August 7, 2019
Five Areas Where Intelligent Data Virtualization Gives Retailers a Competitive Edge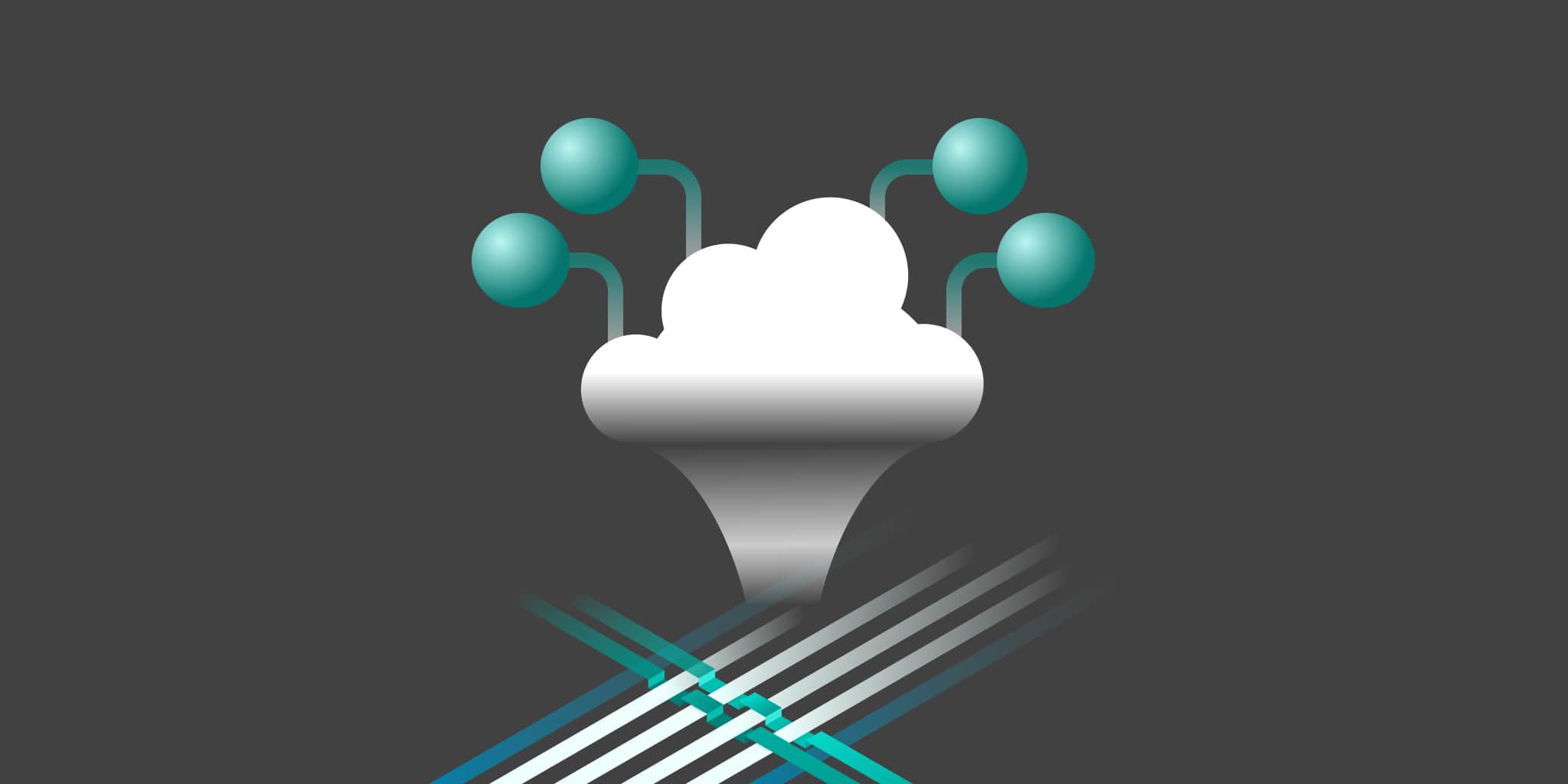
An adaptive analytics fabric has the following four capabilities when bridging the gap between users and data:
1 – Bring all data (silos) to users’ fingertips
IT organizations today have common server orchestration tools to manage their on-premises and cloud server resources. Universal data orchestration tools are needed to automate data engineering while providing a comprehensive view of analytical data. Data virtualization must provide users with uniform visibility to all the data in an enterprise’s diverse analytical repositories. Regardless of the location, configuration, formatting or technology storing the data, data is presented in a fashion that is immediately ready to query and combine with other data. Users need only connect to the virtualized data to access any analytical data they have entitlements to work with.
