April 13, 2023
Actionable Data Insights for Improved Business Results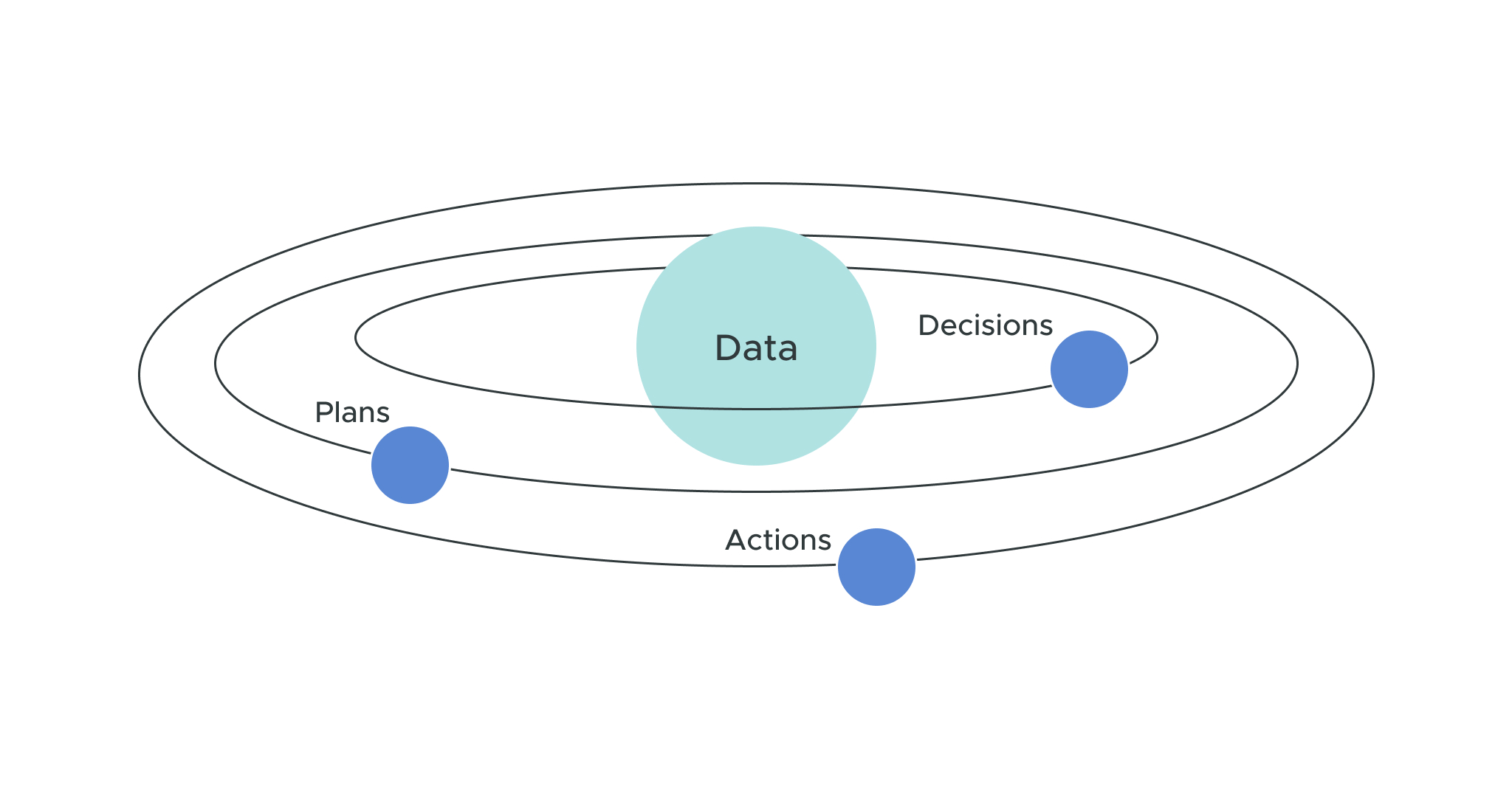
Many companies pursuing a path to data-driven decision-making – accelerated through data democratization – struggle with how to define and support the core people, roles, and competencies involved with data (data creators, enablers, and consumers).
