February 1, 2024
From Data Products to Analytics Products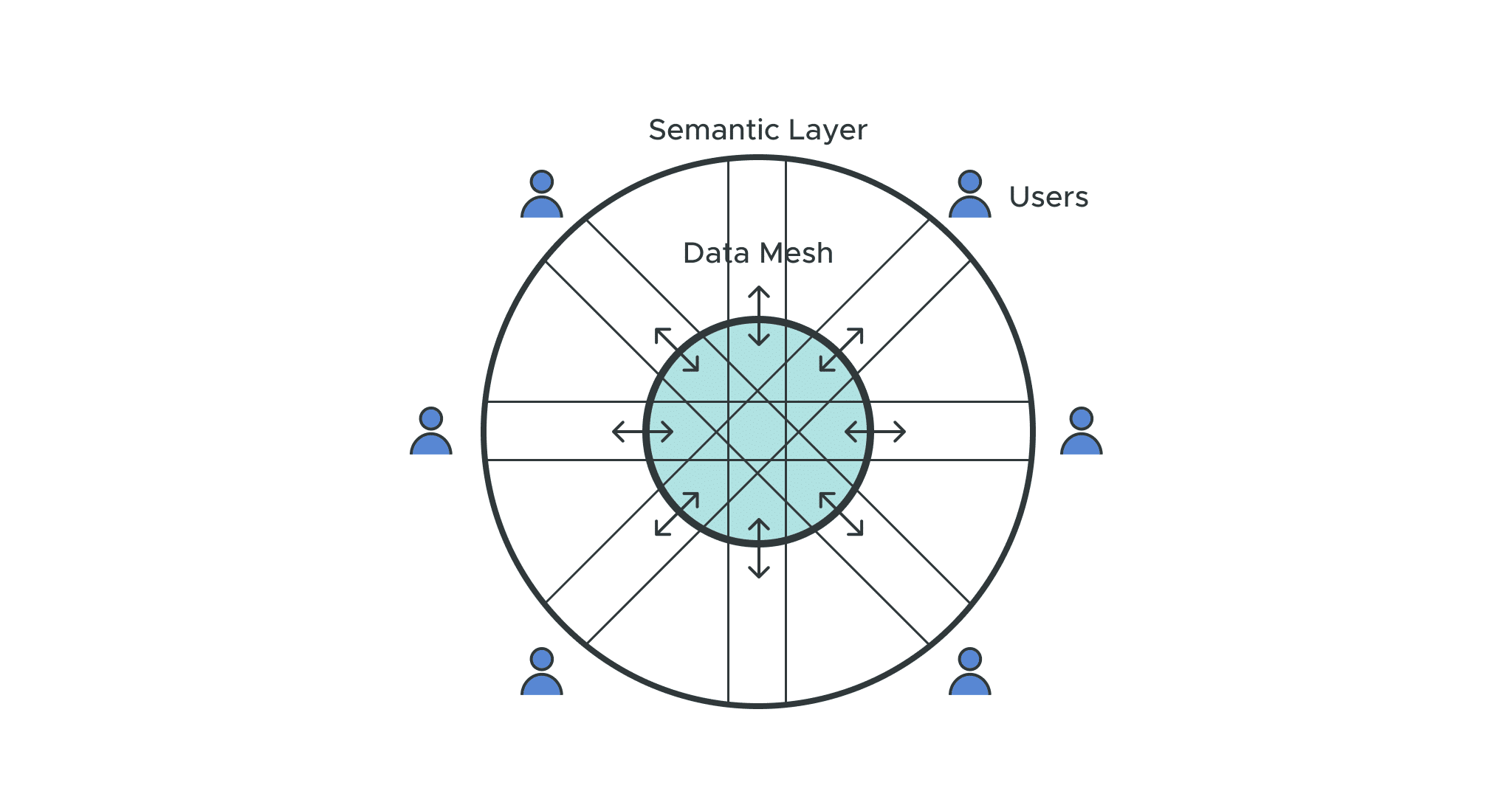
Today’s leading businesses prioritize data mesh. The modern data mesh craze ultimately came about to streamline data democratization – that is, making the right data available to the right people at the right time. Democratization is a huge departure from the data management practices of the past, which relied solely on data professionals to manage and analyze data — not its users.
But adopting modern data democratization requires modern processes and technologies. That’s why so many businesses have started considering a data mesh approach. Data mesh promises a road to data democratization through increased flexibility and agility, enhanced data governance, and the abstraction of technical complexities.
Data mesh is still a relatively new concept. While its beginnings can be tracked to 2019, its popularity has especially grown over the past two years. Google Trends reported around a 300% growth in search volume over the last 18 months. As the pressure to better leverage data assets in order to stay competitive, this widespread interest in data mesh will only continue to grow.
To better understand how can organizations embrace and unlock the data-democratizing power of data mesh let’s first address the nuts and bolts of what it is.
The Definition of “Data Mesh”
As a relatively new term and concept, it’s hard to find a generally agreed-upon definition of data mesh. For starters, it’s not the same as data fabric. “Data fabric” and “data mesh” often compete for mindshare, but Gartner defined the two as distinctly different in late 2021, stating that:
“A data fabric is the utilization of multiple existing technologies in combination to enable a metadata-driven implementation and augmented orchestration design. A data mesh is a solution architecture that can guide design within a technology-agnostic framework.”
