January 12, 2023
The Definition of Data Mesh: What Is It and Why Do I Need One?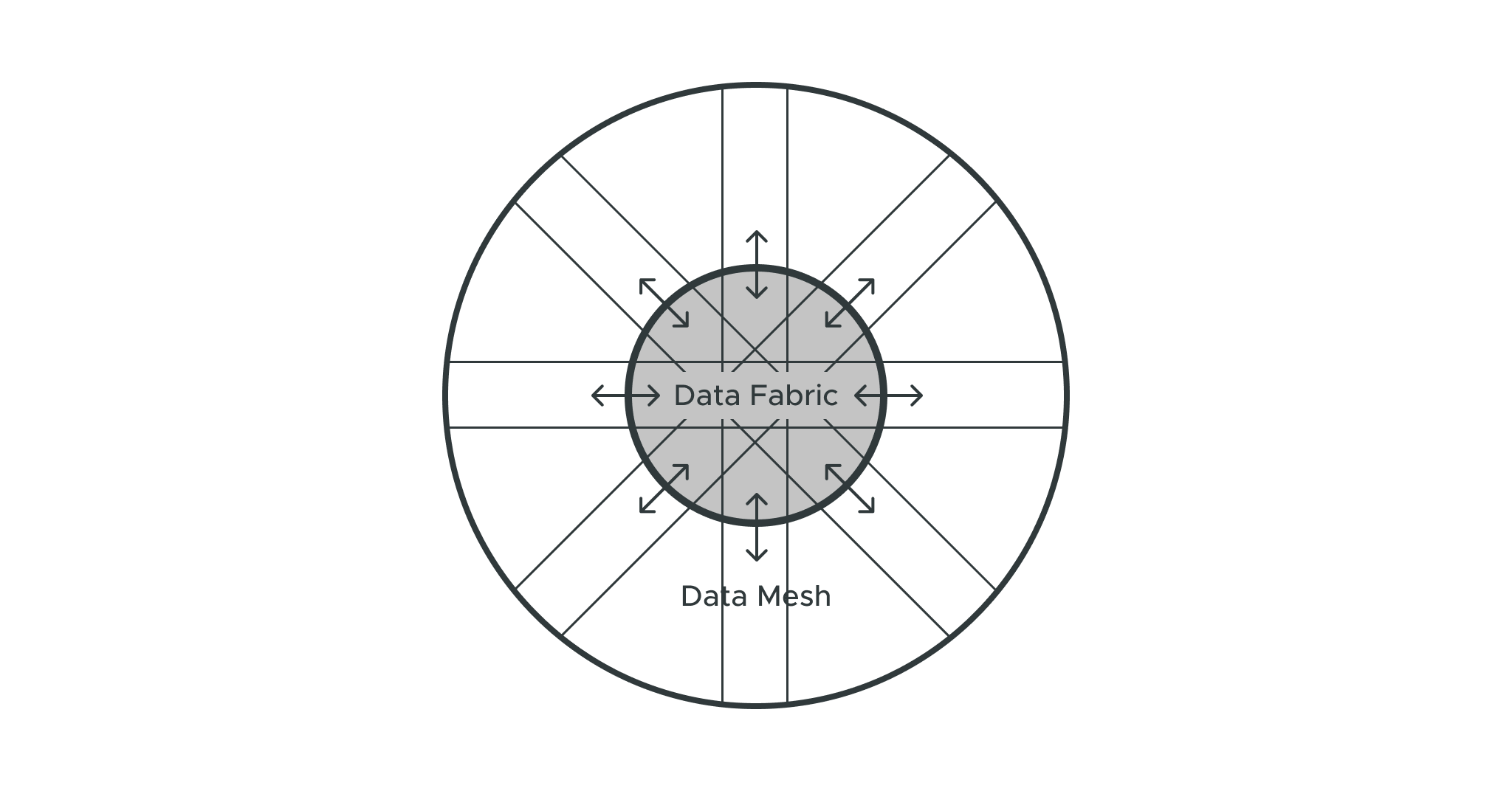
I hosted another round of interviews with data leaders about how they deliver AI and BI for their organizations. We discussed the combining of data fabric and data mesh, the hub-and-spoke approach, and the future of a federated model for data analytics.
