February 1, 2024
From Data Products to Analytics Products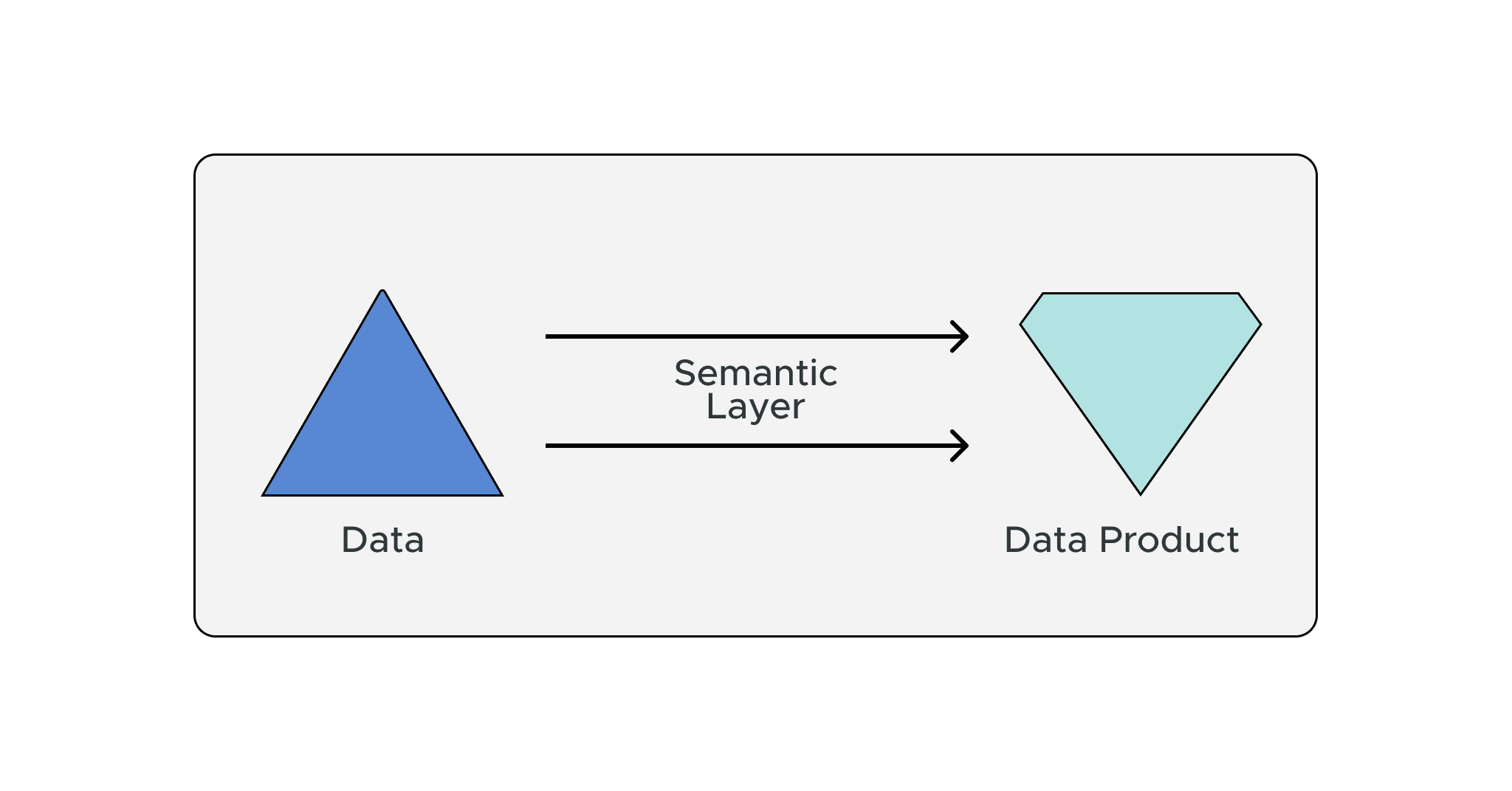
This blog is part of a series from Vin Vashishta, Founder and Technical Advisor with V Squared. V Squared advises businesses on AI strategy, data product strategy, transformation, and data organizational build-out services. They have helped clients deliver products with $100M+ in ARR and built small (5 data professionals) to large (30 professionals) D&A organizations. V Squared clients include Airbus, Siemens, Walmart, JPMC, as well as a wide range of smaller to medium businesses. Follow Vin on LinkedIn, Twitter, YouTube, and his website.
—
Data is not the new oil. According to the World Economic Forum, it is an entirely new asset class. If I buy a barrel of oil, it’s gone as soon as I use it, and I must buy another. Meanwhile, data can be used repeatedly without being depleted. Oil supports a single type of product, and even as broadly applicable as petroleum-based products can be, data supports an unlimited number of potential use cases.
The better the dataset, the more value trapped inside. Data products monetize datasets and deliver that value to customers, the business, and internal users. There are three main monetization categories:
- Business productivity improvements
- Cost savings and efficiencies
- New revenue streams
One example of a productivity-improving data product is intelligent automation.
- Ex: Amazon automates pricing decisions on its e-commerce platform to handle its massive product catalog.
Supply chain optimization or de-risking is an example of an efficiency data product.
- Ex: Apple manages its inventory and supply chains with advanced models.
Customer insight platforms or model-supported features are categories of customer-facing data products.
- Ex: Microsoft’s Copilot serves coding recommendations to software engineers for a monthly fee. Fitness apps track and serve diet and exercise insights to users.
