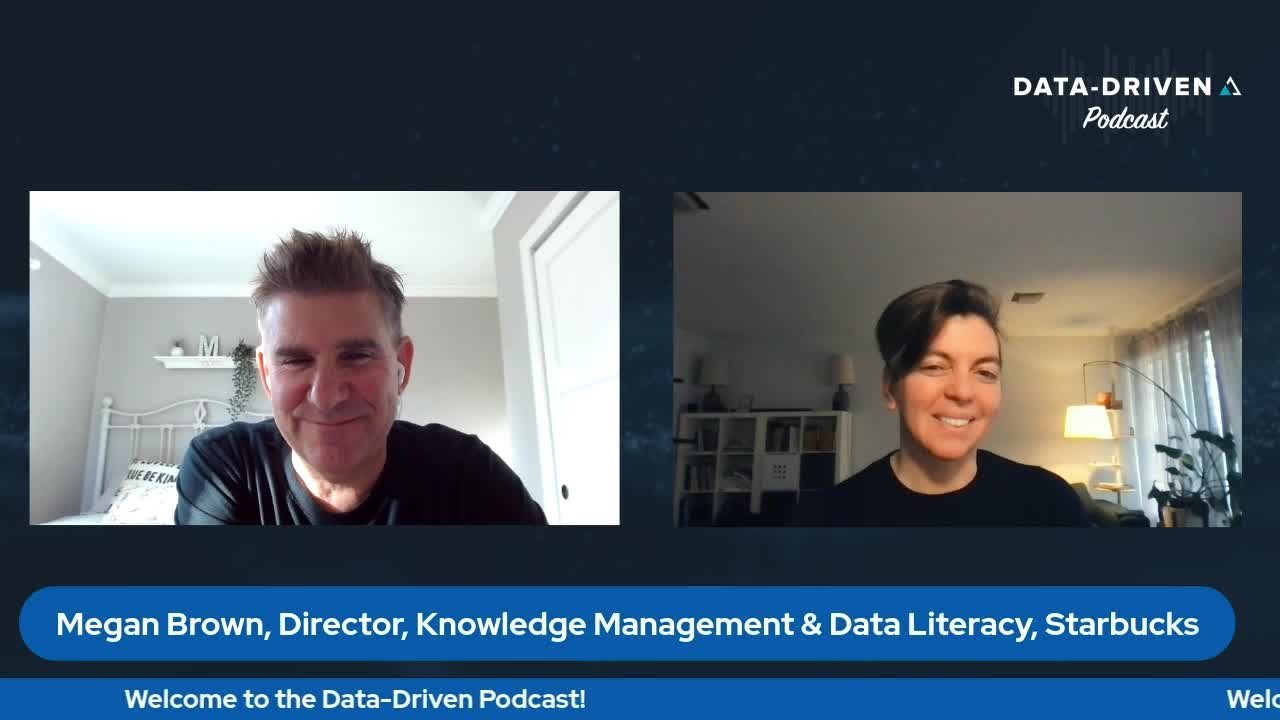
Listen to Megan Brown talk about building a knowledge management and data literacy program and experience launching “the Wayfinder” that offers Starbucks employees a search engine with links to self-service dashboards supported by a library of training material and documentation to use the data and metrics to make smarter decisions regardless of their role.
Transcript
Dave Mariani: Hi everyone. And welcome to AtScale’s Data-Driven Podcast. And today our special guest is Megan Brown. She’s director of knowledge management and data literacy at Starbucks. So Megan, welcome to the podcast.
Megan Brown: Thanks. I’m happy to be here.
Dave Mariani: Great. Everybody knows Starbucks and everybody, and everybody knows obviously about the products you sell. But, there’s a lot of data and analytics and a lot of data-driven decision-making that goes on at Starbucks. So I’m sure the audience is going to be really excited to find out about, just what that’s all about. So, so Megan, let’s just start with, with you. Can you tell us a little bit about, you know, how you got to where you are today, and, and in the specialty and data analytics that you focused on?
