June 21, 2022
Rethinking Feature Stores to Better Align AI/ML to Business Impact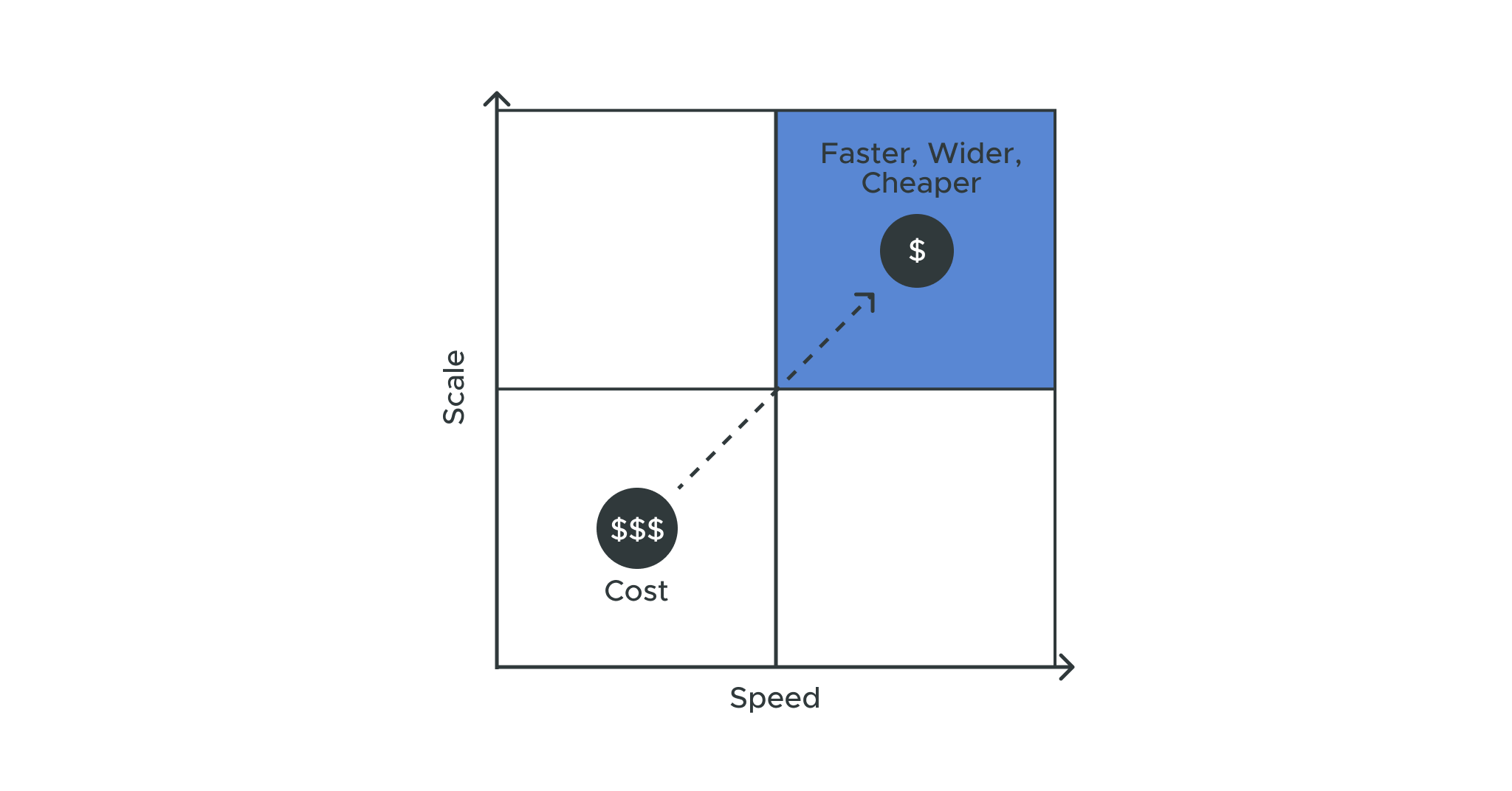
Companies are in the midst of making major investments in modern cloud data and analytics platforms to improve business growth, customer loyalty, efficiency, and profitability. Questions concerning if an investment to enable data, insights, and analytics is warranted have been answered with an overwhelming “yes” by most organizations. Now, companies are asking how to scale the delivery of insights and analytics beyond a few initial use cases.
As a recent McKinsey study Tipping the Scales in AI indicates, companies that scale insights and analytics achieve on average more than 8% points higher EBIT than companies who have not achieved scale. While there are many potential ways to improve the speed, scale, and impact of data and analytics projects, one solution which has been used effectively by enterprises over the past ten years is a Semantic Layer. The Semantic Layer is a critical component within the modern data stack that directly simplifies and accelerates insight creation by better leveraging AI and data investments.
Although the Semantic Layer is an established technology that is used by the world’s leading enterprises, many more companies are considering using a Semantic Layer, but have questions about what to expect from a business impact perspective. To understand the benefits and business impact of a Semantic Layer from those actually using the technology, DBP Institute prepared a survey for enterprise Semantic Layer users and received 108 validated responses during April 2022.