An analytics fabric provides a kind of “hybrid intelligence” that brings portability and flexibility for data sources. If a siloed data source must remain in place due to a legacy system, users can still access it. If an on-premise data source is migrated to the cloud, users can still access it. Virtualization smooths out the bumps in migration to the cloud and even between clouds because the location of the data source no longer limits the end user.
2 – Manage security and data governance across all users and data sources
The enterprise must preserve information about which databases are the “system of record” or maintain the authoritative record for each element of data, what changes were made and by whom. Data virtualization must have the ability to respect the entitlements of individual users on different data sources and carry those entitlements through to data structures that are materialized from a larger corpus of data or combined from multiple sources. Further, entitlements must be enforced within the analytical applications users leverage to access data through a connection pool. Finally, a data virtualization solution should have mechanisms for promoting data quality and managing how data is joined when working with multiple fact tables to ensure like data is matched appropriately.
The immense advantage of virtualizing data for security and data governance is that all queries and results pass through autonomous data engineering. Rather than a many-to-many relationship between users and data, enterprises employing intelligent virtualization will now see a many-to-one-to-many relationship. This enables the virtualization tool to act as an enforcer of security rules and data governance.
3 – Represent multiple data sources as one
Intelligent data virtualization provides a single, comprehensive view of the enterprise’s underlying data platforms, presenting a “single table” view of data for consumption by BI and AI/ML applications. Enterprise data warehouse virtualization further breaks down data silos, makes data easier to understand as a unified entity and fosters more comprehensive analysis.
4 – Interface with any major BI tool
Business users, analysts and data scientists will come from disparate backgrounds and will have individual preferences for the BI tools they use when working with data. Rather than struggling to bend all users to a single standard for BI software, virtualizing data and enabling virtual cubes and models ensures that queries will return consistent answers across different tools. BI tools vary in how they query data, often due to different dialects of query language such as MDX, MySQL, PostgreSQL and so on, so an enterprise data virtualization solution will need to support query translation for many solutions. This delivers the flexibility to use the preferred BI tool of each user so the enterprise can focus on the data.
Let’s look at two examples of intelligent data virtualization in action.
Example 1 – Migrating Data from On-Premises to Cloud Without Disruption
A global home improvement retailer needed to modernize its Hadoop solution, in use by 4,000 stores. Hadoop had proven more challenging to manage and too expensive, so they opted to move to the cloud with Google BigQuery (GBQ). Also, users were comfortable with Microsoft Excel, and IT did not want to introduce a new BI tool, so any new solution would need to work well with Excel.
The migration from Hadoop to GBQ was completed over a weekend without any disruption to the business users. On Monday morning users were able to query the same reports without noticing any difference other than faster response times. AtScale empowered the retailer by providing fast, easy access to 2.5 years of data, refreshed every 20 minutes through its platform in conjunction with Excel and GBQ. Store managers could now not only see their store, but could also benchmark against other stores within and across regions using AtScale. Users retained the ability to use Excel and avoided costly and time consuming re-training efforts.
Example 2 – Democratizing Access to Global Users
A global chemical producer was looking to give over 15,000 employees access to business data that was centralized, organized and accurate. The company generated petabytes of data that was getting siloed in disparate databases. BI tool users wishing to analyze data across multiple sources were forced to download copies of data and create their own local databases. The result was millions of downloads annually into local storage. The company needed a way for employees to work with disparate data sets, minimize extracts from central data repositories to local machines and optimize the quality and accuracy of data.
AtScale was implemented to connect disparate data together and model that data into virtual cubes that employees could connect to using their preferred BI tool. Employees could now find trusted data in a central, globally-accessible location. AtScale’s A3 was able to connect disparate data in a data lake to form virtual cubes created a single source of truth (SSOT) that could be used online by employees globally. AtScale has driven huge efficiencies and has eliminated millions of redundant, divergent copies of data. The near elimination of database extracts greatly reduced security threats related to the copying and storing data on local networks and computers.
Creating a Shared Data Intellect
All cloud migration efforts must serve business drivers such as reducing costs or increasing revenue. It can be easy to become distracted by the short-term technical benefits of cloud solutions, but to accept such distraction would inevitably cause the enterprise to stop short of realizing the ultimate benefits of the cloud.
A data virtualization solution that helps enterprises de-emphasize technology so that business users can have simple, intuitive access to as much accurate data as possible, is the best solution for far-seeing companies. Enterprises leveraging data virtualization will be able to remove all the technical hurdles of disparate data sources and focus employee effort not on tending the garden, but on maximizing the value extracted from the company’s data and creating a shared data intellect and enjoying the fruits of their labor.
For more on this topic, download our white paper Cloud Transformation: The Next Virtualized Data Frontier for Business Intelligence, Machine Learning, and AI.
The Practical Guide to Using a Semantic Layer for Data & Analytics