Megan Brown: Sure. So I actually started my career as a fifth grade teacher. Wow. And the hardest problem I encountered was reading comprehension in a language diverse classroom. The best practices didn’t really work. So I decided, oh, you know what I’ll do. I’ll go to grad school. I learn it all. I’ll solve the problem. It’ll be great. I went to grad school and I ended up kind of at the quant bleeding edge of cognitive psychology. So the lab I was in at the time did neural network models now known as deep learning Corpus analysis now known as NLP and experimentation of course, because it’s psychology. So I did that. I hung out as an academic for a couple of years and I learned that I needed a little more collaboration and I wanted to see the stuff I was producing applied. Like I wanted to see it in action, like, you know, half a year instead of 10 years.
Megan Brown: So I followed the wave of people leaving academia after 2008 and went out to become a data scientist in people, data science. So I did data science in a couple of different domains. So people in marketing and then in the pandemic I’ve moved into leadership roles. And one of the spots I moved into was knowledge management, data literacy. And I think I did that because I was the data scientist that kept asking, why are we doing it this way? You do it that way. Wouldn’t that be better? So I got myself into trouble and that’s how I got to the director role.
Dave Mariani: Well, what’s interesting is that you sort of combined, you know, your love for teaching and academia with, you know, knowledge management in analytics. It’s like with your, with data science. So it really is like a perfect sort of mismatch of all the different skillsets. It seems like it’s a, a great match for, sort of being in charge of data literacy and knowledge management, Starbucks. I,
Megan Brown: I like to think so
Dave Mariani: It’s like, it’s like, if you had, if you could write a job description, it would be Megan Brown.
Megan Brown: It would sometimes be the science seems like a bit of a rarefied field, like, you know, the Oracle on the hill or something like that. But actually we all learned to do it. So it’s in theory, accessible to everyone. We just aren’t really invested in making it accessible to everyone.
Megan Brown: Yeah. You know, it’s like, I know we’re going to talk a lot about data literacy, but you know, data science is also pretty fascinating. There’s a lot of, you know, there’s so much focus on AI and machine learning. And I think a lot of enterprises out there are just struggling to, they know they need to be doing it. but they don’t really know how to, how to get started often or how to operationalize it and how to get beyond the science experiment and get into actually applying the learnings to the business. So what have you, what have you seen there, Megan I mean, what have you learned
Megan Brown: It’s a lot of different skill sets to operationalize data science, right? You have your data scientists too, you know, they probably go for the fanciest model. They can, to be honest, right Like I, I always had something simple and something really cool. a really cool thing. Probably can’t be put into production most of the time, you know, you need machine learning engineers and you need your software developers and you need your technical product managers. And when all of those people work together, you’re more likely to build something that can be put into production quickly and easily. I think often the things that make it into production are shockingly simple, considering the bleeding edge of data science and the bleeding edge of the field. Yeah. A lot of this stuff is pretty, I don’t know, for a data scientist pretty straight forward.
Dave Mariani: Yeah. And then there’s nothing wrong with that either. I mean, look, ultimately, you want to automate decision-making, and if you can automate decision-making with some, you know, with a simple model and a simple way to get to production, that’s really valuable. And, and you can learn from that and, and go from, and you could add complexity later, but why, you know, it’s like, so I, I really, I really liked that,
Megan Brown: You know, the 80% rule in business, right. If you’re accurate enough to support people and, you know, nudge them the way you want them to go more coffee, buy more coffee,
Dave Mariani: Pretty much,
Megan Brown: I mean, what’s, what is a 2% accuracy gain in your offers if you’re already fairly successful with your offers. So, you know, you can also build other models for other things, you know, or get that 2% gain. Right.
Dave Mariani: Yeah. And, you know, you said, like you said that, that the team is important that you have the cross-disciplines between, you know, your data scientists, your, your product managers, your, and your software engineers. I mean, is there cause organizations, typically those are separate silos. Right. So, so, so how do you, how do you bring those together as it project-based or how, how does, how does it work How does it work best for you
Megan Brown: We are, we are cross-functional so it takes a lot of good faith co building. If that makes sense, like, you really do have to trust that everyone’s invested in the project and they’re all going to look for resources for the project. And, that you’re as clear with your communication and your planning as possible. And I think, you know, things fall apart sometimes when someone isn’t as forthcoming or, or isn’t as supportive of the project, as they say they are. but most of the time that’s, that’s, that’s just table stakes. Like that’s what you need to deliver something really cool.