To make matters more confusing, we see different segments of the data and analytics market each putting its own spin on the concept of data mesh. Since there isn’t a singular definition yet, let’s cover a few of the different ways that organizations choose to describe and operationalize the data mesh.
Varying definitions of data mesh from different layers of the modern data stack:
- Data Virtualization companies tend to define data mesh as a virtualization process, as in creating something that looks similar to data federation. In practice, this means different business units build analytics programs on physically disparate data sources and then centrally manage a virtualization solution that enables queries across hybrid and multiple cloud infrastructures.
- Data Governance companies, including data catalog solution providers, focus on the governance perspective. This definition of data mesh emphasizes the need to facilitate discoverability and interoperability. It’s all about giving ownership to decentralized workgroups and empowering each of them to leverage data and analytics building blocks. This way, each business unit can build its own data products on an as-needed basis.
- Data Integration and Transformation providers focus on transformation. This definition emphasizes techniques for managing the decentralization of data and analytics engineering. The goal here is to enable decentralized workgroups to manage data movement and preparation for their own analytics products.
AtScale’s Definition of Data Mesh
While the various flavors of data mesh definition are broadly consistent, they all tend to emphasize elements justifying the need for their particular solutions. So it’s our turn, as the leading provider of an independent semantic layer for data and analytics.
We see the semantic layer as an enabling technology for a data mesh strategy. Here are three of the key enabling capabilities of a semantic layer that are also fundamental to data mesh success:
- It manages the translation of analytics-ready to business-ready data by enabling your data to speak the language of your business.
- It simplifies the creation of new business-ready views with pre-built, composable building blocks.
- It is the logical place to apply governance policies that form the guardrails on data usage, ensuring consistency, compliance, and trust.
AtScale specifically ascribes to the definition of data mesh that emphasizes a hub-and-spoke analytics program: centralized governance of data assets, infrastructure, and access with decentralized data product design and creation.
Using this definition, the goals of a data mesh become:
- Defining data domains and aligning with business domains
- Combining data domains with business context to create data products
- Registering data products and making them available for re-use based on business needs
- Creating the data mesh tissue by connecting the data domains via conform dimensions
How Does an Organization Adopt Data Mesh?
The level of change that an organization must make, in order to adopt data mesh, depends on its relative level of data and analytics maturity. In many cases, the organizational and infrastructure groundwork for data mesh has already been laid. For instance, centralizing data assets on a modern cloud platform is a prerequisite. Likewise, the capability to transform and model data assets for delivering analytics-ready data is fundamental. For organizations that already have a robust data and analytics infrastructure, there are four basic steps needed to adopt data mesh.
1. Aligning data domains to business domains
Data mesh relies on the notion that there is a specific business group (i.e. business domain) that’s the logical “owner” of a set of data assets (i.e. a data domain). Aligning data domains to business domains sets up the basic rules of which business unit bears responsibility for curating and augmenting which data sets. Without this alignment, an organization will inevitably see conflicts between groups as they assign different significance to the same data. Without clear ownership, no one is responsible for defining the proper usage of a given data set.
2. Treating data as a product
Data as a product closely aligns with the definition of data mesh. The idea is that the business owners of a given data domain take responsibility for augmenting raw data with business context, in order to make the data useful to the broadest set of users. Business context may include basic transformations of data to make it more workable for an analytics use case. It may also include augmenting historical data with aggregation logic and hierarchical dimensional logic to support drill-up / drill-down analytics. Data products need to be created with user-friendliness in mind, laser-focused on business users’ needs. So data as a product must include the creation of business-oriented views of data that support self-service analysis.
3. Embracing Composable Analytics and Shareability
Treating data as a product obviously means positioning data assets for direct analysis by data consumers. But it also means positioning assets for reuse in more complex applications. Domain owners should be registering data products within a catalog and making them available for reuse by other groups in the organization. Some of the most valuable data analytics inherently require blending data across multiple domains. A few examples include…
- Profitability analysis blending revenue data from a CRM system with cost data from financials
- Employee productivity blending HR data with financials
- Prediction models blending sales data with 3rd party data on the economy.
Most importantly, data products need to be discoverable and accessible by different work groups around the organization.
4. Building a Connective Tissue
Combining multiple data products managed by different business domains requires a common connective tissue. “Conformed dimensions” are governed by hierarchical master data that define how data can be aggregated. Time is the most common conformed dimension, establishing day-week-month-quarter-year logic that can be used in any data set. Other common master data concepts that often need a conformed dimension include Product, Geography, Employee, and Customer. Conformed dimensions can be used by any work group to combine different data assets into a new data product. Leveraging centrally-governed dimensions eliminates rework and eliminates the chance for mistakes.
What Does Data Mesh Have to Do With a Semantic Layer?
The balance between maintaining centralized governance while enabling the decentralized creation of data products is easier said than done. That’s where AtScale’s semantic layer strategy comes in, establishing a “common language” for all business units.
One of the most important components to data mesh success is making your data business-ready. To find out more about what that transformation looks like, check out the next post in our data mesh series or read the entire data mesh series in the white paper, “The Principles of Data Mesh and How a Semantic Layer Brings Data Mesh to Life“.
ANALYST REPORT