June 10, 2021
AtScale in Action: Scaling Self-Serve BI Program on Snowflake With a Semantic Layer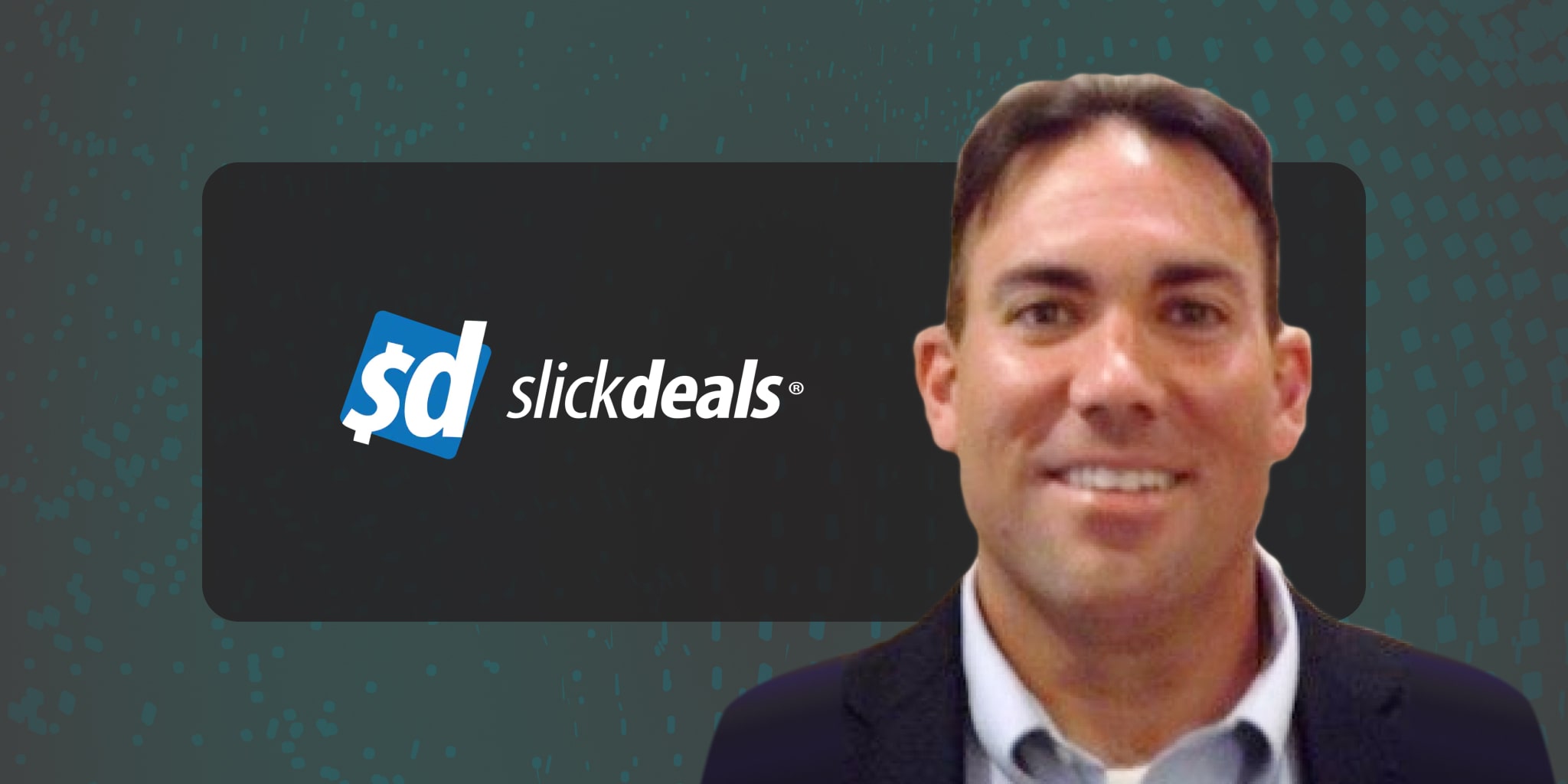
Recently, we hosted a webinar with Greg Mabrito, Director of Data and Analytics at Slickdeals, a user-driven deal-sharing site enabling consumers to collaborate and share info to make savvier online shopping decisions. In the session, which you can watch in full here, Greg took a deep dive into how his team at Slickdeals has upleveled its approach to self-service business intelligence (BI) with data analytics over the years.
To give you a sense of why Slickdeals’ approach to data is well worth paying attention to, it’s helpful to understand the sheer scale of their enterprise.
With more than one billion visits annually, Slickdeals is the number one external referral source for the majority of the internet’s top retailers, including Amazon, eBay and Walmart. They see 12 million monthly unique users and have saved users more than $6.8 billion dollars. The company offers desktop and mobile apps, as well as a browser extension, emails, and more. Perhaps most impressively, they handle all of this volume with only two data engineers, two data analysts, and Greg leading the charge.
The data team’s primary goal has always been to let people use whichever tools they prefer while empowering them to get the answers they need. Applying a universal semantic layer from AtScale accelerated the time to insight from data, regardless of its underlying infrastructure. Here’s how extracting insights became a self-service process for Slickdeals employees.