Dave Mariani: So is there, so who was, who project managers it, who owns it So cause you gotta have some somebody driving it. Right. So how does that work if it’s cost, if it’s cross-functional,
Megan Brown: If it’s cross-functional, I think it’s a very, it’s a dance because everyone wants the glory for AIML and the, and the same thing’s true of data literacy. Everyone wants the glory for data literacy, but you’re working very cross-functionally. so you kind of have to carve off pieces that each senior leader can own and can claim credit for. but hopefully in the long run, the whole endeavor can be like, doesn’t need a single threaded leader from a single org because it’s not true.
Dave Mariani: That’s super interesting when I was at, at clout. you know, we, we determined your, your social, your social score, which is kind of crazy bad thing, but, your cloud score to see how, how influential you were. And, and there was a lot of data science didn’t coming up with the score. and so we built what we called feature teams. So we had it, we had a separate group group of engineers for data science versus this to software engineers, but we would scrum together in a feature team. So for a project that would be our, our focus that would be a delivery of that feature. And it would include data science and they would just, you know, code and build the product together and ship it together. but, that was, that was, that was a small company. So it’s, I, I imagine it’s a little more difficult than a luxury company, like, like, like a Starbucks.
Megan Brown: It is, I think Starbucks is known for being very relationship and community oriented, even, you know, in the Starbucks support center, which is what we call our corporate. Is it an office anymore I don’t have what our corporate
Dave Mariani: Yeah. Is it an office is right. Yeah, that’s crazy.
Megan Brown: I think the relationships really do matter it Starbucks. And so the better your relationships, the more easier that kind of cross-functional work will be, you know, I think organizations always change and reshuffle to like align the interests of things. so that happens every so often at Starbucks to try and get things closer together so that perhaps eventually there is a single threaded executive in charge of AIML, but, you know, not yet.
Dave Mariani: Very interesting. So let’s shift gears a little bit and this let’s talk about data literacy. so, you know, you’ve, you’ve, you’ve done a lot on the topic. we’ve done, we’ve done some, a great webinar together, with Mariska, from bowl.com and the like, and that’s just for the audience, you know, how would you define what data literacy is and why, why is it important Why are people talking about it
Megan Brown: Yeah, I think, I mean, at its most simple, it’s really, can you use data to do your job, right And that’s, that’s a really, really simple definition I can give like, you know, a 20 word, long definition, but in an organization, can you find the data Can you access the data Can you get the data Can you manipulate the data And can you interpret the data and put it into persuasive argument for your costs Right.
Dave Mariani: And Megan, this is not, this is not just for a data analyst, right This is for anyone in the organization, right. Who is making decisions that’s right.
Megan Brown: and you know, in my mind, I’m a, I’m an idealist, right I think everyone at the company should be able to do those things to some extent with their data in their field. Right. the tools are getting closer and closer to being where we need them to be for people to, you know, self-serve is kind of like an old term for this democratization, for people to be able to access the data, know what exists and everything. I think there’s still a bit of fear and I th I’ve seen this at a couple of different places, so it’s not like, you know, Starbucks, isn’t scared of data, but fear out there, but at the individual level about, am I doing this the right way Do I have the right data And maybe I should just go have someone check this. Right.
Dave Mariani: lack of trust. It sounds like a lack of trust in their own abilities or the lack of trust in the data that they’re dealing with.
Megan Brown: Yeah. I think there’s a part of that lack of trust is it’s just not super transparent. The whole process of like, you know, the entry into the point of sale or the app all the way through to this dashboard, like what’s happening to the data in there are these, there is this dashboard from those data. and anyone who’s worked with data knows there’s a lot of nuance to that whole pathway, right
Dave Mariani: Yeah. A lot of people touched that data along the way.
