April 13, 2023
Actionable Data Insights for Improved Business Results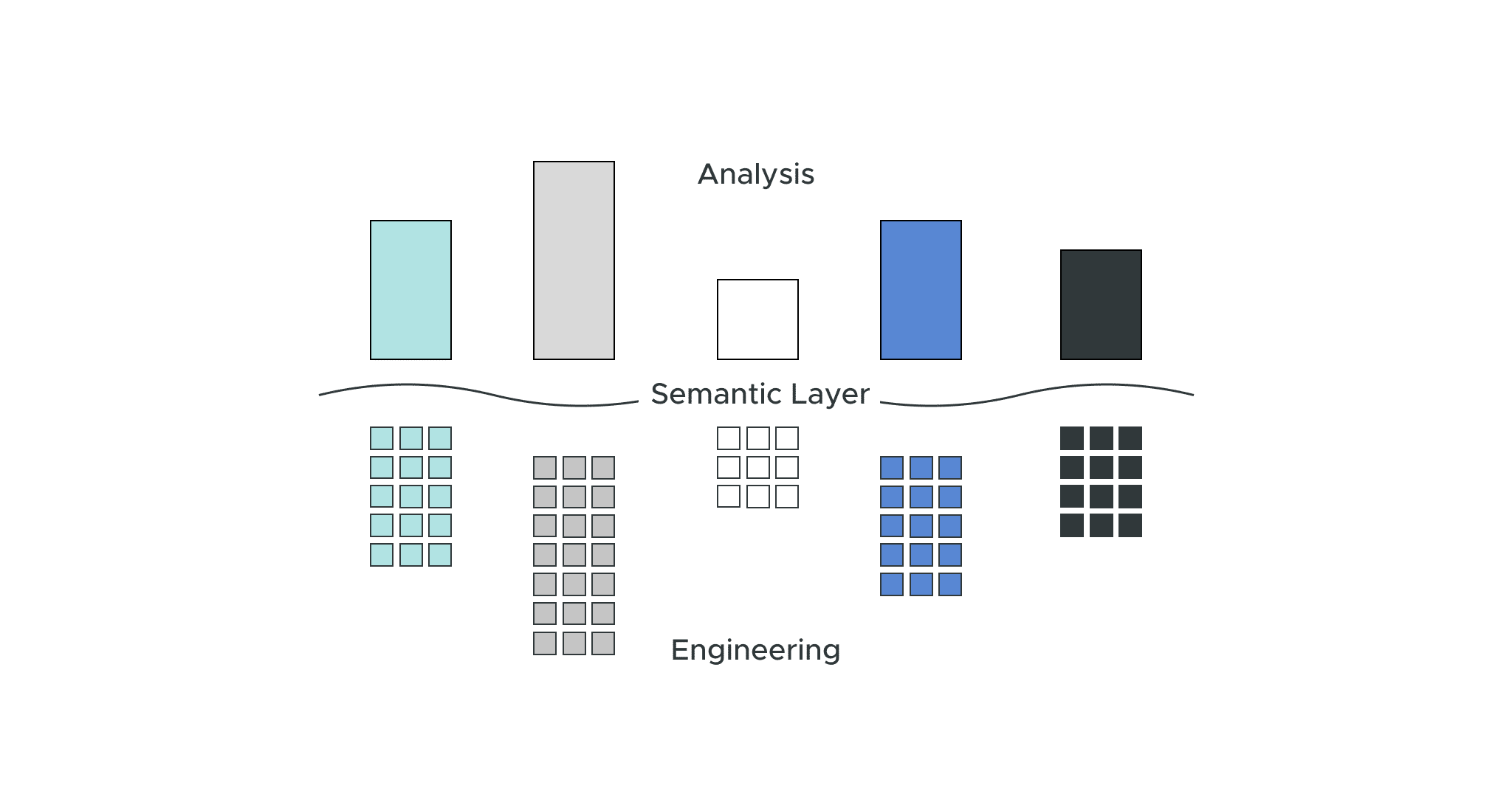
This post was written by Donald Farmer. Donald Farmer is a seasoned data and analytics strategist, with over 30 years of experience designing data and analytics products. He has led teams at Microsoft and Qlik Technologies and is now the Principal of TreeHive Strategy, where he advises software vendors, enterprises, and investors on data and advanced analytics strategy. In addition to his work at TreeHive, Donald serves as VP of Innovation and Research at Nobody Studios, a crowd-infused venture studio. Follow him on LinkedIn.
