April 21, 2021
The Data & Analytics Maturity Model: What is It and Where Does Your Team Stand?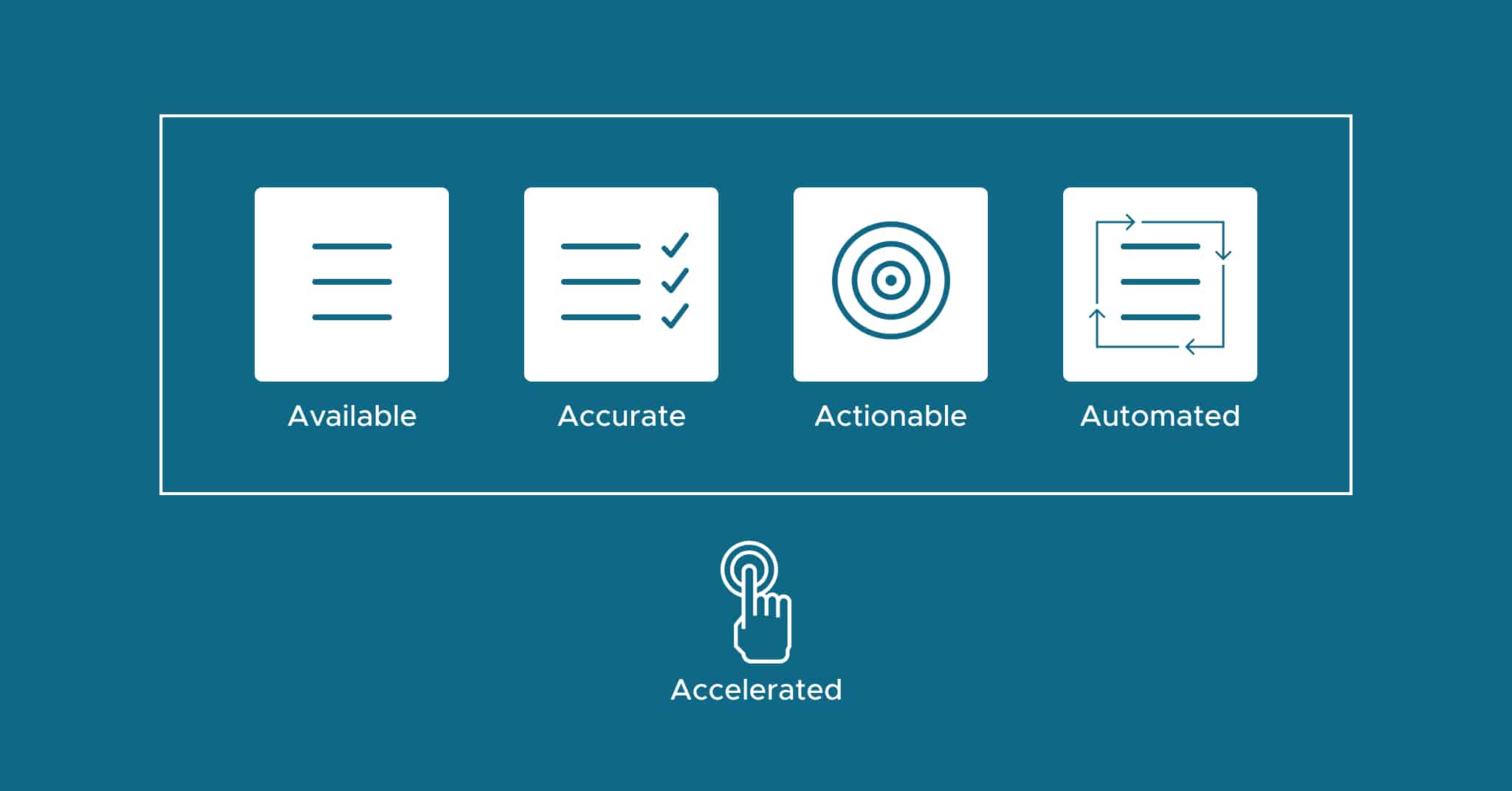
Many companies have difficulties developing a roadmap for data, insights, and analytics. This blog stresses the importance of having an enterprise data strategy as the guidepost for articulating an effective set of use cases and a clear roadmap that scales the impact of data, insights, and analytics. Data is the fuel for addressing key business questions. Aligning business questions to answers available from data sources establishes the expected value from delivering actionable insights scaled over time as a roadmap aligned to current and intended capabilities maturity – accelerated by AtScale.
