June 1, 2020
Analytics Leader Spotlight: Mark Stange-Tregear, Rakuten Rewards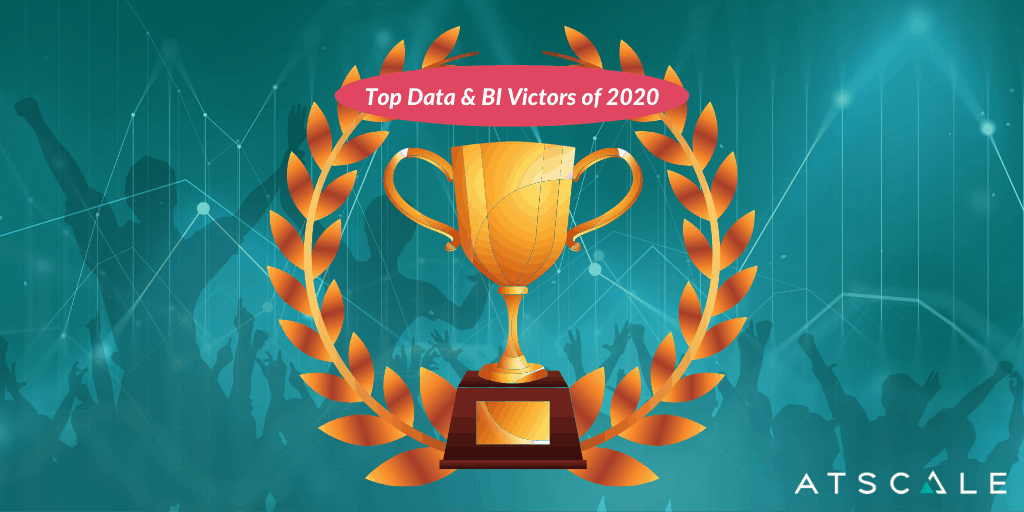
For the first time, AtScale is excited to feature top Data and Business Intelligence leaders across various backgrounds and industries. Our seven BI Victors of 2020 share their accomplishments, best practices, and advice.
What Does it Take to be a “Victor” in Data or BI?
A Victor is an experienced professional in the Data or BI space who constantly strives to achieve excellence through research, education, or within the enterprise. They help others outside their expertise understand the importance of Data and BI.
Whether it’s taking a leap of faith on a challenging project or orchestrating a data-driven story for the enterprise, all of these leaders epitomize what it takes to be a Victor.
Jen Stirrup, Technical & Business Strategist, Director of Data Relish
What makes me a Data Victor: I have a passion for making data work at organizations where previous attempts have failed. This means I inherit difficult projects that have a history, so they start with a negative balance. It’s very rewarding to turn these projects around. I also enjoy risky projects where I am a ‘canary in a cage’ and the first person to try something out.
Best Practice: The “soft skills” are the “hard” skills! Data does not add value on its own. People add value by understanding and using data. This involves communicating a vision, and it also involves listening to the business people. It takes courage to stand up and talk, but it also takes courage to sit down and listen, particularly if the data does not look it is going your way.
My advice: Own everything you do, and try to do your best at it. No matter how ‘small’ or menial it may seem, if you can’t do the ‘small’ stuff well, you will not get to do the ‘big’ things well.
Bob E. Hayes, Ph.D.
What makes me a Data Victor: I try to convey the importance of understanding statistics (and data science methods) for the 99%. You don’t need to get a PhD in research or statistics to get value from understanding statistics.
Best Practice: I write blogs about data science to help my readers (e.g., aspiring data professionals and customer experience professionals) understand the importance and value of statistics and how they fit into the larger picture of data science. Here are a couple of blog posts where I try to achieve that goal:
- Making Sense of Our Big Data World: Statistics for the 99%
- Statistics: Is This Big Data’s Biggest Hurdle?
- Demystifying Data Science For All
My advice: Find out what you’re good at (e.g., domain expertise, math/stats, or programming) and passionate about (e.g., healthcare, education, business, marketing) and pursue a career in that.
