December 17, 2019
Big Data Analytics in the Cloud for Today’s Distributed and Diverse Data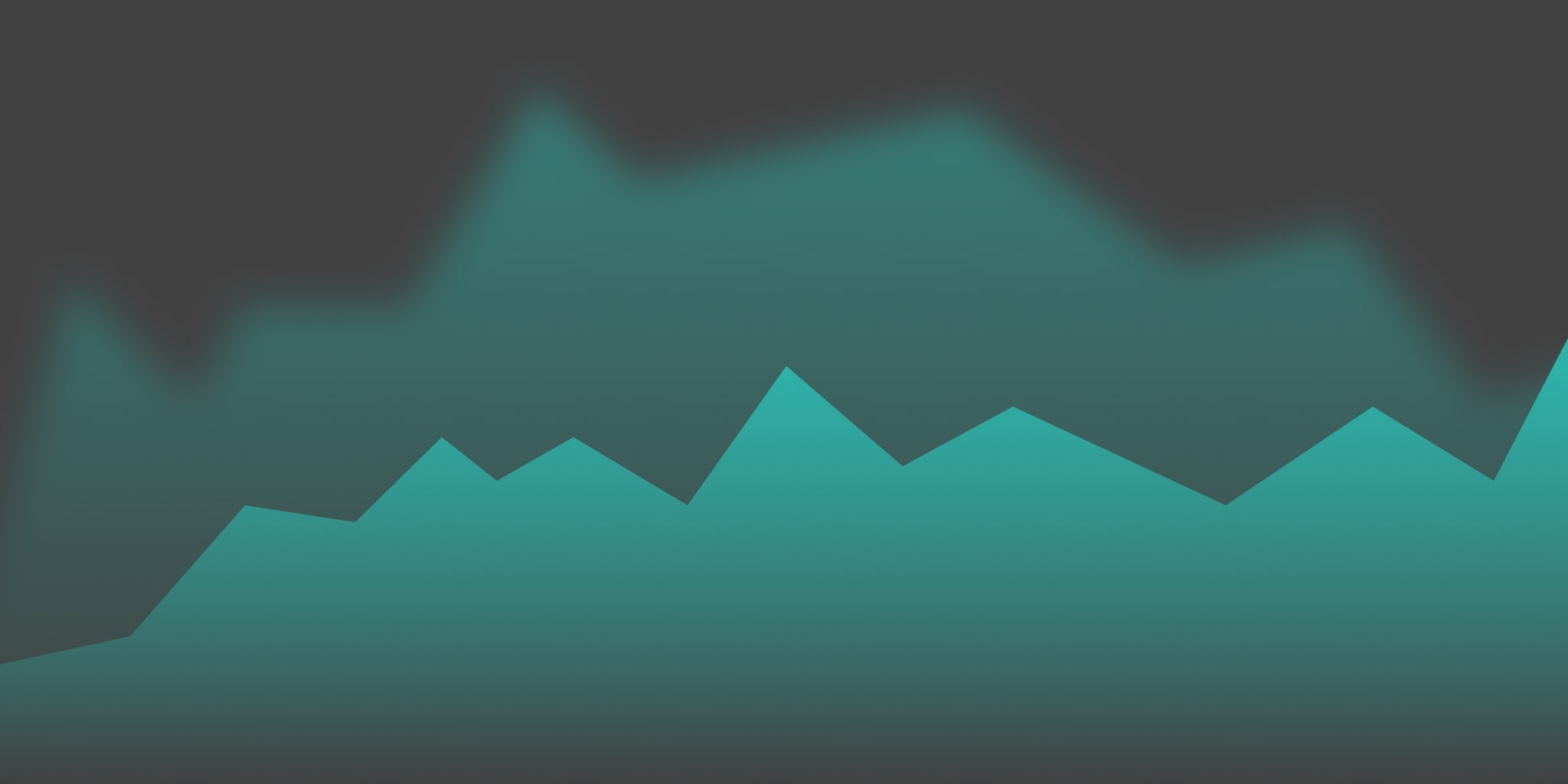
In order for big data analytics to work optimally, data must be consumerized–that is, it must be readily available to all stakeholders across the organization in a self-service manner, while ensuring reliability and security.
What are the most common barriers to that?
The Challenges
Proliferation of platforms
Data infrastructure is changing rapidly. For forty years, there were ten major database platforms. Ten years later, there are at least forty.
This proliferation of platforms means that not only are there more proprietary formats, but there are also now more systems with specializations and advanced performance for specific kinds of data, including time series, graph and text databases. This means data engineers must become experts in a wide variety of technologies, or many more data engineers need to be hired.
Data will always be distributed
Data is also becoming more diverse, dynamic and distributed.
Because of the speed at which new data sources are being added to organizations’ data footprints, and the number of new database types to suit many different use cases, data will likely always be distributed. There will always be more projects and technology added to IT ecosystems, and new local databases will always be created.
