March 6, 2019
TECH TALK: AtScale 6.0 brings Universal Semantic Layer Benefits to Google Cloud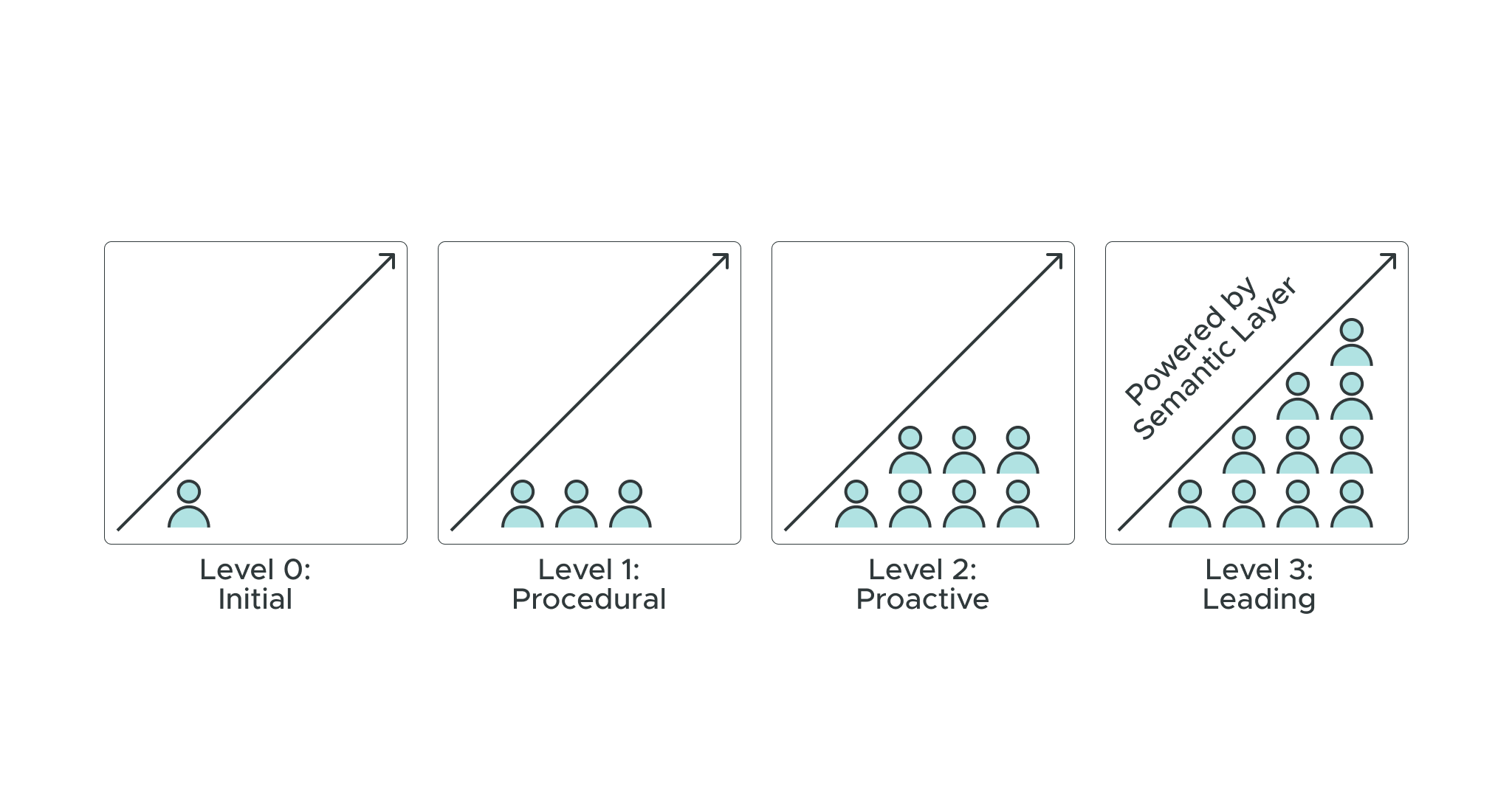
The number of data analysts at your organization is a limiting factor that determines how many stakeholders are able to make data-driven decisions. To make better-informed decisions, organizations need to increase the number of people with the tools and expertise to interpret the troves of data flowing into their systems.
