April 11, 2023
Empowering FinOps With a Semantic Layer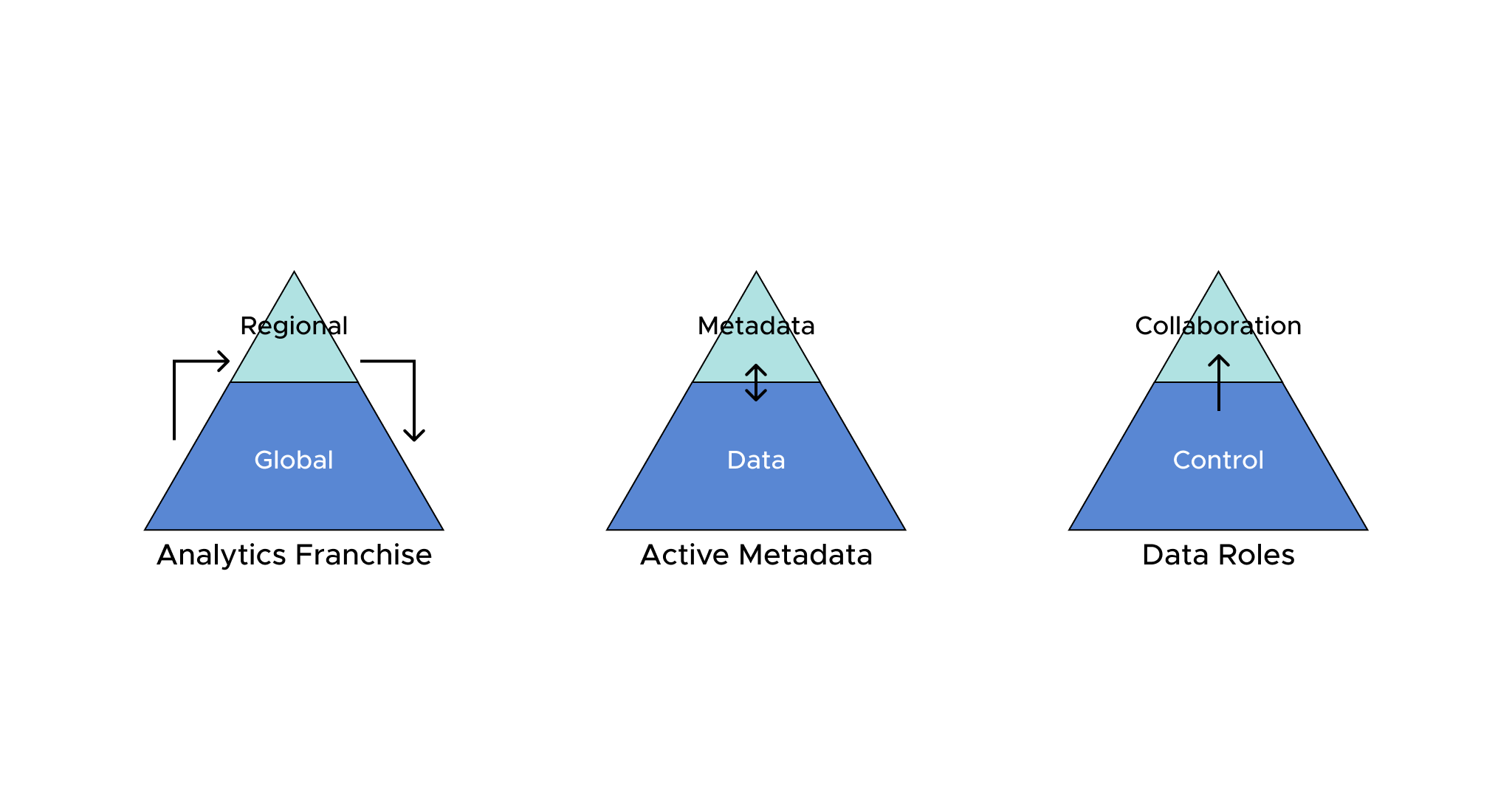
I was recently at the Gartner Data and Analytics Summit in Orlando. As a product leader, it was a great opportunity to meet with many chief data and analytics officers. I also had the privilege of chatting with many data and analytics users to understand their challenges, data and analytics needs, and priorities.
During the summit, I also had the chance to attend many of the sessions. As we go through a continuously challenging global environment, there is a lot of focus on balancing trust, accountability, governance, and security with adaptability and responsiveness. Another important topic covered was using AI ethically while managing data and privacy.
For me, the biggest takeaway by far was to focus on federated and collaborative governance to achieve data-driven business value. This helps ensure performance and cost optimization with cloud data management.
But there’s far more to modernizing data and analytics than that. Here are my top three strategies for improved data analytics and insights from the 2023 Gartner summit:
Data Analytics Strategy #1: Open a Data Analytics Franchise to Nurture a Data-Driven Culture
Kurt Schlegel and Rita Sallam shared this idea of “franchise:” Achieving better business value by using data and connecting data strategy to an organization’s critical priorities.
I really liked this franchise analogy. It demonstrates how to enable central consistency and governance while also enabling agility and flexibility. This ultimately helps achieve higher data and analytics adoption.
