July 7, 2022
The Business Impact of Using a Semantic Layer for AI and BI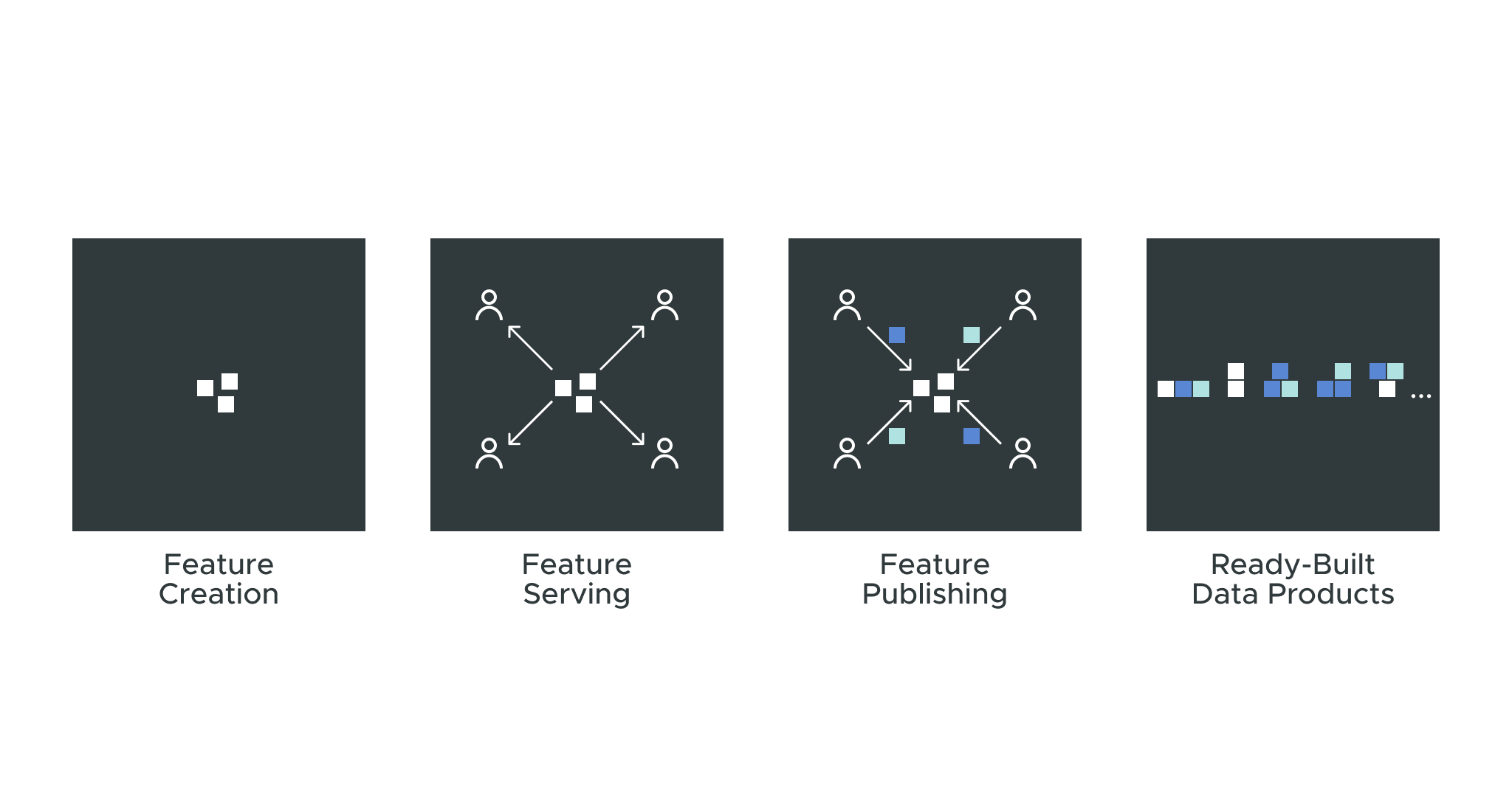
At AtScale, we love talking to data and analytics technologists and hearing their stories. We recently hosted a tech talk (presentation and demo) with Ben Taylor, Chief AI Evangelist at DataRobot, about how AtScale and DataRobot can help align AI and BI teams to drive a greater business impact.
