patterns that drive the core business strategies and decisions.
Using Data to Answer the Right Questions
Modern BI tools extend to the use of AI and provide forward-looking visibility and predictions to the business. With these tools, organizations can fulfill their objectives by preparing for them in advance rather than diagnosing the “what?” and “why?” from the past data. This has become increasingly more important today, as data becomes stale quickly. But with AI, organizations can understand and predict the dynamically shifting needs of the customers and the overall environment in general.
We have seen several advanced algorithms in recent times, which help manage a significant data upsurge. But for data to work for us, we need to ensure the emphasis remains on the semantic dimension of data. An increase in data availability must be met with solutions to provide better context and consistent data definitions.
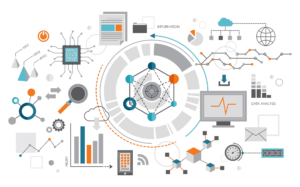
Source: Performance icon vector created by rawpixel.com.
Data Analysis in Context
Consider a case where a retailer notices a lot of deviations in order delivery timelines and wants to know whether a specific order can be delivered on time. The retailer knows about the order delivery and can implement proactive fixes within the supply chain to deliver high-priority orders on time. This is characterized as a predictive maintenance problem.
This business problem requires the retailer to ask some clarifying questions to analyze the issue accurately:
- How do I define an order, and what does it mean for an order to be successful? An order may only be considered successful if a delivery is met in full (ex. 100 of 100 units delivered). Or, it could be considered successful if it meets a minimum service-level agreement (ex. 80 of 100 units delivered, with a service-level agreement of 80% and above).
- How far in advance must the retailer be notified of the delivery status? If the retailer requires visibility into the delivery status two weeks before the scheduled delivery date, the time window is T+14 days.
- What does it mean to be “on time” and when does an order enter the supply chain? An order could be classified as “within the supply chain” on the date of invoicing or on the date on which an order is confirmed.
- What is the delivery date? Is it the date of shipment to the distribution center or a retail store?
Confirming these definitions is a critical step in defining the goals and objectives of an AI/ML project. Everyone needs to align with the same definition of the data.
ML algorithms expect the training data to be in a single flat structure and often undergo an extensive ETL process involving multiple joins, merges, and transformations.
Correct data preparation involves extensive domain and business knowledge and is crucial for the project’s success. But often, such tedious data integrations force organizations to build an expensive DIY solution.
DIY software is very costly for an organization to build and maintain, as compared to preconfigured and optimized software.
The semantic layer removes all such data integration woes by enabling even non-technical business users to work with data through self-serve analytics tools.
The Power of a Semantic Layer
All business users must speak the same language about data and work from a single source of truth. Inconsistent data definitions and a lack of context can derail a data analysis project and lead to setbacks within an organization. A single source of truth ensures that everyone on the team is aligned with the same reporting metrics from which to conclude. This ultimately speeds up the time to act. In other words, it ensures no two analysts work on the same dataset but use different measurement units.
Besides yielding a faster turnaround to insights, the semantic layer brings another important element to the table — trust.
Quite often, business leaders worry about the trustworthiness of data insights. With a semantic layer, analysts feel more confident about the data quality and how data is being interpreted and used across an organization.
Decoupling Data Science Dependencies
Bad data is undoubtedly a bump on the road. It is relatively easy to identify bad data within traditional software tools. However, in the case of AI solutions, bad data can act as a silent killer.
With AI tools, data could be generated from external sources such as social media posts, user reviews, and customer surveys. In such cases, there are many ways data quality can suffer. Cleaning up such data is a tedious task and requires a lot of manual effort.
On the other hand, enterprise data is often well-maintained in sanctity but requires an additional step of conforming to the same end goal and objective.
Data sourced from multiple streams pass through multiple steps of data cleaning, preprocessing, and data transformation before being fed into the model. Model predictions are then compared with the ground truth to evaluate the model’s goodness. It is during this stage of error analysis that a lot of data issues surface.
In most situations, the data science team completes the feedback loop by sharing the findings from their manual analysis and data cleanup efforts. This leads to frustrating feedback cycles and longer turnaround times, where the team is blocked from moving forward on a project until the data gets fixed at the source or origin.
Plus, data science often relies heavily on experiments. And experiments, by nature, are iterative and time-consuming. The semantic layer empowers data scientists and enables them to be self-sufficient regarding access to the correct data. Put simply, the semantic layer makes self-serve analytics easy and feasible by abstracting away the complexities of multiple data sources. It removes the bottleneck of data accessibility, improving the product development lifecycle significantly.
Gaining a Competitive Edge
A semantic layer provides a competitive edge for organizations by enabling them to define and contextualize data for all business users. The use of a semantic layer allows for easy access to data and ensures users remain consistent in the way data is defined and interpreted.
ANALYST REPORT