What is a “Data Franchise?”
A franchise is a way to bring a globally consistent way of doing things to a local market. The franchise has proven itself repeatedly as the ideal business model to scale sharing best practices.
This is exactly what organizations are trying to do with data and analytics. A franchise model supports data analytics strategies and these global consistencies that organizations need:
- Consistency of data definitions.
- Consistency of performance measure.
- Consistency of technology standards.
In addition to promoting that consistency, a franchise also has the ability to accommodate. It adapts to the regional variance the business units need. Where else might a need to accommodate come into play? Different departments, which sometimes need to define their own data definitions and calculate their own metrics. A franchise model is able to handle all of that.
I love the idea of global consistency and regional variance. In fact, this is what we at AtScale have been advocating for and enabling many enterprises to do already with the franchise approach. We’ve just been calling it by a different name: Hub-and-spoke.
The key to this approach lies in the semantic data model. The semantic model creates a digital twin of the business by translating bits and bytes into business terms. Then, domain experts can encode their business knowledge into digital form for others to use.
For this approach to work at scale, it’s critical to implement a common semantic layer platform that supports data model sharing, collaboration, and ownership. With a semantic layer, the central data team (the “hub”) can define common models and definitions (i.e., business calendar, product hierarchy, and organizational structure). Meanwhile, the domain experts in business units (the “spokes”) own and define their business process models (i.e., “shipping,” “billing,” and “marketing”).
With the ability to share model assets, business users can combine their models with models from other domain owners to create new mashups for answering deeper questions.
Learn more about the hub-and-spoke approach from AtScale CTO and Founder Dave Mariani.
Data Analytics Strategy #2: Use Active Metadata to Learn From Data Experience
Mark Beyer had a very interesting session on active metadata. I was intrigued by this session for a few reasons:
- I wanted to understand and validate the definition of active metadata.
- I wanted to validate how AtScale’s semantic engine is currently using active metadata’s unique value and evaluate our plans to innovate with it more.
But to investigate those ideas, we must first answer this question: What is active metadata?
According to Gartner, active metadata is the continuous analysis of all user, system, and infrastructure reports and data governance that enable alignment and exception cases between data and their actual experiences. In short, it is the data about the usage of the data. Active metadata shows how a dataset is used, what platforms it runs on, how well it succeeds, and how it travels through the organization.
For decades, we have been designing systems to collect data. Now, it’s time to observe how it’s used.
That’s what makes active metadata such an exciting area with tons of innovative possibilities. I have spent a good amount of my career in user experience design, and the idea of using active metadata for a better data experience takes my breath away!
By analyzing active metadata, we can:
- Determine data usage patterns
- Purge outdated and unused analytics artifacts
- Enrich the user’s analytics experience
- Build analytics content suggestion and recommendation systems
- Alert downstream end-users to resolve issues quickly
- Optimize analytics performance dynamically
Here’s the good news: AtScale’s universal semantic layer platform already captures active metadata. With its multi-BI and AI tool integration, AtScale’s semantic layer becomes the natural gateway for all data analytics strategies and insights consumption in an organization.
Our semantic engine monitors and knows about all an organization’s analytics queries. Plus, the semantic engine uses graph analytics to analyze the patterns in data usage. That’s how our semantic layer optimizes the analytics experience performance – by creating usage-based data aggregations at the cloud data warehouse.
This is but one of the innovative developments we have accomplished for “data experience.” If you want to learn more about what AtScale’s doing here, join me for Tapping the Enormous Value of Your Active Metadata at the Semantic Layer Summit on April 26th.
Data Analytics Strategy #3: Change Data Roles from Control to Collaboration
At the Gartner summit, there was a huge focus on “people.” This just makes sense. After all, people are the ones accelerating data and analytics success in organizations.
Being successful in data and analytics is just as much about tools and technologies as it is about people. That’s why when it comes to data and analytics, it’s time to shift data analytics strategies from control to collaboration.
Why must this shift happen? Because we now have new “versions” of data in organizations. Traditionally, teams focused on the single version of the data with the curated data supporting the dashboarding and analytics needs. Now, we need to think about the contextual version of the data, the next version of the data, and finally, the automated version of the data. Jorgen Heizenberg has done a great job explaining these different versions in his session.
Contextual versions of data support business processes like finance analytics, marketing analytics, supply chain analytics, and HR analytics. It melds data discovery with business process data.
The next version of data is all about innovation. It supports data science and ML use cases, as well as new use cases like information product management.
Finally, the automated version of data is about the real-time data generated by machines, IoT, and automated processes.
This first version of the data — AKA, the single version — is not going to scale and support new business needs. That’s a problem, since organizations so far have been focusing on the single version of data with strict data governance. This version does not scale because the data and analytics needs are everywhere. Each department and business unit has its own initiatives, they would all like to use data and analytics to achieve a business outcome.
That’s where the semantic layer comes in. Our data tool plays an important role in supporting these new governance models (franchise, or hub-and-spoke), new versions of data, and new data personas. If they want to stay competitive, organizations must start thinking about new governance approaches, as well as ways to balance their resources and skill sets across these four needs.
Get started on your governance journey today and turn your whole team into data analysts with ease. See AtScale in action.
NEW BOOK