A Smarter, Faster Path to Actionable Business Intelligence
As companies move to modern cloud platforms to enable the improved use of data, insights and analytics, many realize that the “last mile” of preparing, analyzing and publishing insights – the data consumption layer for business intelligence – takes too long to create, refresh and refine. Key to realizing improved speeds to actionable insights is developing an effective data source strategy and standards informing data acquisition, preparation, integration and publishing for business insights creation.
Enabling the Five (5) A’s of Data
It’s important to establish standards of performance for how Data, Insights and Analytics (DIA) are created and consumed. There are many criteria to consider; let’s start with some essential criteria, referring to the list as the core five (5) A’s of data: Availability, Accuracy, Actionable, Automated, plus the fifth A: Accelerated, reflecting improved speed and scale!
The Five A’s of Data – Available, Accurate, Actionable, Automated, Accelerated
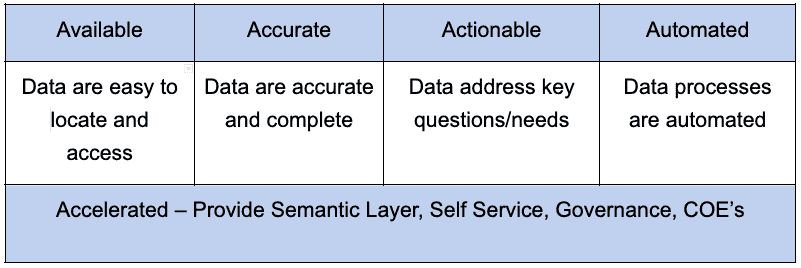
- Availability – Access to data is an obvious requirement for being smarter, faster. In this context it’s important to define the criteria for what availability means, such as typical lead time to make a data source available, location of data, lineage of data, method of direct access, structure of data: dimensions, attributes, metrics, applications used to access, refresh rate and ownership.
- Accuracy – Another core requirement is certainly to be using data that is accurate. Accuracy is a key piece of the larger subject of data quality. The important aspect to stress is that business ownership, governance, automated processes and reporting should be established for ensuring that data is properly extracted, transformed and loaded (ETL) from the source to the data lake and from the data lake to the data consumption layer (where data is reported and analyzed), and that a standard set of data quality metrics should be applied to track lineage, measure completeness, frequencies, anomalies and change, including using statistical methods to automate detection, reporting and remediation. Further, analytics governance should also review accuracy results and plans. Finally, data quality is everyone’s responsibility: business owners of the data and users need to own the quality of their data, along with the technical team.
- Actionability – It’s important to establish a framework for evaluating the relevance, utilization and impact of data, insights and analytics, both qualitatively and quantitatively. This is an area where many companies struggle – the key is to establish formal measures to track, including reporting progress periodically, and setting annual goals. It’s important for business leaders to take responsibility for the impact they generate from using data, both from a benefit driver perspective, but also from a usage perspective.
- Automated – The key to becoming smarter, faster is having all data movement, transformation and consumption activities automated. This includes automated data pipelines to the data lake from source systems, data transformation within the data lake and data structuring for consumption for business intelligence reporting and analysis. It is important to use modern tools for data movement, and it is recommended to set up a data integration COE to manage the many data movement needs that are required to support the modern data platforms as well as the modern architectures using application protocol interfaces (API) for data messaging and movement, including for microservices.