Megan Brown: Yeah. And often there’s like three or four different data sources at a company that may not be in agreement all the time that look like they’re the same. So, there’s a few different things that, you know, the technologists kind of have to think about and clean up a little bit. We have to tell our story about how data gets to beat in that dashboard. We have to have an understanding of what dashboards were built by, by people who are using trusted data. And then, one of the things that gets at people’s lack of confidence with data is really if they present a metric in a meeting and the senior leader says that it doesn’t look like that metric, should I think you, I think you’re wrong. One of the things that happens in that meeting to that person is that whole topic derails and people are distracted by the wrong metric rather than the message or the
Dave Mariani: Completely seen that happen so many times
Megan Brown: Yeah. That erodes trust. Right. So if they can’t say, well, I pulled it from this dashboard that has a gold star of being from the right source. And I checked my stuff with a data scientist or a decision scientist. they’re kind of, you know, really, really in an unfortunate situation.
Dave Mariani: Yeah. You know, I saw you wrote about it in, in, in one of your white papers or the one of your eBooks about, you said that, 21% of employees say they’re only 21% of the employees say they’re confident about their data literacy and their abilities. And that’s a pretty low number. and that’s people sort of admitting it, so that’s probably even lower than that. So, you know, so, so it’s, it’s, so there’s a lot of, there’s a lot of work to do there. So, you know, w why do you think it’s so low Is it because of a lack of trust because people can’t see, they don’t have visibility into where the data is coming from Is it, is it, or is it more than that
Megan Brown: I think there are a few different parts of this, right. So one of it is a lack of trust, right I think, tech tech, people, including myself, could be more transparent about, you know, what does exist in the data lake. What’s not in the data lake, why not Right. metric definitions being solid and enterprise wide is a big push for my team. And that means those metrics become trusted. Data sources are more obvious, perhaps not as obvious as I want them to be right away, but more obvious. so that number is from an Excentra study. And I think every company you go to has a very different ecosystem and a very different data emphasis. So one company might have excellent coverage of their customer data, right? And most companies have excellent coverage of their customer data. It’s, you know, where, where the profits come from, do you have to, but then assorted other areas are, are in states of occasionally disrepair.
Dave Mariani: I like that
Megan Brown: Prioritized. and, but the, in my mind, the most interesting part is really where those things come together. So if you have a couple of states of disrepair and your customer data, you really only have part of the picture. Right. So if you don’t have the whole picture and you know it, you’re not going to really like to try and sell something based only on a part of the picture. Yeah. Someone who doesn’t know, they don’t have the whole picture. We’ll totally go for it. Yeah.
Dave Mariani: And then get embarrassed in that meeting when somebody calls them out. Right. And then square one
Megan Brown: Meetings in that last meeting, just
Dave Mariani: Whoops. Check that. I think,
Megan Brown: Yeah. I think a lot of people also don’t know what data exists and that’s why knowledge management supports data literacy. yeah. They’ll have an idea that it should exist. And there’s also this idea that analytics or data science is kind of magical. Like, I’m going to ask you for this thing. You’re just going to go to the table. You’re going to pull from that table and you’re going to give me that thing. And it’s going to take 30 seconds and it’s just not true. And when they learn just how not true it is by trying to build what they want or get what they want out of the builders. it erodes their trust because they think that’s how it should work.
Dave Mariani: You know, it’s like when we talked, you know, you built some, cause you talked about people can’t find it. They can’t use it. Right. and so I have always been thinking about findability, I call it findability, but it’s, I think you can build some tools at Starbucks to help people find data. What, what, talk to us a little bit about what you’ve done to help solve the findability problem.
Megan Brown: Yeah. So analytics and insights is an org that has everyone from futurists to production data scientists in the same work. Right. So we have an array of methods. Sometimes we can talk to each other really well. And sometimes we can’t, but we’re a tech or that situated in the business. Okay. And so there’s always a strong emphasis on the relationships we have to maintain. But one of the aspects of being a relational company is that information is shared in relationships. And so as the pandemic hit relationships kind of weakened, they became more transactional in my experience, this only seems like it was like, oh, I need this from you. Oh, can I get then, you know, that’s kind of what shifted instead of like, know about people’s kids and their dogs and
Dave Mariani: Right. Yeah. There’s no chat anymore. It’s just like, this is what I need. Give it to me now.