Data engineers are expensive and difficult to hire
Data engineers are expensive to employ, and finding qualified candidates who will add value to your business is arduous in itself.
A quick search on job hunting engines shows 7,500 open requests for data engineers in San Francisco alone. Meanwhile, there are only 7,400 people with data engineering job titles on Linkedin for all of the United States. This critical shortage in skilled data engineers is preventing business users and researchers from provisioning models, conducting analyses, and deriving critical business insights.
The consumerization of IT makes business more demanding
Many aspects of IT have become democratized – technology has made them accessible to laypersons, and they are no longer the exclusive domain of certified experts.
However, this democratization has unfortunately not, for the most part, applied to analytics. The fragmented state of data in most organizations leave analytics very much in the hands of specialized IT wizards. This is because data engineering as a core profession has only recently come into being, so this tribal knowledge is not yet automated.
Hybrid cloud increases surface area for security risks
Hybrid cloud adoption is increasing exponentially. Gartner predicts that by 2020, 90% of organizations will be utilizing a hybrid cloud infrastructure.
However, having both cloud and on-premise systems also creates additional security challenges. More surface area is exposed, and there are more technologies to manage. The pace and adoption of hybrid cloud and new technologies has outpaced the maturation of security tools and technologies. The result is that many organizations are now more vulnerable to cyber-risks.
The Good News
The good news is that we are within striking distance of a new, self-service analytics culture, where data is consumerized throughout the enterprise. This means organizations will no longer have to wait for data engineers to prepare data, or rely solely on data scientists to analyze data to derive actionable business insights. It is now possible to make data discoverable, agile, and operational for all of your business users.
However, there are certain prerequisites an enterprise must meet before successfully implementing self-service analytics. The best way to achieve this and truly consumerize data across the organization is with an adaptive analytics fabric–a new approach to accessing and using all of an enterprise’s data, without wholesale movement of data. An adaptive analytics fabric provides:
Autonomous data engineering
The shortage of data engineers means that organizations need to automate as many aspects of data engineering as possible.
Autonomous data engineering uses machine learning (ML) to look at all of an organization’s data, how it’s queried, and how it’s integrated into models being built by users across the enterprise. All of this information is then analyzed and used to build acceleration structures that reduce query times. The appropriate tool for the job is identified automatically, leveraging strengths and placing data where it will achieve optimal performance. This turns a traditional liability—the variability of all your different database types— into a strength.
Cloud cost predictability
As beneficial as the cloud is, research shows that costs are often unpredictable and can easily spiral out of control. The anticipated cost savings of hybrid-cloud environments can’t be realized unless resource usage is well-managed.
Acceleration structures that optimize queries, enabled by autonomous data engineering, save a tremendous amount of money on cloud operating costs. Depending on the platform you’re using, you may be charged for the storage size of your database, the number of queries you run, the data being moved in a query, the number of rows in a query, the complexity of the query, or a number of other variables. With autonomous data engineering, the same query yields the same answer automatically using acceleration structures for both performance and cost optimization. You’re only charged for the data you use in the acceleration aggregate, not the size of the entire database.
Automatically enforced governance, compliance and security
Data accessed via an adaptive analytics fabric inherits all of the security and governance policies of the database where it resides–the source data is virtualized and thus doesn’t move and isn’t ever touched by the users.
While your data is readable to all of your users and a multitude of different BI tools, your permissions and policies are not changed because nobody is accessing databases directly. Security and privacy information is preserved all the way to the individual user by tracking the data’s lineage and the user’s identity. The user’s identity is also preserved and tracked even when using shared data connections from a connection pool. When users are working with multiple databases with different security policies, policies are seamlessly merged, and global security and compliance policies are applied across all data.
Discoverability and self-service
The cloud era has made discoverability particularly difficult. Data is now distributed across multiple formats and systems, making it difficult to locate, access, and integrate for analysis. If you have incomplete data, or if it’s out of date, the results of your analysis could be unreliable.
With an adaptive analytics fabric, all of your data is available for analysis through a virtualization layer, without the end-user needing to know where or how it is stored. A universal semantic layer creates a common business logic that is tool agnostic, resulting in consistent answers and reports across users and teams. Now, all departments can not only access and analyze data for their own specific purposes, but also act cohesively, making insight-driven decisions for better business outcomes.
The Way Forward
Leveraging an adaptive analytics fabric is the most efficient, worry-free, and cost-effective method to consumerize your data and bring the transformative power of self-service analytics to your organization. AtScale has saved tens of millions of dollars for our customers while enabling and optimizing their big data analytics, propelling them into a new playing field of agile, data-driven strategy and decision making.
Learn how to overcome the challenges of Big Data Analytics by downloading our white paper Big Data Analytics for Today’s Distributed, Dynamic and Diverse Data.
ANALYST REPORT