In this post we will cover some of the key findings from the survey, including the perceived benefits from using a Semantic Layer, the current challenges to achieving scale, and how the Semantic Layer addresses those roadblocks and delivers the desired business outcomes.
Achieving Speed, Scale and Cost Savings
The Semantic Layer emerged as a concept for enterprise data and analytics organizations to scale insight creation. By supporting decentralized insights creation with centralized data governance and data management, the Semantic Layer enables companies to develop and deploy data products (e.g. data models, metrics, and insights) rapidly and effectively at scale. The benefits and business impact of a Semantic Layer can be organized into 3 categories: Speed of Insight Creation; Scale of Enterprise Insights Program; Savings in Data Infrastructure Costs:
Speed
- Accelerating time to insights
- Accelerating data preparation time
- Accelerating time to analytics model creation
Scale
- Expanding the number of users for self-service insights creation
- Expanding the number of data sources available
- Enabling metric definition and usage consistency
Cost Savings
- Improving ROI
- Reducing Cost
- Improving Efficiency
Quantifying the Business Impact of a Semantic Layer
The purpose of this research was to understand the business impact of using a Semantic Layer to address the “last mile” of data analytics. Survey questions were designed around three value drivers associated with using a Semantic Layer: speed to actionable insights, scale of actionable insights, and cost savings and reduction.
Additionally, the survey sought responses from qualified specialists including Business Intelligence and Analytics Developers, Data Analysts, Data Scientists, Machine Learning (ML) engineers, Vice Presidents, and Chief Data Officers (CDO) who have used a Semantic Layer for carrying out AI and BI activities.
The survey was launched on April 10, 2022, and 260 people accessed it over the ensuing three weeks. Of these, 108 were validated as being qualified based on company size, role, and industry sector.
Here are the key results:
Speed to actionable insights
Respondents noted an overall 4.4x improvement in time-to-insight using a Semantic Layer. This broke down into a 4.2x reduction in data preparation time, 4.6x reduction in analytics deployment time, and 4.5x improvement in time to generate insights.
Respondents noted that a typical project took approximately 903 hours, while the same project using a semantic layer would require only 484 hours, representing a 46% reduction in the level of effort required. The results further break down the time savings for each type of activity involved in a typical data project, including infrastructure (54% reduction in time required), data engineering (40% reduction), modeling (44%), consumption (53%), and deriving insights (40%).
Scale of actionable insights
Survey results showed a 4.4x average increase in scale. This broke down into a 4.0x improvement in metrics consistency, 4.8x increase in the number of data sources, and 4.5x improvement in the number of self-service users.
Cost Savings and Reduction
Survey results showed that using a semantic layer reduces costs across the board for every activity around data and analytics. For example, for a typical insights creation activity, the effort to create insights is reduced from 550 hours to 300 hours, representing a 45% reduction. Meanwhile, the typical time spent on data preparation without a semantic layer was 350 hours; with a semantic layer, this time dropped by 18% to an average of 287 hours.
Qualitative Results and Further Reading
In addition to the 17 quantitative questions on the survey, an open-ended question asked respondents to describe their experience using a Semantic Layer. Here are two representative answers:
“For a data scientist, a Semantic Layer simplifies data preparation and feature creation with no/low code feature design. This enables the governed exploration of model generated insights, thereby accelerating the time to value for common and critical business processes in the enterprise.”
Chief Data Scientist, Siemens
“As organizations have started to concentrate on data literacy, they are planning to follow more mature models like data mesh, data fabric, or hub & spoke model. And for these models to be successful organization-wide, the Semantic Layer plays a crucial role to provide an abstract layer which can harmonize the taxonomies and provide a central hub which is aligned with data governance and data catalog.”
Director, Data Architecture & Data Engineering, Loblaw Companies
Furthermore, Megan Brown, Director of the Global Center of Excellence for Analytics and Data Science, Starbucks, wrote a conclusion describing the goals Starbucks is addressing with its data programs and how a Semantic Layer helps achieve them.
Read the full report to learn more about the impact a semantic layer has on delivering AI and BI at scale: The Business Impact of Using a Semantic Layer for AI & BI.
—
This article was written by Prashanth Southekal, the Managing Principal of DBP Institute (www.dbp institute.com), a data and analytics consulting, research, and education firm. He is a Consultant, Author, and Professor. He has worked and consulted for over 80 organizations including P&G, GE, Shell, Apple, and SAP. He is the inventor of DEAR Model, a systematic and structured approach for data-driven decision-making. Dr. Southekal is the author of two books – “Data for Business Performance” and “Analytics Best Practices” – and writes regularly on data, analytics, and machine learning in Forbes, FP&A Trends, and CFO.University. Apart from his consulting pursuits, he has trained over 3,000 professionals worldwide in Data and Analytics. Dr. Southekal is also an Adjunct Professor of Data and Analytics at IE Business School (Madrid, Spain) and CDO Magazine included him in the top 75 global academic data leaders of 2022. He holds a Ph.D. from ESC Lille (FR) and an MBA from Kellogg School of Management (U.S.). He lives in Calgary, Canada with his wife, two children, and a high-energy Goldendoodle dog. Outside work, he loves juggling and cricket.
ANALYST REPORT