Megan Brown: Yeah. so one of the things I watched happen was like, there wasn’t just this moment of brainstorming with someone about what already existed. And so there wasn’t that information sharing, like greater information sharing, not just like I need this fact for today and that fact for tomorrow. I think the other thing that happened is we started onboarding people who, you know, we’re based in Seattle, they don’t live in Seattle. How do you as a new employee at any company in the pandemic, that’s now going like hybrid or remote build those same relationships that you would have had walking up and down the stairs with someone in the building I think, yeah. I don’t know if there is a solution for it. I keep asking that question just to get people thinking about it. So I decided that, there were some real concerns, the equity concerns, right
Megan Brown: Like if only people who have that old network are getting the information, that’s a real problem pulling in all these smart, new people, they need equal access. And there’s demographic differences in there too, that you probably should worry about. and then I, you know, realize that people are like emailing people to email people, to email people, to email people. So they wanted this deck from a year ago, but which roles in the meantime. And so it takes them two weeks to get the deck, maybe five hours of focused effort. And it’s like, that’s, that’s ridiculous. We don’t we’re, we’re in the future. We don’t have to work that way. This is, this is how I get myself in trouble. Right.
Dave Mariani: Well, well, you know, you think about it, right It’s like you said, that it’s just, you’re right. The people that have been there know where to go and where to go to get it. And it’s also the people who are technically oriented, who are in, you know, can, can configure it out for themselves, but that’s a small fraction. I mean, it ends up being a small fraction of the business. And so as time goes on, you know, you have more and more people who are, who aren’t using data to make decisions. So, okay. So let’s keep going on. This is, the story is getting interesting now because, because you’ve done something about it.
Megan Brown: one of the first things my team turned out when we were created, in, you know, summer of 2020 was what we call the Wayfinder and, you know, like good data and decision scientists. We tried to build it in the most automated way. So we’re pulling from APIs in tools that we use already. And what we ended up creating in the first pass was a data set and a dashboard of the previous four years of products that, and I had turned out. And so it included, you know, w Starbucks communicates index. So it included decks. Obviously it included white papers, it included videos of events that we’d done. I’m blanking on things, but,
Dave Mariani: But not, I mean, I thought you were going to say, Megan, like, you know, we’re talking about access to, you know, SQL queries and the life that you’re talking about, you’re talking about real, like information here, and you’re talking about, you know, PowerPoint decks and, and videos and the like as well. So it goes much further than just what we, what I think of data in data, in a database somewhere
Megan Brown: Well, and what the business wanted, like the business had the pressing need, what the business wanted was access to the stuff we’d done previously, right? Like it just felt really urgent at that point. What we’ve done since then is we’ve also layered in a sort of github code that’s stored in github. We’ve layered in technical documentation. We are, we’ve done a big push to get our metrics into a metadata platform. And we’re going to pull it from the API of that metadata platform and put it into, we now have the Wayfinder for business stakeholders and the library, for our, our data and decision scientists. And so the code will show up over here, but, the metrics will show up in both, right And so the goal with those metrics is to clean up the space of it, to get a shared definition, to point to dashboards that have that same definition to point at deck technical documentation, for those of us who have to build with it and to get people as close to the code as possible.
Megan Brown: So I could, in my daydreams imagine a brand new data analyst like fresh out of whatever degree program they came from. And, you know, you give them a task. That’s like, I want to see this metric and this metric and this metric over time. And they just like go to the library, they type the names of the metrics and they get the github code, they steal it, they put it into a new notebook and they try like their effort then is a making that code run and be interpreting what they’re finding rather than rebuilding the wheel with a slightly different definition from what everyone else is using or begging someone else to give them their code or, you know, whatever it is in their first three weeks. Right
Dave Mariani: Yeah. That’s a look, that’s one of the reasons not, that was the reason why I started the company at Yahoo. We had the same, that same kind of problem in that, you know, everybody, you know, it’s too hard to find stuff that people have already done or stuff that there is no corporate source of truth. So people go and they roll their own and when they roll their own, it’s going to be different. and it’s, it’s just, it’s, this has to be people, you know, there’s it for you to be the same would be a miracle. so, you know, for us, for me, I call it a semantic layer, but you gotta have some common, you know, set of business terms and KPIs that everybody can agree on. And what you, what you just talked about is no, where to go find them, which is really, really key.
