October 9, 2019
How Digital Transformation Enables Big Data Analytics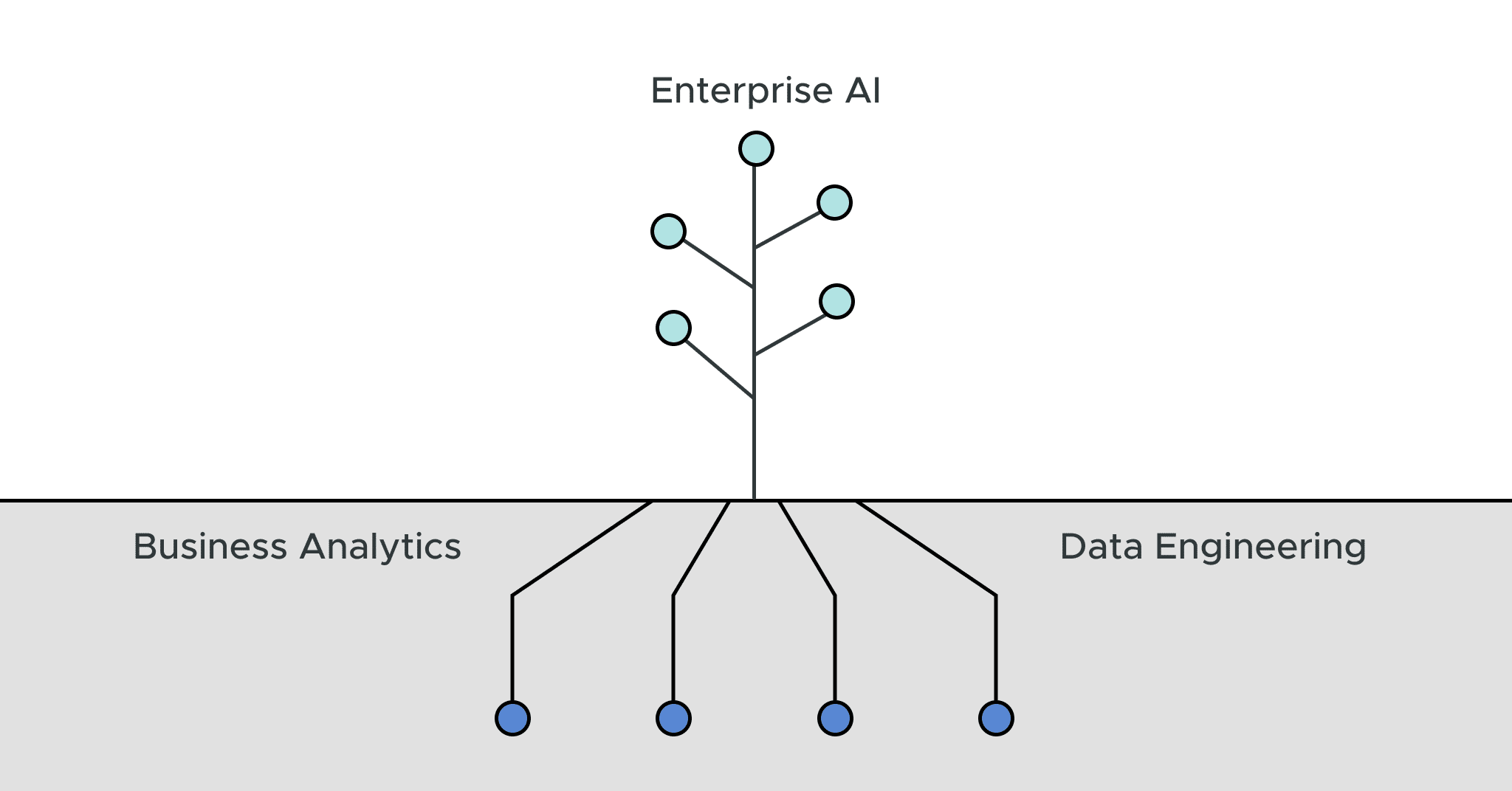
There is a massive global investment in enterprise AI. While the potential for AI-driven value is huge, organizations are struggling with a common set of challenges to realizing this potential: Lack of data scientists, difficulty in moving more models to production, and the complexity and disaggregation of tech stacks. But the most common failure pattern to scaling an enterprise AI strategy is starting without a strong foundation in data analytics.
AI and ML can only exist on top of a foundation built on solid data and analytics
Succeeding in Enterprise AI Strategy Requires Strength in Data Engineering and Analytics
First and foremost: if you aren’t good at data engineering and business analytics, you won’t be good at AI/ML. In my experience, management teams often assume they can leapfrog best practices for basic data analytics by directly adopting advanced ML/AI technologies.
Heed these words: Companies who rush into AI before mastering the fundamentals will end up paralyzed. Not only do you need to be good at data engineering and business analytics, but you also need to embrace advanced automation. Lack of automation means people manually use the ‘bug-entry device’ (a.k.a. the keyboard) to process pipelines, do ETL, move data, and create downstream assets which cannot scale. All of these activities can and should be automated to avoid outcomes epitomized by the infamous AI phrase: “Garbage in, garbage out.”
AI requires a highly sophisticated application of data to draw correlations and operate properly. If you do not have technology that creates and maintains data pipelines and basic data engineering, your ability to solve problems with AI will suffer for it.
Do Not Undervalue Data
The best algorithms in the world cannot overcome bad data. When it comes to improving data quality, it’s key for you to:
- Identify the real data that can impact your business
- Focus on that data and understand how to use it
- Contextualize your data so you know how it relates to your enterprise
Remember, you don’t necessarily need to know WHY that data is important right now. Have you got data that is proprietary or impossible for your competitors to gather? Comb over that and figure out how it’s relevant first.