In many cases, the path to data democratization is hampered by the inability to make actionable data, insights, and analytics available to decision makers because the core competencies, capabilities and processes are:
- Not mature enough (not capable)
- Don’t deliver fast enough (slow speed-to-insights)
- Don’t scale enough (limited capacity due to manual processes and resources) to support the creators and consumers of insights and analytics
This blog discusses the catalysts, opportunities, and challenges to delivering actionable insights through data democratization. This includes defining the core principles, key roles and desired capabilities necessary to develop and deliver actionable, impactful insights and analytics with sufficient speed, consistency and scale.
Fundamentally, enterprises must possess a data-driven decision making culture. This involves focusing on centralized data management to support de-centralized data democratization. By managing data as a product – with key roles and competencies, and capabilities provided – it’s possible to deliver increased insights automation, alignment and advancement
Foresight over Hindsight
Reaping the benefits from utilizing modern data platforms to deliver timely, actionable data, insights and analytics (DIA) for the enterprise requires establishing well-defined strategies, capabilities and priorities. These should be aligned to targeted opportunities, intended performance, scale and maturity. The promise is to deliver actionable insights – focusing more on foresight than hindsight – through modern data platforms that improve the confidence and clarity of the answers we seek, the decisions we make, and the actions we take.
Developing and delivering actionable insights requires five core components: Data-Driven Culture, Modern Data Platforms, Data Strategy, Data Product Management and Data Democratization
These components need to be aligned to create and distribute data as a product (e.g. data products) through centralized data management, enabling de-centralized, democratized insights and analytics creation and consumption at scale.
Components of a Successful Insights Program
Data Driven Culture | Modern Data Platforms | Data Strategy | Data Product Management | Democratize Data |
Data as a Core Strategic Asset | Cloud-based Data Platforms | Data Strategy and Agenda | Managing Data as a Product | Decentralize value creation |
Data-Driven Decision Culture: Creating the Catalyst for Data Democratization
Organizations that possess a data-driven decision culture view data, insights and analytics as a core strategic capability. Fundamentally, a data-driven decision culture is one that believes in the business advantages of using data to improve understanding and decision-making. For many of these organizations, the primary use case for data is to measure performance and feedback.
Data-driven cultures understand that markets, customers, and operations operate as “systems of actions and interactions”, and that data is the “fuel” for understanding how these systems behave. This includes how their enterprise as a system performs with a focus on understanding the drivers of performance: marketplace demand, customer experience or satisfaction, and operations effectiveness and efficiency.
Establishing a Data-Driven Decision Culture:
Data as “Fuel” for Powering Systems and Performance
Fundamental Principles
Data as Fuel | Measure to Improve | Systems Thinking | Performance Drivers | People Competencies |
Data Fuels Operations and Decisions | Performance Feedback is Measured | View Markets and Operations as Systems | Determine Drivers of Performance | Develop Core Competencies among People |
A data-driven culture adds data as a new dimension to complement existing core organization capabilities represented by people, process and technology. Data represents how organizations improve using data as the fuel for planning, acting and refining. Delivering data-driven decision culture means identifying and supporting three key roles within the organization: consumers (decision makers), creators (data analysts, statisticians and scientists) and enablers (data engineers, architects, modelers and platform engineers).
Establishing a Data-Driven Decision Culture:
Placing Data at the Heart of the Enterprise Business Model
Data as the Fourth Dimension of Transformation
Supporting Data, Insights and Analytics Consumers, Creators and Enablers
Defining a Data-Driven Decision Culture
What does it mean to be a data-driven enterprise? Fundamentally, a data-driven enterprise is one where data is at the heart of all plans, decisions, and actions. A data-driven culture understands the value of data, relies upon it, and quantifies the benefit of using it. There is also ownership by the business and joint accountability shared among leaders and team members from business, technology and data science. Key elements of being a data-driven decision enterprise are as follows:
1. Data and Analytics as Strategic Assets – Rather than viewing data and analytics as simply an operational capability or contained within a broader initiative like digital transformation, being data-driven means that data, insights and analytics are viewed as strategic assets that represent a better way to plan, decide, and act.
2. Data Literacy – Data-driven organizations are literate about what data is and how it is created, used and scaled. Data literate cultures understand and support the three key roles of data consumers, creators, and enablers:
- Consumers – Those who consume data, insights, and analytics, most often with the purpose to provide plans, make recommendations and decisions, take action, or prepare for remediation or innovation.
