AtScale forms a bridge between AI and BI while establishing the foundation for simplifying AI/ML pipelines and accelerating feature engineering by data scientists.
Build a Common Data Language
The AtScale semantic layer can establish a common definition of metrics and dimensions for data science and business intelligence teams.
Eliminate inconsistency and duplicative work that happens when data science and business intelligence teams look at data differently.
A single source of governed analytics, that can be continually updated and managed as the business evolves, creates trust in data and fosters a culture of collaboration across all data consumers.
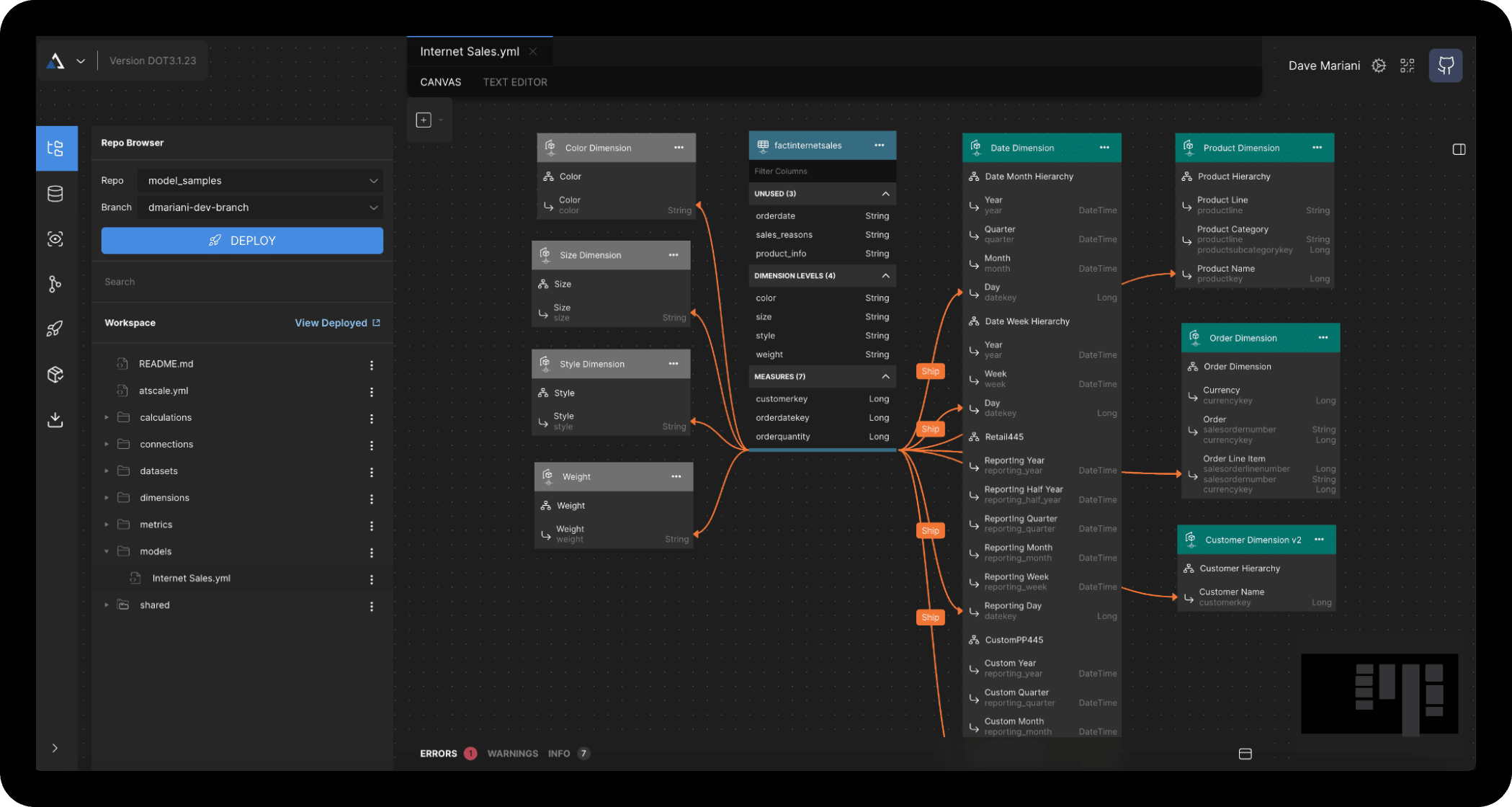
Simplify Feature Engineering
AtScale includes a powerful feature design utility that supports both code-based and visual data modeling. Data teams can collaborate closely with business users on engineering features defined on top of raw cloud data.
Transform numerical fields to categoricals. Build custom-calculated fields. Easily create time-relative features with a full range of lags. Feature engineering at the semantic layer is dramatically simpler than physical transformations, as it exists purely as a logical definition.
