March 6, 2019
TECH TALK: AtScale 6.0 brings Universal Semantic Layer Benefits to Google Cloud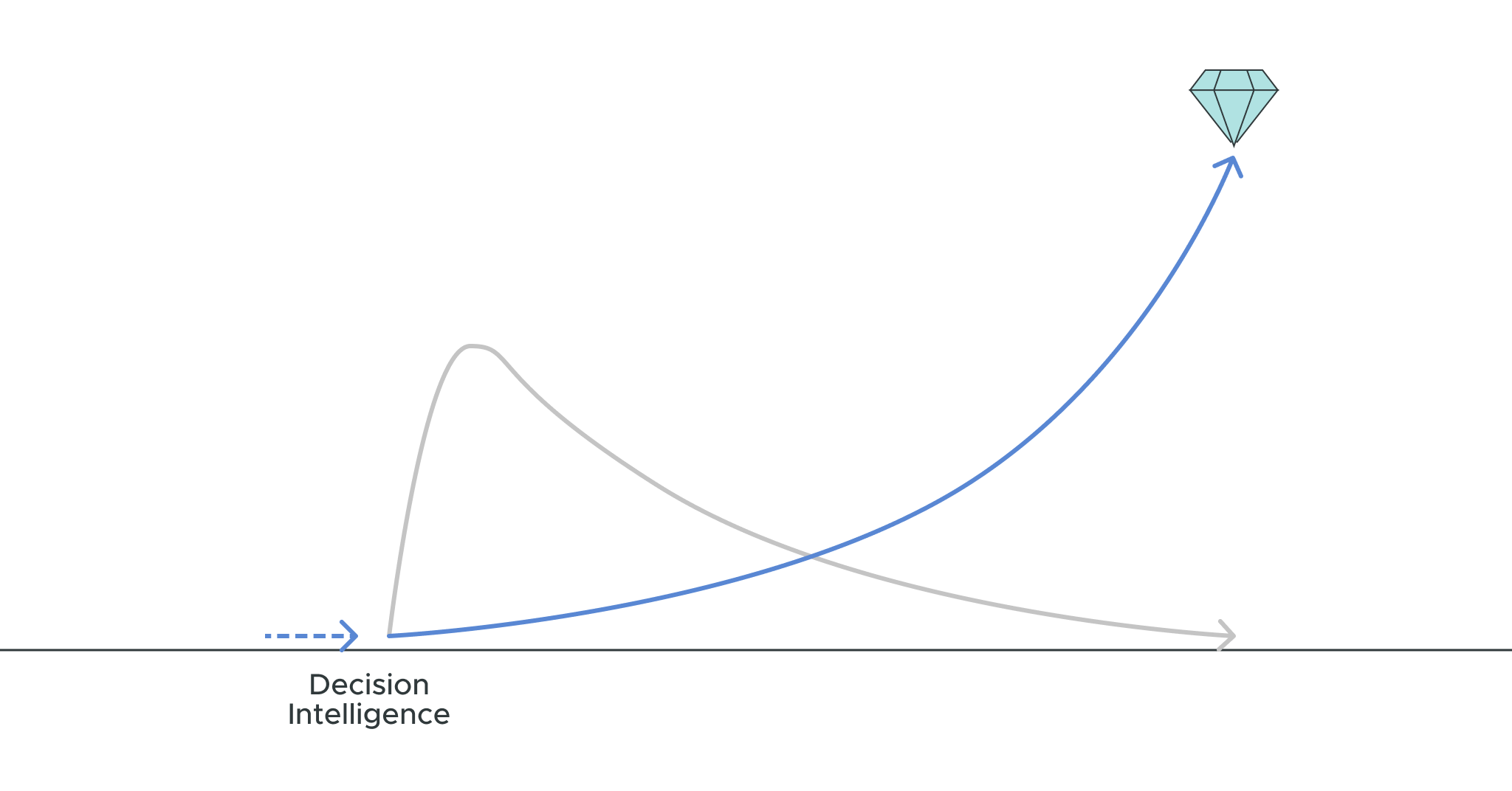
This blog post is part of a series from Ganes Kesari, entrepreneur, AI thought leader, author, and TEDx speaker. He co-founded Gramener, where he heads Data Science Advisory and Innovation. He advises executives on decision-making with data. He helps apply data science to solve organizational challenges, tell stories with data, and build winning analytics teams. Follow him on LinkedIn.
This is the fourth and final post in the blog series on Decision Intelligence. Check out the earlier posts: Why Decision Intelligence is the Most Important Data Analytics Trend of this Decade, How to Implement Decision Intelligence in Your Enterprise With a Semantic Layer, and How You Can Unlock Decision Intelligence At Scale by Aligning BI with AI.
—
Today, most organizations believe in the power of data and analytics. Almost every executive aspires to build a data-driven organization. However, a survey by New Vantage Partners found that just 26.5% of companies have done so successfully.