Most Data Products Should Not Be Built By Data Teams
Amazon, Microsoft, and Apple bring to mind technically complex models built by high-end data teams. However, most data products are not created by data teams. That may surprise many, but relying on data teams for all the business’s data products makes the data team a productivity bottleneck.
Why is that? Because businesses don’t have enough access to data talent. Costs quickly escalate when the business attempts to raise staffing levels to handle every initiative. It’s not sustainable. Many use cases cannot be justified due to the cost of data talent and time, so they go unserved.
Plus, data teams don’t have the domain expertise. Users understand their needs best and build solutions that are aligned with business value. Data teams take longer to deliver solutions because of time-consuming tasks like requirements gathering and user acceptance testing add significant project overhead.
Innovation initiatives generate the highest value. Taking data scientists off these projects to work on simpler data products slows the pace of innovation. It also reduces the data team’s ROI.
How Should Data Products Be Built?
Companies like Google and Meta are seen as data titans. Both have been challenged to turn innovative data products into business value. Like most other businesses, they have a high rate of initial failure. Technology and top-tier talent can’t solve the ROI equation.
Meta recently reorganized their data science team in an attempt to improve the amount of value those teams delivered. Their data science team began as a Center of Excellence (CoE). In the CoE model, businesses put all their talent and infrastructure in the hands of a centralized team.
The CoE model has multiple benefits. The biggest is centralizing data and supporting infrastructure. Consolidation makes data teams more efficient. Then, innovation thrives (as it did at Meta), but maybe a bit too much.
The Critical Foundation of Domain Expertise
Domain expertise is critical for high-value data products. When the data team is a CoE, there is a greater distance between users and solutions. Data teams don’t have as much interaction with their internal users or customers. Knowledge doesn’t flow into the group from other business units. Rotating different data teams or personnel to support the same business unit compounds the problem.
Most people are surprised to learn how much domain expertise plays into successful data products. Data scientists make several business decisions while implementing a solution. What data is necessary? How should the data product function? What unstated requirements help integrate the data product into user workflows?
Requirements gathering and knowledge transfers add overhead to data product development projects. Meta broke up its CoE model. Now their data teams are embedded into the business units and product teams they support. The closer solutions developers are to the business and customer needs, the better data products align with value.
Self-Service Data Product Development
Domain experts are the best solutions developers. Expert knowledge is better than any version one model, no matter how complex the deep learning behind it is. I start every data science project with an expert systems approach. I talk with the people with the highest domain knowledge level and build a digital twin. Why?
The digital twin is the answer to the major challenges facing data product production. The first version is built on solid foundations. Most of these solutions never lead to a version two. The ROI of improving on the digital twin isn’t there. That’s the reality of digital products. High value can come from simple solutions that are quickly delivered and closely aligned with business needs.
But don’t we need data scientists and analysts to build data products? That is a widely held misconception. It comes from the assumption that all data products involve complex models and implementations. Self-service tools are just as effective, allowing users to meet their needs.
However, setting users up for success requires some work upfront.
How Your Semantic Layer Strategy Sets Self-Service Up for Success
Centralized data is a prerequisite for self-service solutions in the modern data landscape. Access to data is a significant barrier to delivering data products. Most users don’t have data engineering capabilities. When data is scattered across the business, building access to it is time-consuming. That’s because there are inconsistencies between datasets, and it’s challenging to know what’s available.Data mesh architecture is also essential to facilitate scaling data analytics and self-service across the business. In order enable a self-service architecture, data should be centralized. Meanwhile, access and tools should be decentralized. Each organization should have the flexibility to build data products with enough customization to meet granular needs. Without the data mesh architecture, enterprises are left with one size fits all deployments, which reduce the effectiveness of self-service tools. That architecture is a critical part of keeping solutions development close to user needs.
How Governance Comes Into Play
Standard data governance and quality practices are other prerequisites. Low-quality data sabotage data products. Remember this key phrase: Garbage in, garbage out. If users cannot rely on the data, there’s no point in using it to build data products. Security, regulatory compliance, and privacy must also be managed and standardized.
Training and Continuous Learning
Data literacy training is essential to enhancing data accessibility and time-to-insights. Front-line workers can succeed as data product developers and don’t need full-blown data science capabilities. However, there are still pitfalls to avoid and best practices to implement.
It’s critical that organizations learn how to define data product reliability requirements and develop solutions to meet theml. Data can be overextended so learning about limitations is important. That’s because data can give people the illusion of certainty. Data literacy training prevents those illusions from both happening and exacerbating.
Data literacy training should also focus on the tools. When selecting self-service tools, the learning curve should be a consideration. How long does it take to train basic and super users? Tools should make workflows simpler, not more complex. Front-line users are not primarily data product developers. Selecting a tool that is intuitive, focused on usability, and full-featured (like a semantic layer) is a critical success factor here.
How Should Users Be Supported?
Self-service requires company-wide support. It is vital to have a data analyst or data scientist available for each business unit. While they won’t be involved in solutions development, they will play a critical role in developing high-quality data products.
That role begins with a use case review. Some data products require higher reliability methods to implement. Having a data professional review each one will catch the small number that do need more intensive attention so users don’t overextend their data and self-service tools.
Then, the data professional should review the approach and solution. The review and approval process helps extend data literacy training. When the methods and solution are not sound, or there is room for improvement, it’s a teachable moment. Reviews prevent a faulty solution from being deployed, and the data professional can use them to teach the user how to improve.
Developing data products with self-service solutions is a partnership between the data team and front-line workers. Decentralizing solutions development speeds up time to insights and frees the data team to take on high-value, complex initiatives. Centralized data, data mesh architecture, intuitive self-service tools, and data literacy are critical strategies to building data products that make your organization soar above the rest.
ANALYST REPORT