In Module 7 of our Data & Analytics Maturity Workshop Series, we discuss the four levels of data analytics maturity:
- Level 0: Initial
- Level 1: Procedural
- Level 2: Proactive
- Level 3: Leading
We’ll run through the personas that engage with data as organizations mature and the power of a semantic layer to help accelerate this progression.
Level 0: The Initial Phase of Data and Analytics Maturity
At Level 0, only one persona — the data analyst — is involved with analyzing and interpreting data to answer questions across an organization. The data analyst is charged with wrangling data and applying their technical expertise to make sense of it. They typically have specialized skill sets, including knowledge of SQL coding and the architecture of data assets within the warehouse or lakehouse.
There is nothing like a power data analyst. The problem with relying on these specialized players to interpret data is that it’s not scalable. It’s way too expensive for organizations to get enough of these specialized resources. Furthermore, even SQL experts take time to extract business-ready analytics to support real-time decision making.. This limits the scope of who can leverage data to make decisions, ultimately limiting the data insights within the organization.
Level 1: The Procedural Level of Data and Analytics Maturity
Graduating from Level 0 to Level 1 represents significant progress. Rather than relying solely on data analysts to make data-informed decisions, organizations expand their stable of data consumers to include a broader set of personas including business analysts and data scientists.
At this tier of the maturity, business analysts are using tools like Excel or Tableau to explore data and find out what’s going on across the organization. Additionally, at this level, data scientists may explore data assets with python and leverage AIML platforms to augment their capabilities.. At this stage, there are far more people interacting with data than at Level 0, but there’s still a reasonably high level of expertise required to interact with data and generate actionable insights.
Level 2: The Proactive Stage of Data and Analytics Maturity
At the Proactive stage of maturity, organizations begin to develop and leverage a new data analytics persona: the citizen data scientist or citizen data analyst. These individuals combine their understanding of the business with practical data skills to integrate data-driven insight creation into daily business processes. This also enables teams to begin leveraging predictive analysis to move beyond a pure retrospective analysis of historical data.
Prior to Level 2, data analysis is largely retroactive. At the initial and procedural levels, organizations are looking back at what has already happened at their organization, analyzing existing data on recorded events, and making decisions for the future based on this historical data.
At this new level of maturity, organizations can create forward-looking decision support systems that allow business decision makers to get more accurate, actionable answers to their questions. Furthermore, these citizen analysts and scientists should be able to more freely interact with data assets without the need to code SQL,
Level 3: Data and Analytics Leaders
Most organizations have not progressed to this level of maturity. At a Level 3 organization, data analyst is no longer a job title. That’s because data analysis has become a function of every role across the company.
This enables an environment where everyone is sharing information and interpreting data to make better-informed decisions. As a result, the data literate persona at a Level 3 company is “everyone”.
The Power of the Semantic Layer
Leveraging a semantic layer that stands independent from BI tools and cloud data platforms
centralizes data governance and control while enabling de-centralized analytics consumption and data product creation.
This exposes data consumers to a business-oriented view of data and analytics, and empowers a broader segment of your organization to interpret descriptive, predictive, and prescriptive data.
Increasing data literacy across your organization ensures all stakeholders can make better-informed, data-driven decisions.
With a semantic layer, data consumers can instantly apply insights without having the training and expertise to understand the physical structure of the data itself. Furthermore, organizations can more easily interact with predictive analytics that create powerful tools for being more prepared and proactive in ever-changing competitive business environments .
Fostering a culture of self-service and data literacy enables organizations to take their data maturity model to the next level. With AtScale, AI-generated insights can reach broader audiences, eliminating the need for discrete data analysts and inviting all stakeholders to engage in data analysis.
Watch the full video module for this topic as part of our Data & Analytics Maturity Workshop Series.
ANALYST REPORT