DataRobot’s Experience Delivering a Best in Class AI Data Platform
Ben shared with me the origins and some key highlights about the evolution of DataRobot’s AI Platform. Back in 2012, the company founders set out to automate data science. While the concept of AutoML is mature today, there was a lot of pushback because data scientists viewed their modeling activities as more of an artisan’s craft than a procedural science.
DataRobot was able to break through the skepticism with a best-in-class AutoML platform, and much of the rest of its evolution was a natural progression of finding opportunities for business value through its technology. For example, the need for quick, maintainable, and reliable deployments guided the company into MLOps.
What’s impressive, though, is the boldness of the vision that led to DataRobot positioning itself, as Ben put it, to own “A to Z” in the AI lifecycle. Through a combination of in-house innovation and strategic acquisitions, DataRobot essentially created the end-to-end AI lifecycle as we know it today.
How the Semantic Layer Accelerates Data Science and the AutoML Business Impact
Organizations know they need to deliver data-driven decision-making at every business layer, and Ben and I discussed how to get there. Achieving data democratization means taking data-driven decisions beyond the data experts with SQL skills. That starts with removing technical barriers so that decision support frameworks end up in the hands of the business experts rather than just data scientists.
The semantic layer does this by helping data teams bridge the best features of AI and BI, simplifying ML pipelines and greatly accelerating feature discovery. One of the most powerful capabilities of combining a semantic layer and AutoML is how model-generated insights get published to the business for further insights and iterations.
In our talk, Ben and I honed in on how the semantic layer creates value for AI/ML data platforms, and it comes down to four key capabilities:
Feature Creation
Feature creation starts with raw data, and AtScale can build a semantic model on top of any raw data set no matter where it is. I demonstrated during the webinar how we could use the semantic model to quickly build a business-friendly interface and a set of managed features that are tool and data platform-independent. We can quickly build complex features like time relative metrics without anycode using our modeling canvas.
Feature Serving
The features created then give us the capability to serve them up to different audiences using the tools of their choice. There is also a powerful capability to discover and query these features through the semantic layer to perform descriptive and diagnostic analytics for our business. From there, we can create data products so our business users can use that information without having to know the process or structures that created the products. The same thing happens with our data scientist personas, who can also access the semantic layer to simplify the data prep and focus on features already approved by the subject matter experts.
Feature Publishing
Data scientists can use these features as a starting point to create their machine learning models to predict the future. The next step is to develop new machine learning features in the DataRobot platform and publish those features back to the AtScale Semantic Layer, which becomes automatically integrated into the semantic model. The data is then stored back in Snowflake. With this process, we effectively combined the work of the subject matter experts building the semantic model with the AI experts creating the machine learning model to create new features that we can share across our business as ready-built data products for consumption.
Ready-Built Data Products
As we walked through this, Ben noted that this method of feature creation carries the benefit of extensibility. It’s easier for feature creation to evolve organically as the data needs of the organization change over time. The end result allows us to enable data products that incorporate augmented intelligence to drive better decision outcomes across the business.
DataRobot with AtScale Aligns AI & BI Teams and Improves Business Outcomes
So how does AtScale create value for AI/ML platforms like DataRobot? Ben’s perspective on this drives a future state where we can deliver faster time to value for data-driven decision-makers, expand our knowledge sources with easier access to data types and sources, and provide the all-important defensibility.
Defensibility is critical as business intelligence, and data science business cases grow to include high-consequence predictions in areas like healthcare and defense. Decision-makers need a high level of confidence and comfort to stand by these predictions, and the combination of AtScale with DataRobot delivers that confidence today.
AtScale with AutoML Helps Data Teams Simplify ML Data Pipelines to Drive Value for BI
The AtScale Semantic Layer is the single, unified source of truth for data scientists and business experts. It enables data teams to experiment with new views, metrics, and dimensions across all of a business’ data sets. Complex features like time-series data sets become greatly simplified even in the face of disruptions and changes to underlying data.
Sign up for an interactive demo if you want to see AtScale with AutoML in action.
ANALYST REPORT