The data leaders I spoke to included:
- Ujjwal Goel, Director of Data Architecture and Data Engineering at Loblaw
- Andrea de Mauro, Head of Business Intelligence at Vodafone
- Biju Mishra, Director of Corporate Business Services and Automation at Enbridge
A Hub-and-Spoke Approach for Data Management
According to Ujjwal Goel, a clear vision is crucial for modernizing data analytics at an organization. At Loblaw, this vision is what Goel calls a “centralized to decentralized model.” Or in other words, a hub-and-spoke approach to data analytics.
It starts with the data fabric (the hub) — a centralized architecture for orchestrating data. The data fabric relies heavily on metadata to continuously identify, collect, cleanse, and enrich the data. In addition, Goel sees enormous benefits to a centralized governance approach, though there could be potential for a federated governance model in the future.
The data mesh, on other hand, is a decentralized and distributed solution that puts data ownership in the hands of different teams or domains (the spokes). The problem with the classic version of this approach, according to Goel, is that domains are creating their own data pipelines, leading to data duplication and technical debt.
“The data mesh is a new concept,” Goel explained. “And I personally believe it cannot be fully implemented until the organization is on the top of its game for data literacy.”
When there’s a high level of data literacy and centralized data fabric, each domain can build its own data products and overcome the pitfalls of data silos.
“We, as the data team, see ourselves as enablers,” Goel concluded. “That’s how the mix of data fabric and data mesh becomes the best of both worlds.”
// You may also like: The Principles of Data Mesh and How a Semantic Layer Brings Data Mesh to Life //
Centralized vs. Decentralized Governance Models
“The analytics journey is first and foremost an organizational and cultural challenge,” stated Andrea de Mauro of Vodafone. He believes technical complexities are much easier to solve once you’ve implemented an effective organizational structure to support self-service analytics.
From an organizational perspective, there’s a continuum of possible ways to do this. On one end, you have fully-centralized data governance. Usually, this is the case when companies are at the beginning of their analytics journey with a lower level of maturity.
Centralized governance usually means a single center of excellence (usually data scientists and business analysts) working towards analytics initiatives. However, this approach doesn’t fully utilize the domain knowledge of the business.
As de Mauro explained, fully decentralized organizations are at the other end of the spectrum, with data scientists embedded into business units. This way, they can understand the business better and proactively anticipate business needs. However, these organizations lose out on the cohesion of a centralized analytics approach.
“I think where most companies end up is somewhere in the middle with a hub-and-spoke organizational model,” de Mauro said.
In this situation, you have a centralized data team working on scaling data and analytics and collaborating closely with embedded analysts who are part of the broader enterprise data program.
“It’s not easy to implement,” de Mauro concluded, “but it’s been proven to be the most effective, scalable, and sustainable organizational approach for enterprise analytics.”
// You May Also Like: Improving Data Analytics: Key Insights from Fifth Third Bank, Stanley Black and Decker, and Snap Inc. //
How To Facilitate Quality Self-Service Analytics
“Organizations have evolved over time, often taking important data capabilities and centralizing them,” stated Biju Mishra. “But I think the future is a more federated model.”
This means having some centralized capabilities for maintaining standards and protocols across the organization while also allowing different departments and business units to find and use information.
“One of the challenges with this federated model is making sure that when people look at the information that they have, that it actually makes sense to them,” Mishra suggested.
With a federated model, there’s always the risk that someone will interpret data incorrectly because they don’t fully understand its context. Mishra believes a great analytics organization trains people about data while also making it easy for them to access the correct data.
Learn More About Delivering Actionable Data Insights
In short, each of these data leaders believes in combining centralized and decentralized capabilities. The centralized function can drive economies of scale, ensure standardization and quality, and spread education and understanding. Then, the decentralized capabilities enable people across the organization to access the data they need to do their jobs effectively.
You can watch the whole discussion I had with these data leaders about How to Deliver Actionable Business Insights with AI & BI. In addition, we put together an eBook about how to Make AI & BI Work at Scale, featuring 15 thought leaders and experts.
WHITE PAPER