Harden AI/ML Data Pipelines
AtScale serves features on demand by leveraging a powerful query virtualization platform, meaning data is not physically moved into the AtScale platform. Once a feature is published, AtScale can dynamically generate queries against source data based on the logical feature definition, allowing downstream pipelines to consume features from the semantic layer.
Leveraging AI-Link, features can be leveraged and bi-directionally managed through Python scripts. This approach radically simplifies AI/ML data pipelines while hardening against disruption caused by changes to underlying feature definition.
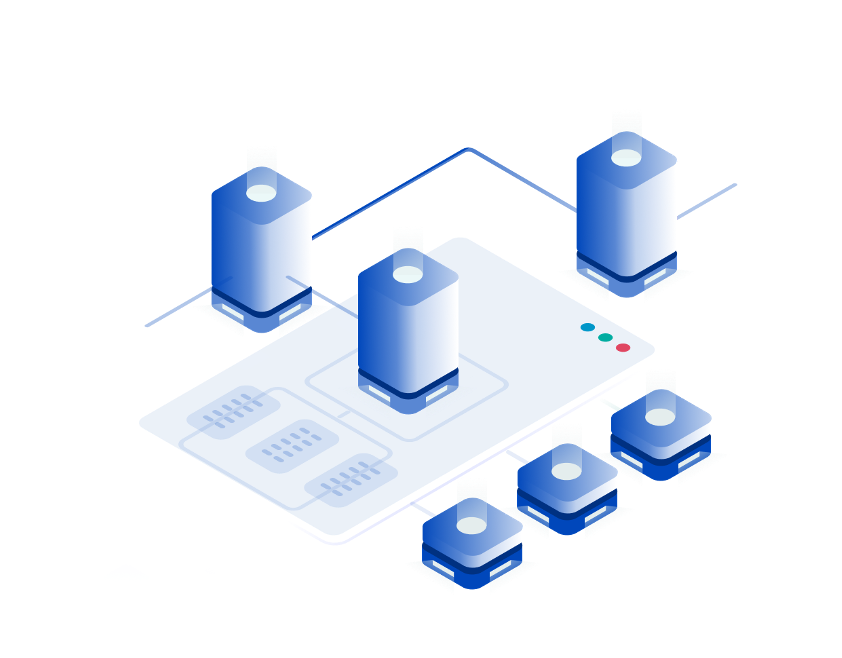
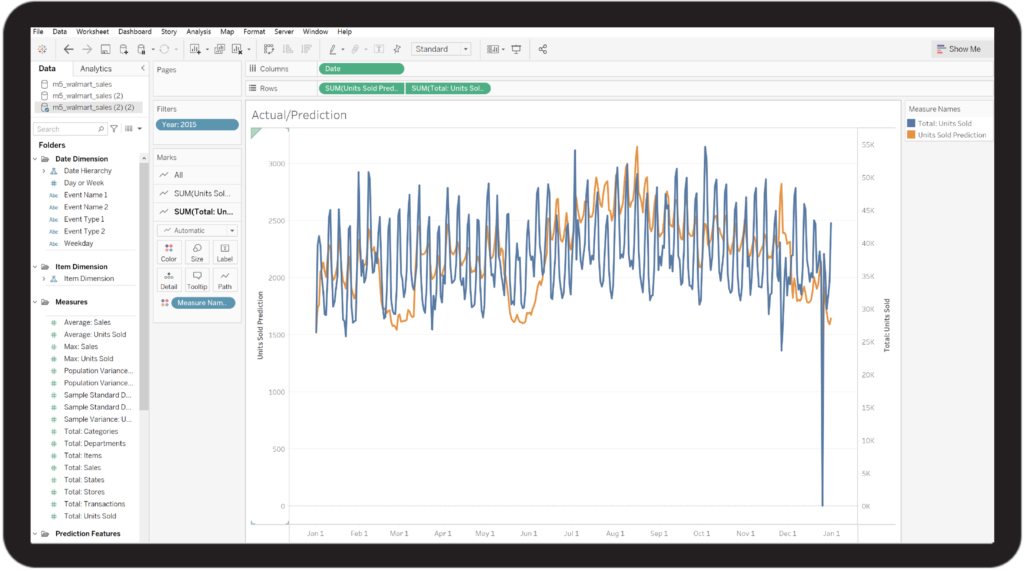
Publish Model Results to Existing Dashboards
Machine Learning Model-generated insights, like predictions, can be published back to cloud data platforms and the semantic model. This approach lets modeled insights integrate with the same semantic structure the business is already utilizing. Thus, predictions can easily be consumed with the same BI platforms already in use by the business
By leveraging dimensionality established by BI teams, decision makers can more confidently navigate large predicted data sets — using the same time, product, and geographical hierarchies to analyze predictions as they would actual data.