What holds teams back from this coveted goal? While advising business leaders on building a data culture, I’ve encountered three common data analytics challenges. Below, we’ll look at those challenges and review how decision intelligence can help address them.
Why is it so difficult to build a data-driven organization?
A data-driven firm is one in which decision-making at all levels is firmly grounded in data and analytics. There are three significant roadblocks leaders face while operationalizing data and analytics to deliver business benefits:
- Ineffective collaboration amongst business and technology teams
Technology, data, and business teams operate in silos in many organizations. Suboptimal collaboration impacts the kind of data and analytics projects that get picked. It affects how projects are executed and gets reflected in the poor adoption of technology solutions by end users. As a result of poor adoption of data analytics solutions (ex. BI dashboards or AI solutions), it’s no wonder that data is disconnected from these firms’ business decisions. - Lengthy timelines to deliver business insights
Firms that get past the collaboration challenge often need help to deliver insights effectively and in a timely manner. It takes a painfully long time to source data, compute the business metrics, identify insights from analytics, and present them for actionable decisions. So long in fact that the data insights identified often go stale — or business users move on to their next challenge — by the time the insights are delivered. - Duplication of efforts across the organization
When organizations tackle the above challenges by managing team collaboration and devising an effective data analytics process, they do it inefficiently and in an unscalable way. Imagine multiple business units across the firm or geographies devising their own data-to-decisions lifecycle. This wastes organizational effort and impacts business agility.
Examples of how decision intelligence can help
What is Decision Intelligence? In an earlier blog post, Why Decision Intelligence Is The Most Important Data Analytics Trend Of This Decade, I explain how the use of actionable data insights using Decision Intelligence delivers effective, impactful, and timely decisions across an organization at scale. We demystified Decision Intelligence and looked at its three disciplines – cognitive science, data analytics, and organizational intelligence in another blog post, How To Implement Decision Intelligence in Your Enterprise With A Semantic Layer.
Interestingly, the above three disciplines of decision intelligence help build crucial capabilities across the three components of people, process, and technology. All three are essential to unleashing data-driven decisions at scale within a firm.
Let’s look at three ideas to operationalize data and analytics by improving these three components:
- Organize teams as hub-and-spoke [People]
Organizations beginning their data analytics journey typically start with a centralized structure. Centralized data and analytics teams are easy to set up and manage but have suboptimal business integration. On the other hand, fully decentralized data and analytics teams are embedded in the business, are more agile, and deliver better business value. However, they duplicate efforts and often reinvent processes across teams.Mature data and analytics teams often settle on a hub-and-spoke structure: a centralized data and analytics team sets the tone for the organization and devises processes. The spokes are specialist teams embedded deep in the business. They embrace the central direction and combine it with localized domain know-how to deliver value for users effectively, scalably, and sustainably.This idea tackles head-on the first challenge we covered earlier — ineffective collaboration amongst business and technology teams.
- Embrace a data product approach [Process]In the traditional approach to data analytics, teams begin with business use cases and then design data pipelines and data stores. This backward integration makes the data engineering and data transformation efforts inefficient and unscalable. Imagine how this would play out at an organization operationalizing a few dozen use cases.Mature data and analytics teams treat data like a product (see blog post How You Can Unlock Decision Intelligence At Scale by Aligning BI with AI). This novel approach designs a data store aligned with a business entity, which can then serve many business use cases downstream. For example, say a customer data product is designed to provide a 360-degree view of customers. This single data product can serve multiple use cases, such as customer BI dashboards or customer churn predictive modeling.
This idea addresses the second challenge by reducing the cycle time to deliver actionable insights.
- Adopt a semantic layer [Technology]Organizing teams as hub-and-spokes and enabling them with data products calls for solid technology capability. With traditional approaches, data and analytics teams end up duplicating efforts across the data and analytics lifecycle. For example, while identifying analytics insights, business metrics are computed in non-standardized ways across teams. This leads to inefficiencies and multiple sources of truth within an organization.A semantic layer addresses this by centralizing business metrics computation by the hub teams as part of the data product design. A good semantic layer enables the blending of data across multiple data platforms and data sources. Access to metrics is then federated across the spokes teams, which helps avoid costly (re)computations for individual applications.Thus, this idea addresses the third challenge of effort duplication across the organization.
Decision Intelligence is the missing puzzle piece
Leaders have been spending millions of dollars to upskill teams on data and analytics, devising processes around modern data platforms and buying expensive tools and technology. Despite the best intentions and efforts to realize business ROI, many wonder why things haven’t been clicking into place.
Without a philosophy that explicitly connects the people, process, and technology aspects, orchestrating data-driven decisions is impossible. Across the four posts in this blog series, we’ve learned how to get started with decision intelligence. Through innovative ideas and industry examples, we’ve seen how the principles of Decision Intelligence can make your data dollars deliver transformational business value.
ANALYST REPORT