December 15, 2022
How to Maximize the Value of AI and BI at Scale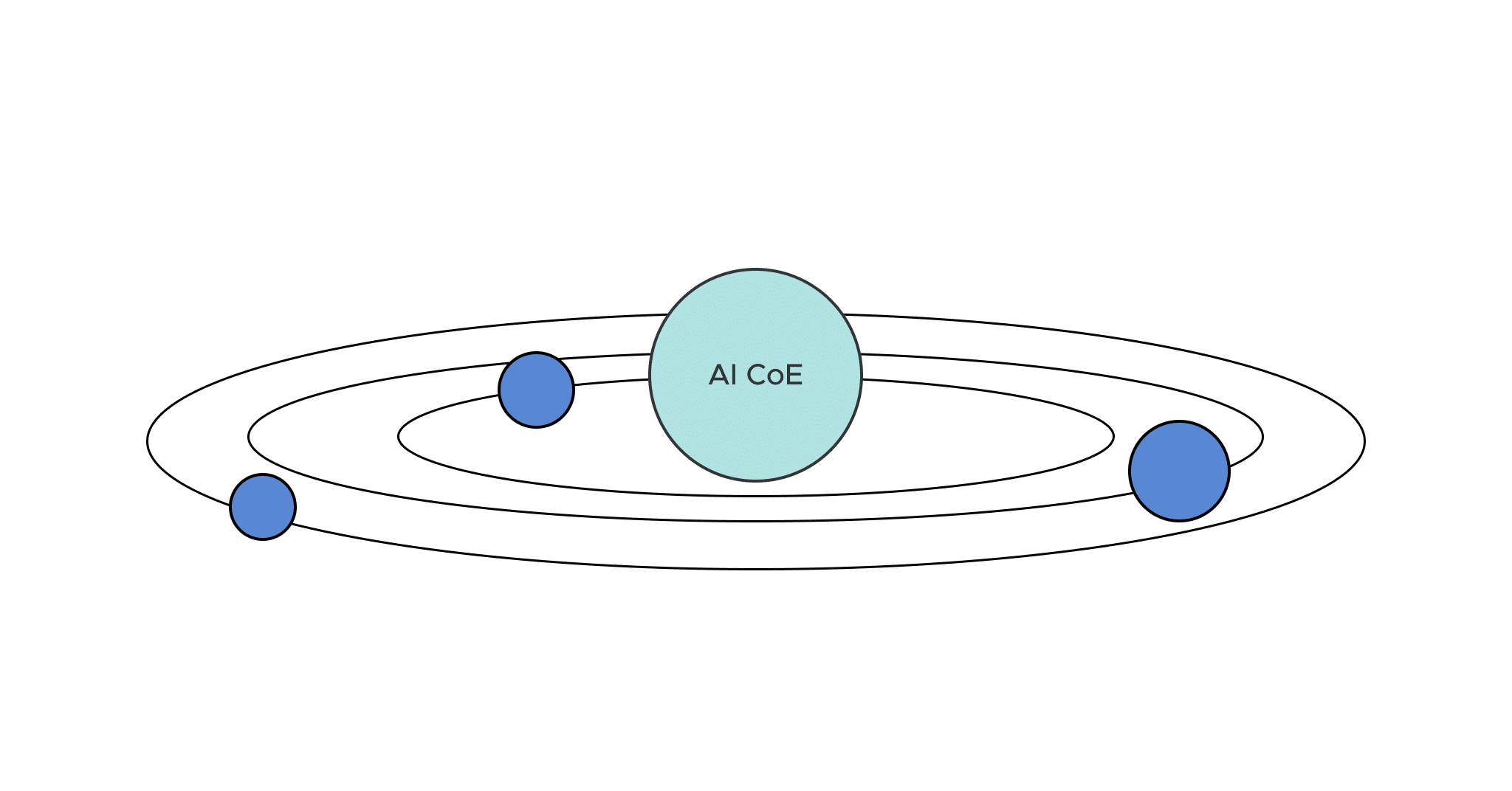
This blog is part of a series from Vin Vashishta, Founder and Technical Advisor with V Squared. V Squared advises businesses on AI strategy, data product strategy, transformation, and data organizational build-out services. They have helped clients deliver products with $100M+ in ARR and built small (5 data professionals) to large (30 professionals) D&A organizations. V Squared clients include Airbus, Siemens, Walmart, JPMC, as well as a wide range of smaller to medium businesses. Follow Vin on LinkedIn, Twitter, YouTube, and his website.
—
The AI Center of Excellence (AI CoE) model is modern business’ preferred organizational structure for several reasons.
- The total cost of data science initiatives is reduced.
- Data team productivity improves, and AI scales to handle more use cases.
- CoEs promote data and model literacy across the business.
- Data science workflows and tools are standardized.
- Innovation initiatives are better supported and prioritized.
Companies like Meta provide a roadmap for how AI CoEs are implemented and mature in Big Tech. They centralized AI talent, resources, and infrastructure under a data organizational umbrella. Meta succeeded in becoming an AI-first business and deploying model-supported products throughout the enterprise.
This summer, they announced a reorganization to decentralize. Once the CoE’s objectives are achieved, dedicated resources embedded in the organizations and product teams they support can improve the business value they deliver. Meta and other businesses went through a maturity journey from scattered resources to centralization to decentralization.