Slickdeals and business intelligence: A quick history
Slickdeals kicked off their BI program with a single, on-premise server in 2012, using Excel as the main end-user interface. By 2014, the team needed to make adjustments to their hardware and capacity in order to improve performance and user experience. They did this by separating their cube processing from their database by moving their cubes to a single SQL Server Analysis Services (SSAS) box.
Come 2016, they needed to grow that box and add Tableau to the mix for visualization. They also added Wherescape to orchestrate between SQL Server and help the team move data around more seamlessly. In 2018, the SQL Server hardware had to be upgraded yet again—a constant theme over the years. As their data volume increased, the team worked hard to keep up.
By 2019, they had pushed to the very limits of SSAS. Users were often dragging massive amounts of data into pivot tables and user experience took a rapid nosedive. It was time to rethink the system altogether.
In 2020, Slickdeals built out a whole new architecture using Snowflake, AtScale, visualization with Tableau and Excel, and Wherescape to integrate the various components, plus Kafka to improve data latency. They also refactored data pipelines to be event-driven. Rather than approaching this transformation as a simple “lift-and-shift” to the cloud, they recognized an opportunity to overhaul and modernize their entire approach to data analysis.
The 7 big barriers to self-service business intelligence
Last year, the team at Slickdeals committed to major changes to improve the architecture and outcomes of their data stack. Here are the seven big challenges they tackled—challenges many rapidly scaling businesses have faced or will face in the quest to enable self-service BI at scale.
1. Unscalable Infrastructure
The team at Slickdeals realized they needed—and lacked—a scalable data infrastructure. Serial processing of data pipelines and “one and done” approaches were painful. This monolithic, heavy data movement approach also resulted in incohesive and stale data.
2. Semi-Structured Data
The team deals with a lot of semi-structured data. Ingesting deal information from the internet and brokers meant loading up that unstructured data as JSON objects and running scripts to flatten and process it. This approach couldn’t scale with their incoming data velocity.
3. Data Movement Constraints
Data movement previously took hours—sometimes as many as 10+—on a weekly basis. This was a maintenance nightmare and meant cube builds were fragile. Challenges could easily spill over and leave the team without access to data.
4. Tight Coupling of Components
Before, all of their cubes and data pipelines relied heavily on shared resources across the environment. This led to conflicts and issues when the systems were pushed to their limits. Ultimately, it resulted in a bad user experience and a lack of data-driven insights. They needed to develop a data strategy that allowed for loose coupling.
5. Lack of Agility and Speed
The team found they were lacking the ability to iterate and move quickly in a presentation layer. Often it would take hours to build a cube, model it, and deploy it, which is not acceptable given the pace of business at Slickdeals. They have a very sharp development team, and the data team itself could move quickly, but the tools were dragging them down.
6. Small Data Team, Big Needs
As mentioned earlier, the Slickdeals data team has long been on a mission to enable self-service BI. The data team is just four people, but the larger organization comprises 150 employees, almost all of whom need to be able to generate data-driven insights, fast, to do their jobs effectively.
7. Data Volume Growth
As with most businesses, Slickdeals is seeing massive data volume growth. They recognized opportunities to drive the business using this data, but they needed to remove the barriers described here to make that happen. Flexible and elastic storage is a must.
How Slickdeals made self-service BI a reality
Given the seven barriers above—which, again, both legacy businesses and growing startups often face in various forms—Greg and his team had their work cut out for them. Here’s how they tackled these challenges.
The team decided it was time to make the leap into the cloud. First, they adopted Snowflake for a proof of concept (POC) and started pushing data there. This almost immediately solved their JSON parsing problem, taking it from a days-long process to something they could be done in minutes.
Next, to facilitate visualizations, they connected their data in Snowflake to Tableau for email analytics. Emails are a primary way that users find out about deals, and ensuring they receive relevant emails in a timely manner is very important. The data team built out an excellent BI dashboard for internal usage, but it wasn’t self-service yet. Slickdeals teams like product management, engineering, and content needed to be able to access data but they’d have to use SQL queries inside Tableau to do this—a very tall order for the average business user.
This was the key pivot point when the team realized they needed a “common semantic layer and single source of truth that can serve multiple BI tools,” in Greg’s words. Enter AtScale.
AtScale helps accelerate and simplify BI. With AtScale on board between their data and BI tools, Slickdeals realized faster time-to-insight, empowering them to make better business decisions, and delivering even more ROI on their cloud investment. For SlickDeals, this enabled them to finally make self-service BI a reality for all users.
The proof is in the pudding
At Slickdeals, it doesn’t matter if you are an engineer, a data scientist, a marketer, or a product manager. If you need data to power your business decisions, you can access it—quickly. With its new cloud-optimized data infrastructure, Slickdeals has managed to take a highly complex and voluminous data problem and translate it into data-driven decisions at scale.
To learn more on this topic, join us for our upcoming webinar, “How to Make Smarter Data-Driven Decisions at Scale,” on 3/31 where data-driven leaders will share how they are scaling smarter decision-making across their organizations and making data more accessible as a strategic asset.
Power BI/Fabric Benchmarks