Why do many organizations still struggle to make data-driven decisions despite the abundance of data and the power of modern analytics tools? The answer often lies in the disconnect between two crucial roles, data engineers and business analysts, which leads to inefficiencies and missed opportunities.
In the past, when business intelligence was a priority, we often talked about the disconnect between IT and business users, which showed up as a tension between governance and self-service or between the centralization and distribution of effort. Today, the dynamics are different because so much work of all kinds is distributed while reporting or visualization is no longer a specialized practice. In the new enterprise, data engineers build robust data pipelines and create data products, while business analysts concentrate on developing insightful dashboards and analytics products. But there are other contrasts too…
- Different skill sets: data engineers have deep technical knowledge, while analysts focus on business domain expertise;
- Distinct priorities: engineers optimize for data infrastructure and costs, but analysts are looking for insights by value and impact;
- Organizational structures are too often siloed, limiting collaboration between these groups;
- We often lack a common language and understanding, partly due to varying knowledge levels and different skill sets and partly due to the piecemeal growth of initiatives across the organization;
- Of course, we use different toolsets: engineers leverage data integration and processing platforms while analysts prepare visualizations for complex analysis and dashboards for business presentations.
These differences can be creative and effective when well managed and supported, but too often, they result in misaligned priorities, inconsistent data definitions, and suboptimal decision-making. To get the most out of our organizations’ skills and techniques, we need a bridge between the fields of data engineering and business analysis.
Sometimes, this bridge is simply the human connection of people collaborating on a shared project. However, with distributed teams, remote and hybrid working practices, and increasing specialization, this is not enough. Or, at least, it’s not reliable or consistent enough to be strategic.
Semantic layers offer a promising solution to this challenge. By providing a common language for data and establishing standardized definitions and metrics, semantic layers can enable collaboration on data over distance and over time, improving data governance and innovation.
The question may not be whether to implement semantic layers but how to do so effectively. How can organizations identify the key business entities and relationships that form the foundation of their semantic layer? What processes should be put in place to ensure the ongoing maintenance and evolution of the semantic layer? And how can semantic layers be leveraged to unlock the full potential of data and analytics within an organization?
Another key concern is often overlooked. If the technology is to bridge between approaches and techniques, it cannot just impose one way of working on data stakeholders. Data engineers prefer to work with code, while business analysts gravitate toward non-code environments. This difference in approach can further widen the gap between the two roles, making effective collaboration and alignment even more challenging. So, the semantic layer must provide a unified – and unifying – platform that enables data engineers and business analysts to work in their preferred manner with their preferred tools while still ensuring a consistent and integrated data experience.
The semantic layer should offer a robust and flexible API that enables data engineers to programmatically access and manipulate data using their favorite coding languages and tools. This allows data engineers to incorporate the semantic layer into their existing data pipelines and workflows, ensuring integration with the broader data infrastructure.
On the other hand, business analysts should be able to interact with the semantic layer through intuitive, drag-and-drop interfaces and visual query builders. These non-code environments empower business analysts to explore, analyze, and visualize data without extensive technical expertise. By abstracting the underlying complexity, the semantic layer enables business analysts to focus on deriving insights and making data-driven decisions.
However, semantics’ true power is that it brings these worlds together. By providing a common foundation of standardized definitions, metrics, and relationships, the semantic layer ensures that data engineers and business analysts work with the same concepts and assumptions, regardless of their preferred working style.
Moreover, an effective semantic layer should foster collaboration and knowledge sharing between data engineers and business analysts. This can be achieved through shared workspaces, version control, and annotation capabilities, allowing both roles to contribute their expertise and insights to the evolving semantic layer.
An effective semantic layer bridges the gap between data engineers and business analysts and unlocks the full potential of data and analytics within an organization by supporting both code and non-code preferences. It enables data engineers to build robust and scalable data infrastructures while empowering business analysts to derive actionable insights and drive business value.
To effectively implement semantic layers in startups, stakeholders and domain experts must be involved in identifying key business entities. The data model should evolve with the business, and a flexible yet robust governance framework should be established for ongoing maintenance. The semantic layer can be leveraged to democratize data access, enhancing cross-functional collaboration and data-driven decision-making. By prioritizing data quality and aligning the semantic layer with evolving business needs, organizations can accelerate their time to insight and unlock new business opportunities. They are built on a solid data governance and compliance foundation crucial for scalable, sustainable growth.
>> Learn more practical advice and best practices from top data leaders and technologists on how to implement a semantic layer at this year’s Semantic Layer Summit, a free one-day virtual event on April 24, 2024.
ANALYST REPORT