- Creators – Those who prepare and present relevant insights and analytics to consumers. Often these roles involve business and data analysts, statisticians, and data scientists.
- Enablers – Those who support making data, insights, and analytics available, accurate, operational and scalable. These roles often include technology professionals, such as data engineers, analytics engineers, BI engineers, data platform engineers, data architects, and data modelers.
3. Analytical Mindset – Given that data-driven decision organizations are driven to use data, insights and analytics to make better plans, decisions, and actions, it’s crucial that those who consume insights and analytics have an analytical mindset. An analytical mindset means understanding what it means to use data to avoid information and decision bias, and how to use data effectively to enable more rapid decision making. This requires greater clarity, confidence and alignment among executives and teams. Understanding and avoiding decision bias is crucial.
4. Maturation and Scale – Data-driven enterprises concentrate on making sure they have the most relevant and effective strategies, capabilities, and roadmap that delivers improvement in business impact, capability and scale.
Many companies are now reaching a point where they’ve proven the value of data, insights and analytics. They’ve invested in modern data platforms and tools and hired or developed effective talent, but find themselves unable to scale to support many use cases while supporting increased need for new data, insights and analytics. Achieving effective scale involves embracing the following:
- Simplification – Preparing data, insights and analytics is a multi-step process involving as many as seven steps that can involve multiple resources and hand-offs. Efforts to streamline the production process steps reduce data movement, reduce data transformation and reuse integrated data sets, including enabling versions without operational maintenance are super important.
- Automation – In addition to simplification, automating data access, accuracy, transformation, integration, aggregation and publishing of data, insights and analytics is crucial to achieve speed, capacity and productivity.
- Alignment and Governance – Implementing effective data governance and providing centralized data definitions for metrics and data models so that all users are sharing one definition of metrics and datasets.
- Optimization – As data movement and query volumes increase to serve the organization’s need for smarter, faster data, insights and analytics, organizations must look to optimize data movement and query speed. Doing so will increase capacity, delivery and reduce cost.
The Recipe for Delivering Actionable Insights: The 3 D’s of Actionable Insights – Data Driven Culture + Data Product Management + Data Democratization
We’ve already discussed the importance of creating a data-driven culture and what it means to provide the core capabilities necessary for turning data into actionable insights. In addition to implementing a data-driven decision culture, enterprises must also embrace delivering data managed as a product enabled through data democratization. The fundamental approach to achieve productive, impactful scale is to centralize data management while decentralizing value creation and actionable insights.
- Centralize Data Management – Manage Data as a Product
- Decentralize Insights and Analytics Creation – Enable Data Democratization
1. A data-driven decision culture establishes the overarching mindset, capabilities, and processes for creating and consuming actionable insights.
2. Data product management is the ability to answer business questions more effectively using data, insights, and analytics. A data product is essentially a dataset that is purpose built to deliver actionable insights for a specific domain or across domains at the enterprise level. Data Products are a self-contained set of available data, insights, and analytics that can be easily created, distributed, consumed, and refined by both business and technical teams. Key elements of a data product are as follows:
-
- Defined purpose – answering defined business questions
- Defined integrated data model and physical dataset with sources, structure, and queries pre-defined and available for immediate use
- Defined outputs – reports, visualizations, analytics, explanations
- Aligned with enterprise standards for business-defined metrics and views
- Automated to enable rapid, resourceless access to underlying sources, transformations, enhancements and consumption
- Governed to manage permissions for access, usage, and modification
We will cover more details on the definition and management of data products in future blogs and publications. Managing Data as a Product is critical to transforming disparate data sources into actionable insights.
3. Data Democratization – Data democratization is the ability for data creators and enablers, particularly non-technical domain experts, to easily and rapidly create, share, publish, and refine data products using self-service tools. Data democratization is supported by an effective, efficient set of centralized services, which enables rapid data access, computation, distribution o publishing, and governance.