The Two Key Frameworks for Enterprise AI Strategy
AI done right will fundamentally change your business. In these highly dynamic situations, there are two frameworks I merge for success:
- The OODA Loop
- The golden triangle of people, process, and tools
How to Manifest the OODA Loop
Start small. Execute pilot projects to test your digital maturity and stress your data pipelines to both find opportunities for improvement as well as gain momentum.
What is the OODA Loop?
The OODA loop is Observe, Orient, Decide and Act. The goal of the OODA loop is to compress cycle time to maximize feedback and learning. In the shifting field of ML and AI, the OODA loop is more critical than ever.
Observe and Orient
- Define the nature of the challenge
- Contextualize and chart a map of the territory
- Identify obstacles
- Recognize opportunities
Decide and Act
- Actions to carry out
- Guiding policy
- Resource allocation
- Coordination to create impact
- Harvesting opportunities
The Golden Triangle of an Enterprise AI Strategy
Over my many years of running startup engineering teams, I’ve relied on the golden framework to help get me oriented in a new unknown situation.
- People. Always start with people. Identify your key role players in the AI space and then understand what they bring to the table. After you take stock of what you have, you’ll have a map of what you need.
- Process. Process is where the Golden Triangle overlaps with the OODA Loop. Ensure you’ve identified desired outcomes and reviewed the process and goals with the right people.
- Technology. Consult your team and review the available AI technology. Chances are you will be deploying on one of the big cloud platforms: AWS, Microsoft (Azure), or GCP. Here, you’ll have to consider the specific capabilities of your users and their technical backgrounds. The last thing you want is a tech that’s not compatible. Fit your tech stack to your people after defining your major business problems and solution requirements.
You can drive AI outcomes while paying mind to data analytics trends. Make sure your people know they are building a lab AND a factory. Use this opportunity to improve all the elements of the golden triangle:
- Remove the people-based skepticism that exists when making a huge shift in how you operate. AI will scare people and there has been more than enough hyperbole to make people wonder what’s real.
- Improve the overall process of collecting, cleansing, and serving data to consumers of all types. It may be that AI is the desired “goal,” however, if you can improve your BI at the same time, that has an immediate impact.
- Tools and tech are more important now than ever. Test before you invest.
Focus on Outcomes, Not the Path to Them
Avoid the natural human nature to understand all the details of why your AI or ML is making certain conclusions and outcomes. Your team doesn’t necessarily need to know how a Convolutional Neural Network (CNN) works in great detail, but they do need to know what class of problems have appropriate applications.
Take advantage of the G-MAFIA (Google, Microsoft, Amazon, Facebook, Intel, Apple) and leverage their pure research and basic science. as a lot of their work is either open sourced or documented for your consumption. Let them figure out the details. Build your in-house AI team and help them make an initial bet on which of the AI vendors make the most sense for your business.
Avoid A Strategy Tax
What is a strategy tax? Sometimes products developed inside a company have to accept constraints that undermine their competitiveness, or might displease its user base, in order to further the cause of another product. That’s a strategy tax. To avoid those pitfalls when creating an enterprise AI strategy, remember to:
Be Specific: Don’t just state that you want to be a leader in the AI space for your business problem. Design the roadmap that will get you there. Fuzzy strategic objects are everywhere right now. Understand the key metrics you want to improve and apply focus and resources there.
Coordinate: Lack of coordination will almost always ensure that your AI/ML initiatives will fail. AI/ML benefits from coordination across a variety of disciplines. It truly takes a village to execute, so the sooner you get your teams on the same page, the better.
Implement Continuous AI Education
Ensure that your AI group has a mandate for education across all lines of business. Great ideas will emerge from within deep pockets and different groups. Remind people that AI is important and that you are looking for opportunities to boost employee engagement and drive innovation.
Besides, who doesn’t want to learn about AI/ML these days? It’s not just about internally sourced content. You should give your people the incentive to learn online and augment with your specific insight. Train at all levels. Remember, data democratization includes all users, not just the math and computer whizzes.
AI is Made of and for People
AI will change your non-AI-focused employees. People will need to evolve their skills and duties through AI training. That training helps democratize data for all users in the long run.
AI can be held back by a culture that doesn’t recognize need, a lack of data, and a shortage of talent. Keeping your team in the loop on the decision-making process and enlisting them to help solve problems will help you get the right buy-in.
Remember, AI is a means, not an end. “Do you have an AI strategy?” is like asking “Do you have an app server strategy?” Creating an environment for innovation, the perennial top concern for CEOs, is more important in the age of AI than ever before. Real innovation brings failure and requires strong-willed commitment.
The Realities of Regulation and Ethics in AI
There’s a growing trend in shaping AI and ML to be empathetic and explainable. Can we trust AI? What if it has a bias or makes weird decisions? Who in your organization is responsible? The morality of AI is a huge subject that will directly affect how successful you become with the technology.
There will be situations where the AI will need to make a “lesser of two evils” decision. No doubt all industries can benefit greatly from machine-augmented intelligence, but when does its involvement step over the line? AI can and does craft offers that can be very attractive to humans, and we can drive behaviors of consumption that may not be appropriate. It’s key for your business to consider these ethical questions while developing its enterprise AI strategy.
Most importantly, implementing an enterprise AI strategy is not a set-it-and-forget-it pursuit. It is a continuous journey that requires education, communication, and a strong foundation in data analytics.
Building a strong data analytics program that enables strategic AI implementation can be tricky to navigate. Learn how AtScale provides the right pathway to democratizing data for all.
***
This post was originally published on August 20, 2019 by Matt Baird.
RESEARCH REPORT