Megan Brown: One of the important things of finding them is I’ve seen a lot of tools that is, you know, everything has a search in it, right Everything you can search, github, you can search confluence, you can search SharePoint, you can search, sharper, you can search all of these places, but only those places. Right So in my daydreams, it’s like an Uber search, everything you have, every tool you have feeds into this search. And so you start to get that graph, like really what I’m after is
Dave Mariani: College graph that
Megan Brown: Graph. What we have right now is not a graph, but I think there’s a lot of very clever people at Starbucks who are pushing in the right direction. And I just happened to give them a proof of concept at the right time.
Dave Mariani: So you have, so you got them, so you got them interested. so as a knowledge graph, your ultimate goal is sort of get that, that Uber searched so people can find that that’s a
Megan Brown: Right. Yeah. If you, if you search for a metric and it’s in three dashboards and you go to the dashboard and you’re like, kind of new to dashboards, there should be a training video and a bug report on that dashboard. And that, that training video might lead to other training videos for similar dashboards or to, you know, decks that came off of that dashboard. So all of these things are connected. Like this we’re in the information age, this, we can’t possibly keep all of this knowledge in our heads. Like it’s, it’s, we really can’t anymore are As easy as possible to see those links and get from one end to the other of the knowledge right now that the knowledge is outside of our bodies to a great,
Dave Mariani: Yeah. And it’s, it’s it’s, and you can’t find out at the water cooler now all the time, any way in any way with our disconnected sort of work styles. but, yeah, but, but Megan, so how is it because there are tools out there for findability, like a data catalog, for example. So is it D do those tools just not sort of go far enough and in sort of creating that knowledge graph for you
Megan Brown: In my mind Yeah. When you think about the business user, they do want to know about the metric. They don’t need the code, and, but they do want to make sure that their analyst is pulling the metric the right way, but then they also want to, if, you know, if they want it to present, prevent themselves from being, you know, caught off guard in a senior leadership team meeting, they might also want to look at some recent texts for that metric to make sure that like, oh, I know how to check my analysts work. Or my analyst has to context with these two decks. Right. it’s not really about either the metric or the data. It’s really about the knowledge and wisdom that come out of the metric and the data. And we can’t get all the way there. Some of those will always be to some extent human processes, right. Creating wisdom. but we can get them closer than just the metrics.
Dave Mariani: Yeah. I really love that. So, you know, so you, you’ve, you’ve, you’ve been through, and you’re continuing this journey. but for the audience, you know, w what are the, some of the things that you think that worked, maybe some of the things that didn’t work, to, so, in this, in this journey to sort of, get more people using data and in Starbucks,
Megan Brown: I think one of the challenges, one of the technical challenges is that SharePoint used to have an API. SharePoint it’s, you know, the backbone of teams. It’s the backbone of,
Dave Mariani: I mean, I don’t even know. It’s, it’s funny. We don’t talk about SharePoint anymore, but it’s like, it’s there. And I’m the president and probably every enterprise, you know, in the, in, you know, in the world. Yeah.
Megan Brown: It’s the easiest way to make a website at a, at a company you don’t have to go through security. You don’t have to go through whatever it’s single sign on. and it has no API.
Dave Mariani: Wow.
Megan Brown: It has power apps internally, but no API. so that’s, that’s the biggest lesson is like, put your whole library there.