Ganna Pogrebna, Professor of Business Analytics and Data Science at Columbia University
What makes me a Data Victor: I am a behavioural data scientist. I believe that behavioural data science is the future. Behavioural data science (which is often confused with behavioural analytics but, in fact, is not synonymous to it!) emerges as a direct response to the need for studying behaviour “in the wild”, outside the “sterile” laboratory setting and controlled environments. It concerns human, algorithmic as well as systems behaviour. Decision support systems, suggestion systems, automation, etc. — all these technologically intense aspects of human life require accurate predictions of what people like, what people prefer, and where people need help from automated agents and/or algorithms.
Further, we need to better understand how humans and algorithms can harmoniously co-exist in a system as well as how to make these systems resilient to change. In my work, I try to get us one step closer to developing analytics and technology that really understands humans and works towards achieving their goals and needs, maximising their potential.
Best Practice: I love two things about my job: (i) as a behavioural data scientist I get to work on many different projects (from how people make purchases in the supermarket to how astronauts make decisions under risk and uncertainty in space) and this is really cool; as well as (ii) diversity and inclusivity of the field, where we have thinkers (who understand the “black box” data science/AI models conceptually); do-ers (who apply “black box” models in practice) and developers (who change “black box” models, perhaps even working on making sure that the models we use are no longer “black boxes”).
One of the main challenges for me is always about learning new tools, or, finding the time to learn new tools. There is always something new to learn and it is easy to miss, as there is a lot of information out there about data science. For example, I now realise that my knowledge of Python is not enough for everything I do, so I am learning Julia and it takes time to do that. Another important challenge for me is thinking about how to make sure that all models and tools I develop use people’s data in a responsible way. It is very easy to hurt people with data science tools, so I always try to anticipate the potential adverse effects of the behavioural data science models I develop. In facing all these challenges, two brains is always better than one, so I really try to involve researchers and practitioners from many different fields into what I do.
My advice: Firstly, treat every obstacle as an opportunity. Many people give up too early and it is a shame. And second, have a BIG dream. So big, it might sound crazy to other people. I believe that if you have your eyes set on a BIG dream, you will push yourself to achieve more! James Cameron famously said: “If you set your goals ridiculously high and it’s a failure, you will fail above everyone else’s success.” It is so true!
Mirna Domancic, Senior Data Analyst at Hulu
What makes me a BI Victor: I am always aware that the field we are in is constantly changing and there are a lot of different skill sets in it – being aware of your own strengths while being open to constantly learning from others is important.
Best Practice and advice: There are different ways to present the same data insights. Always make sure to tailor your presentations to the type of audience you are talking to – sometimes a more technical approach will take you a long way, while other times a different point of view will be the way to go.
Jerrin Jose, Senior BIE at Amazon
What makes me a BI Victor: Having the bird’s eye vision to understand business problems and requirements and then translating them into a highly technical SQL query.
Best Practice: Whenever I get some requirements to build a tool, dashboard, dataset, or investigate an issue – I think, “What would I do if I had owned this company? What would be the probable bottlenecks that would be causing this?”. By quickly changing into an owner mindset from an employee mindset, you are automatically getting closer to your business and stakeholders. This also enables you to be more razor-focused on building scalable solutions.
My advice: Invest in your communication. In the end, the big difference between a good BI Victor and an excellent one is not the technical skills but the ability to convey a data-driven story beautifully to your stakeholders in the right language (without misinterpreting the acts of course!) 🙂
Yasir Ali, CEO of Polymer
What makes me a BI Victor: I am the founder of Polymer. Our database connector connects with all BI tools to access databases and secures sensitive data access by modifying queries in real-time. I built this company & product to make BI tools easier to propagate across organizations without having to compromise privacy and security.
Best Practice and advice: Access controls and privacy should be paramount in designing BI solutions. Exposing sensitive data is easier than some people think. With the adoption of self-serve installs, it’s important that controls are put in place without having to limit innovation and Data-driven decision making.
Abilash Reddy, Merchandise Planner, BI Product Developer at The Walt Disney Company
What makes me a BI Victor: Picking the right data and frequent reconciliation of the data.
Best Practice and advice: Always set a goal in mind and crunch the problem into pieces, one small problem at a time, clean your data, and make sure it is the right set for the problem.
Do you have a story to share with us? Send us a tweet @AtScale!
Or, email me directly: mary.ohara@atscale.com
NEW BOOK