However, your business probably isn’t building a platform to support a world filled with floating avatars and digital monkey art. The arc is the same across business types, but the focus is much different.
What Are the Benefits of Transitioning to a CoE?
Organizational structure should support transformations. CoEs make the early and mid-stage data maturity journey more efficient. Here are the main business problems this model solves:
Duplication Increases Costs
In early-maturity businesses, infrastructure is the biggest source of duplication. Data is managed with different applications in different business units. The same data is often gathered with multiple pipelines to accommodate enterprise-wide needs. The result is higher data storage costs, levels of effort, and time to insight.
Ownership
A CoE centralizes ownership for all the infrastructure required to build and deploy data and model-supported products. Infrastructure consolidation and standardization are possible with a single organizational owner serving the rest of the business. The fragmentation comes from every business unit being forced to service its own needs.
Workflow Organization
Data talent scattered across the business makes it difficult to standardize data science workflows. Data scientists don’t learn from each other and improve as a cohesive unit unless they are part of a single hierarchy. Best practices that improve model reliability rarely take hold because there’s no central leadership driving those initiatives.
There’s duplication here too. When separated into different groups, data scientists and analysts use different tools. That makes hiring or moving resources from one project to another difficult. Code and models are harder to maintain. The lack of consistency also results in different frameworks being built to handle data product integrations for each team.
A CoE puts data science experts in leadership roles. They standardize tools, workflows, and best practices. Consistency extends to data and model literacy training. Uniform self-service tools are bought and purpose-built. The CoE becomes a single source for data capabilities and products.
That’s the technical side of the equation. Alone, a CoE model doesn’t guarantee business value. There’s another side of the CoE transformation that most Big Tech companies don’t address.
Best Practices for AI CoE Models Supporting Traditional Strategic Objectives
I align the maturity journey with traditional business drivers instead of technology. Businesses need to mature along a strategic arc vs. a technology arc. I call this the Continuous Transformation Framework (CTF).
- Legacy Business
- Competitive Maintenance
- Competitive Advantage
- Innovator and Industry Leader
Not every company chooses to mature to phase four. This journey framework makes it easier to decide how far the business should go. Big Tech goes to phase four without a second thought, but most companies should carefully consider strategic goals, customer needs, and competitive forces.
The objective of establishing an AI CoE is to compress the timeline and reduce the costs of maturity. Here’s how the journey plays out from an investment and returns POV.
Transitioning from Legacy Business to Competitive Maintenance requires an initial investment. CoEs are the most efficient way to get to break even. At the Competitive Advantage stage, data and AI become a source of growth. That requires scaling to handle more use cases, and again, the CoE is the most efficient model.
Innovators generate significant returns from data and AI efforts. Those technologies become the business’s primary growth drivers. At this phase, the challenge is different. The firm must improve new KPIs. The first is the percentage of innovation initiatives that result in a product. Second is the average revenue per innovation initiative.
The CoE allows an innovation mindset to form and take hold across the business. That shift won’t happen when data resources are distributed. Business units and product teams are laser-focused on the near term, but innovation requires a long-term perspective. Innovation also requires a high tolerance for failure that isn’t present outside a CoE.
Once innovation becomes a core competency, it should be optimized for value creation. That’s the driver for decentralizing the team. The closer data professionals are to the users and customers they support, the more closely aligned innovation initiatives are with value creation.
What Does an AI CoE Do To Support the Business?
The CoE model focuses attention on the data organization, infrastructure, and innovation. What’s overlooked is the rest of the business. The CTF makes it obvious that data, AI, and machine learning will touch every part of the business. Transformation must happen within the CoE but also outside of it. The CTF shows the complete journey and highlights the need to prepare for the CoE’s eventual decentralization.
When the business doesn’t see the big picture, over-centralization results. The data organization takes on too much and does work that other organizations can do more effectively. For example, opportunity discovery must be strategy-driven vs. technology-driven.
With the CoE model, initiatives are often chosen because the technology enables them. The data organization discovers an opportunity and presents it to senior leadership. While that sounds like the right approach, the result is technology driving strategy. It should be the other way around.
Opportunity discovery is a C-level workflow. The CDO should be involved. They can bring forward opportunities, and the executive team can decide which to move forward. The decision is based on top-level strategy, goals, needs, and competitive advantages, so every business unit should be represented.
The conversation here moves away from answering the question ‘What could we do?’ and evaluates ‘What should we do?’
The data team in a CoE model can become the driver for use case selection and prioritization. Both are better managed by external teams. Front-line workers can surface use cases better than most data teams. Product managers have the right skills mix to estimate and prioritize those use cases.
Keeping activities like these in teams outside the CoE prepares the business for eventual decentralization. Knowledge should flow from the CoE to other parts of the company. Domain expertise, customer needs, and opportunities should flow into the CoE. It’s not only an excellent long-term strategy, but it also prevents a silo from forming around the CoE.
Business-ready Data and AI
The data and AI monetization journey needs a CoE model for early and intermediate steps. The vision for AI must be strategy and value-first. Companies like Meta and Google innovate first and look for ways to monetize second. Outside of Big Tech, innovation must be driven by value.
Technology and talent are significant pieces of monetization. The CoE model and organizational structure fast-track the technical maturity process. The CTF aligns business transformation with data and AI maturity.
The business must be ready to adopt and monetize. After all, the ROI of models no one uses is still zero, no matter how amazing the technology behind it is.
RESEARCH REPORT