Enabling Data Democratization: De-centralized Value Creation from Data
Delivering actionable insights at scale, offering each business domain the combination of centralized enterprise insights with domain-specific insights requires the ability to enable domain-specific data democratization. Domain-specific data democratization requires an effective strategy for defining, supporting, and deploying people, skills, processes, alignment and support. Key elements of domain-specific data democratization are as follows:
- Clarify Roles and Responsibilities – Consumers, Creators, Enablers
- Create Enterprise Data Analytics Skills Matrix with levels for training plan
- Create standard process for creating and consuming actionable insights
- Establish enterprise change management team to support transformation
- Seek executive support from key functional leads who sponsor use cases
Enabling Data Democratization: Key Elements
Roles and Responsibilities |
Skills | Standardize Processes | Change Management | Executive Sponsorship |
Consumers, Creators, Enablers | Skills Matrix and Training Curriculum | Data Product Management Process | Implement Change Management | Executive and Function Lead Support |
Actionable Insights at the Center of People, Process and Technology |
Identify and Define Key Roles – Identify the responsibilities for key roles, segmenting into major groups such as insights “consumers”, “creators” and “enablers”. Discuss how each role should work together so that an effective, repeatable way of working framework can be created, deployed and refined.
Create an Enterprise Data Skills Matrix and Training Plan – Identify and align on the necessary capabilities and skills based on a core, common set of capabilities. Then assign importance of each skill to each role as a group, and for specific types of positions (e.g. decision maker, data analyst, data engineer) focus on the combination of business, analytical and technical skills. Many companies also provide a set of core learning curriculum to encourage building an “analytical mindset”, focusing on the importance of using data and analytics to answer key questions about performance and improvement (e.g. feedback). This includes understanding and addressing decision bias, and the role data plays in helping us avoid bias and achieve clarity, focus and alignment in our understanding, deciding and acting.
Major Types of Data and Analytics Skills: Business and Technical
Business Context | Data Preparation | Analysis & Synthesis | Understand, Decide, Act | Monitor Performance | Create Analytical Models |
Technical Skills – Data Architecture, Engineering and Operations |
Create Standard Processes for Data, Insights and Analytics – Establishing standardized processes for how data, insights, and analytics are created is crucial to achieving speed, scale, productivity, including insights relevance and action-ability. Process design also helps to ensure that those being served are aware of the steps, resources, and time required to instantiate new data sets, create insights and analytics, etc. Process designs should address key data and analytics activities, including new data source assessments and sources, data and insights access, database enhancements, EDW and Information Mart enhancements, data platform enhancements, data integrations, analytics and new capability assessments.
Establish an Enterprise Change Management team – Change management is critical to ensuring that the new platforms, processes, capabilities, talent and skills are being utilized effectively. Establishing a data and analytics change management team provides the support necessary to ensure that the entire organization is supporting the transformation to be smarter and faster. This includes enterprise communication, skills and training, recruiting and data, and analytics center of excellence (COE) organization and deployment.
Seek Executive Support from C Suite and Key Functional leads – The success of any major enterprise capability transformation requires transparent commitment from the C-suite and key functional leads, and the transformation to being smarter, faster through data and analytics is no exception. Perhaps more so, since for many organizations that face the need to embrace digital transformation, data and analytics transformation is just as critical. In fact, many organizations that undertake digital transformation also have a team addressing data and analytics transformation, as the two transformations compliment each other. Organizations that take a “wait and see if it works” (vs knowing that it works if done well) mentality will find that they will either fail to realize the benefits of being smarter, faster or simply continue to do what they are already doing by pursuing small incremental improvements. Making the commitment to being smarter, faster means making a change to embrace modern data and analytics platforms, capabilities, and processes and utilizing those capabilities to address strategic enterprise opportunities more effectively.
AtScale Semantic Layer: Enabling Actionable Insights for Everyone
AtScale provides a Semantic Layer, which sits between the Data Source Layer and the Insights Consumption Layer (e.g., AI, BI and Applications). The Semantic Layer converts data into actionable insights via Automation (self-service data access, preparation, modeling, and publishing), Alignment (centralized data product management and governance with a single, consistent metric store) and Acceleration (cloud analytics optimization – BI query speed optimization, multidimensional OLAP in the cloud, AI-based data connectors, and automated PDM tuning). This supports insights and analytics creators, enablers and consumers without requiring data movement, coding, or waiting.
AtScale Semantic Layer
Enabling Actionable Insights for Everyone
Providing Automation + Alignment + Advancement
With No Data Movement, No Coding and No Waiting
Automation | Alignment | Advancement |
Self-service data access, preparation, modeling, publishing for AI & BI | Centralized Data Product Management with Single Enterprise Metric Store | 10X Increase in Query Performance, Automated Tuning, Cloud OLAP |
ANALYST REPORT