Dave Mariani: Microsoft listening, Microsoft
Megan Brown: Guy again, I mean, you know, it’s fine. I think the other learning is you’ve got to meet people where they are. So if you have, you know, hypothetically five different dashboarding platforms, hopefully each of those have an API and you can fold them into the same tool. And maybe then you can start to see, well, this one is really, really unused. And this one’s like only for one area, can we, you know, is there streamlining that can happen. you also will start to uncover processes that really just aren’t working, right So this doesn’t happen at Starbucks, but like if your analytics team isn’t sharing their code and isn’t publishing their code to like, get up, for example, where, where is it going Is it going somewhere else Is it falling into the ether What about get hub Isn’t working for them, you know, and you start to have those process conversations, because all behind the knowledge management is human process. Like where are you? Are you pushing your code? Did you document that project Right. All those processes. So as long as we’ve all agreed that like the code lives here, the tech documentation lives here, and our decks live here, suddenly knowledge management gets much easier. So that’s been a part of the effort.
Dave Mariani: So Megan, that’s, that’s sort of policy, it sounds like that’s policy, right. Is, is that in your scope as, as in your role, can you set that policy or is it more something that’s, you know, is it so because that’s, that’s, that’s the first place to start, right. To make, to set some rules. Okay.
Megan Brown: I’m totally a psychologist about this. so
Dave Mariani: You tell us, I want to hear this. I love social psychology. So
Megan Brown: I would say probably just about this, in that we can have official policy and it can say what we’re supposed to do, and then we have what people actually do. Right. And so my team we’ve actually built up our user research chops in order to figure out what people actually do. And then we can offer some new corrections. We try to keep it really lightweight. We don’t call them corrections. We say, there’s little change. That’ll make your life much easier. Right. It’ll make you more efficient in the long run. So we’re going to change these three things about how we put our decks somewhere when we’re done with them. and we from the research kind of have a plan for how we’re going to feel going off screen with my plan, but for what we’re going to approach next. so their user research gets at the actual practices.
Megan Brown: I think within tech orgs, we know generally what we should be doing, but also we work really quickly. Like we meet the demands of the business as the business moves and the business moves really quickly. So sometimes we can’t do the proper, no, I’ll, I’ll just say like, as a data scientist myself, sometimes I couldn’t do the proper version of things. And, you know, three months later I’d look back and say, oh, I didn’t push that code, push it out. so things like that get in the way. so, you know, you make it as easy as possible. You make it as lightweight as possible, and you look for the incremental changes that will get you closer to pulling from the API. Right.
Dave Mariani: And so you’re, I mean, because you’re a data scientist, you’re using data to make those decisions, right. So it sounds like you’re using, I mean, APIs are important because you’re collecting and monitoring information and you’re using that data to determine where things could get better. Is that, is that accurate Is that a good description of what you’re doing
Megan Brown: for example, there’s a team. It’s a very conscientious team that turns out a lot of dashboards for analytics and insights. they were using some fields within Tableau, but not necessarily the description field. Right. And so as soon as we started pulling from the Tableau API, that just became painfully obvious. Cause what are we supposed to search on
Dave Mariani: Yeah. You’re talking about metadata now. And there was, there was no metadata, there,
Megan Brown: There was no metadata. And so we went back to the team. We were like, Hey, could we maybe think about doing this in the future And they were very happy to help because all they wanted was the adoption of their dashboards. So, you know, some changes are really easy like that. Like we can drive traffic to your dashboards, just put some more information in here. I think the last thing that I’ve learned is marketing.
Dave Mariani: I was just going to say, marketing’s an important part of this role. Yeah.
Megan Brown: It really feels like you have to remind people like the Wayfinders pretty cool and they get there and like, oh, I can find this. And I can find that, I’ll look at that test, you know, but you know, two weeks later they haven’t looked at it and like, you know, so you’ve got to remind them, you have to have the Wayfinder, like a link showing up routinely. I think I put it into the chat and every single meeting I’m in, like, just like it’s in our newsletters, it’s in our, our knowledge drops. It’s in, like, if I could get it into the template of one of our decks, I would, you know, put the link. and that, that really was a, I, I kinda, I knew that that was going to be a thing, but I didn’t understand just how much of a thing.
Dave Mariani: Wow. It’s like, you know what you’re describing I mean, I think a lot of people think of data literacy. They’re going to go buy some product. and then that’s going to be it, but it really is a people organization and technology sort of opera, opera. It’s an operation. and it’s not something that you just, you know, go and buy a product off the shelf and, and then put it up there and then they will come and you will use your knowledge and data literacy problems are solved. it takes, it takes, it takes effort and concerted effort and marketing and continued education. So I really love what you guys are doing. I think
Megan Brown: It goes people, process tech, process people.