- Accelerated – To turbo charge the path to smarter, faster, there are a couple of important capabilities to consider that can accelerate the speed and effectiveness of data, insights and analytics by offering and supporting self-service tools for analytics and data scientists, deploying a semantic layer to speed data access, accuracy and insights automation and implementing data governance. Deploying a semantic layer can also significantly reduce the need for data replication and movement – enabling multiple data sources to be rapidly integrated and aggregated from multiple sources for business intelligence (and analytics) without changing the underlying data values or structure.
Creating an Enterprise Data Source Strategy
Often overlooked is the importance of establishing a strategy and plan for how data sources are acquired and utilized across the enterprise. Organizations earlier in their development tend to have many data sources in disparate locations and conditions of readiness for insights delivery. Further, data science teams depend on having rapid access to a multitude of data sources to develop effective analytical models. Finally, moving data for analysis to the enterprise data lake should be done with prioritization in mind so that the cost to set up automated data pipelines, data structures and reporting from newly integrated data sets can be aligned to the agreed-upon use cases.
An effective data source strategy is crucial to ensuring that the improvement in both breadth and depth of insights is aligned to the data, and that all data sources be seen as being available for enterprise use, albeit with appropriate considerations for data usage, privacy and access. An effective, aligned data source strategy typically consists of the following:
- Identify use cases and data source subjects (e.g. sales, pricing)
- List key questions that are to be addressed for each use case
- Explain how data source(s) address the questions – key features
- Determine data source availability and readiness
- Establish roadmap for data source acquisition and distribution
Data, Insights and Analytics – Data Source Strategy Key Elements
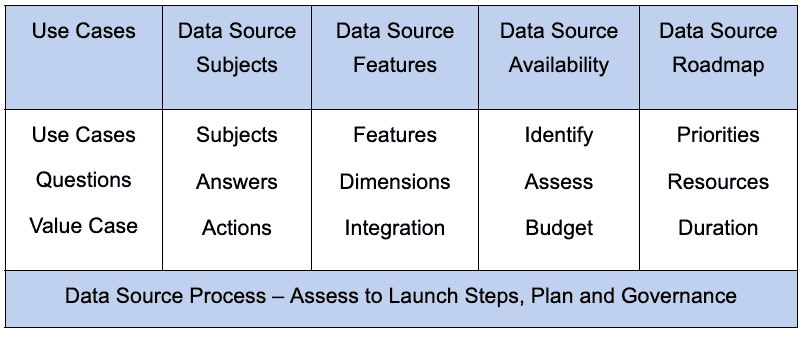
Delivering the Five A’s of Data – The AtScale Advantage
AtScale has created a solution that automates and simplifies the process of making data available for business intelligence, including to BI tools like Tableau, Power BI and Excel. Thus, all that is necessary is for one user, whether a data analyst, data scientist or data engineer, to visually select the dimensions, hierarchies and attributes or features desired, and AtScale automatically creates the data extract for loading into the BI tool immediately. Further, given that many BI tools cannot handle atomic-level data, AtScale automatically optimizes the aggregation of the data to ensure that reports generated within BI tools are effective. Finally, because AtScale automates the entire process with no coding required, self-service BI is enabled with point and click simplicity, including supporting self-service data refinement, sharing / reuse and governance of data access, preparation and consumption.
The Path to Rapid Insights from New Data Sources – AtScale Semantic Layer
The key to this new solution for business intelligence automation is the semantic layer . AtScale has created the ability to visually view data, including attributes, metrics and features in dimensional form, clean it, edit it, refine it by adding additional features, and have it automatically extracted and made available to any BI tool, whether Tableau, Power BI or Excel. This only requires one resource who understands the data and how it is to be analyzed, which eliminates complexity and the need for a lot of resources. This also eliminates multiple data hand-offs, manual coding, risk of duplicate extracts and suboptimal query performance.
Want to Accelerate Your Business Intelligence? Contact AtScale Today.
ANALYST REPORT