Dave Mariani: Hmm. Oh, that’s interesting. You took it a little further
Megan Brown: Over here. The knowledge management part is over here, but the, you know, you can have some really dumb tech, like there’s tech that like, feels like it solves the problem. Yeah. But no one uses it or no. One’s putting the data in there. It’s awesome.
Dave Mariani: We see that all the time. We see lots of customers making big investments in technology and it’s sitting on the shelf and it makes them feel better that they bought it and they think, okay, I budgeted and I did it and I bought it and this is solving the problem. If nobody’s using it, then it’s, it’s just shelf ware. so I’m totally hearing on that. So, let’s wrap up with the future. So I’m making more, where do you think data literacy is going, from, from here you know, you with your, your experience, what, what do you think, what do you think we’re going to be seeing next in this
Megan Brown: I think people are starting to be more aware of data literacy. We’re seeing a lot more consulting firms come through with data literacy. Now
Dave Mariani: That’s a sign, that’s a sign of interest. There’s money, money there, all the money that my dad always said.
Megan Brown: I think the big questions for me are, can you have general data literacy training, right? You can talk about distributions made medians, modes, cue, outliers, right. You totally can. will that be as effective as getting it to the data in the tools that your organization actually has Right. So I, I think one of the challenges here is the pandemic everyone’s fried. Like everyone’s so tired. We’ve been on video all day, every day for two years, the trainings are on video. Everyone’s multitasking. And it’s like, so we also have to be really mindful about the quality of educational opportunities we’re putting out there. They have to be really engaging and they have to be interactive. So there’s this question about how do you create a program that is somewhere between, between generic content and super personalized content, and then how do you find the right point in the middle So you can scale it because everyone at the company needs that course. But if you’re only running 10 people through that course at a time, it’s not going to go super fast.
Dave Mariani: Yeah. You’re not going to get, you’re not going to make progress. So it sounds like, you know, figuring out how to scale education is an area you think there could be more work done in this, in data literacy.
Megan Brown: Yeah. I think I also see the question I’m always asking myself is how close is this to the person’s actual work. Right. If they’re not, if they’re in a course and they’re like, yeah, cool distribution medium. Yeah. Cool, cool. I learned that, you know, in my stats course in college. Great check. do they actually use any of that the next day This is also totally a psychologist question. Like, did they apply it the next day and then did it generalize to other things And that generalization is never easy. In terms of the future of data literacy, I think, I think the most challenging thing with data literacy and this came up just yesterday is that it implies that people aren’t literate.
Dave Mariani: That’s very true. It is kind of an insult, isn’t it? Because if you’re not, it’s like you’re illiterate, you know, your data illiterate. I don’t even think about that. That’s a market, that’s a marketing issue. It is a
Megan Brown: Marketing.
Dave Mariani: I don’t want to make people feel bad about themselves.
Megan Brown: So, so, you know, a leader brought that up and I was like, Ooh, I’m extent. How much does it actually matter what we call it, if we’re still doing the education of our, all the people at our business. but to another extent, it’s like the psychologist. I mean, it’s like, but we’re not illiterate, but also like we would never do that in business. Right. Like we would say the data future, we’re going towards, You know, come with us on this journey to the future.
Dave Mariani: So,
Megan Brown: So, you know, I think that’s an open question. And as we move closer to the business, we probably have to think about that.
Dave Mariani: I’m completely with you. So that’s a great way to finish this thought. That’s a challenge for the audiences to come up with a better term. But, but Megan, you’re a pioneer in this area and it’s been amazing to work with you and hear, hear your experiences. So thanks for joining the podcast today, and, wish you have a great rest of your day and to everybody out there in the audience, be data-driven and thanks for listening.
Megan Brown: Yeah. Thanks for having me. Let me know if you solve the data literacy naming problem.
Dave Mariani: Likewise, take care.
Megan Brown: Bye.